One-step incremental multi-view spectral clustering based on graph linkage learning
Neurocomputing(2024)
摘要
Most traditional multi-view spectral clustering algorithms involve two separate steps: solving the spectral embedding matrix and clustering, which may introduce errors during the clustering process. Moreover, in practical applications, the number of available views may increase over time, and the approach of re-fusing all views in each computation would result in elevated computational costs. In this paper, we propose a novel model: One-step Incremental Multi-view Spectral Clustering based on Graph Linkage learning (OIMvSC). OIMvSC only needs to store the consensus spectral embedding matrix and the consensus label matrix of all previous views and combines them with the spectral embedding matrix of the newly available view to solve the fused consensus spectral embedding matrix and consensus label matrix. To further enhance clustering performance, OIMvSC introduces graph linkage learning, which reduces erroneous connections between clusters while preserving correct connections within clusters. A convergent iterative algorithm for solving OIMvSC is proposed. Experimental results demonstrate that OIMvSC exhibits excellent clustering performance.
更多查看译文
关键词
Multiview clustering,Spectral embedding,Graph linkage learning,One-step spectral clustering
AI 理解论文
溯源树
样例
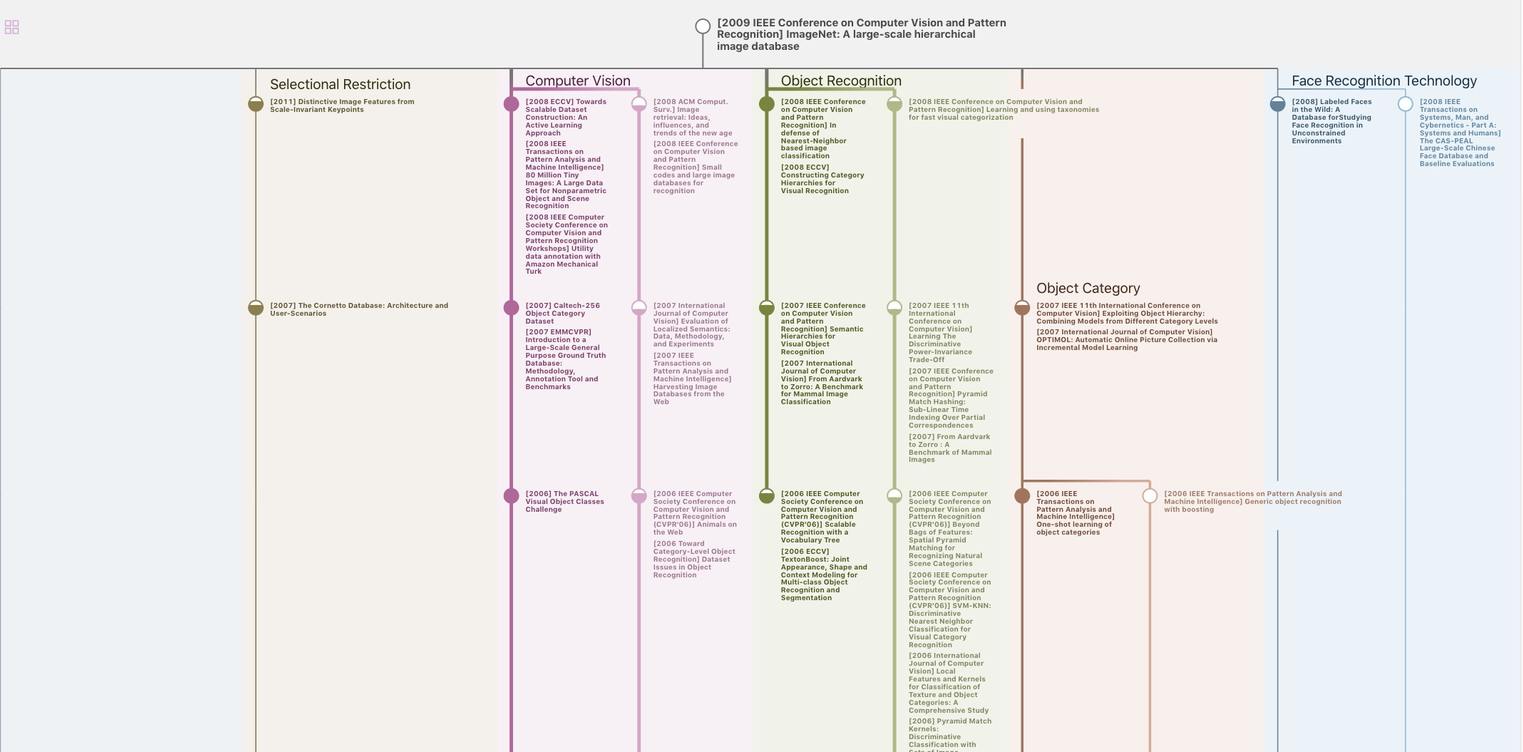
生成溯源树,研究论文发展脉络
Chat Paper
正在生成论文摘要