The mosaic permutation test: an exact and nonparametric goodness-of-fit test for factor models
arxiv(2024)
摘要
Financial firms often rely on factor models to explain correlations among asset returns. These models are important for managing risk, for example by modeling the probability that many assets will simultaneously lose value. Yet after major events, e.g., COVID-19, analysts may reassess whether existing models continue to fit well: specifically, after accounting for the factor exposures, are the residuals of the asset returns independent? With this motivation, we introduce the mosaic permutation test, a nonparametric goodness-of-fit test for preexisting factor models. Our method allows analysts to use nearly any machine learning technique to detect model violations while provably controlling the false positive rate, i.e., the probability of rejecting a well-fitting model. Notably, this result does not rely on asymptotic approximations and makes no parametric assumptions. This property helps prevent analysts from unnecessarily rebuilding accurate models, which can waste resources and increase risk. We illustrate our methodology by applying it to the Blackrock Fundamental Equity Risk (BFRE) model. Using the mosaic permutation test, we find that the BFRE model generally explains the most significant correlations among assets. However, we find evidence of unexplained correlations among certain real estate stocks, and we show that adding new factors improves model fit. We implement our methods in the python package mosaicperm.
更多查看译文
AI 理解论文
溯源树
样例
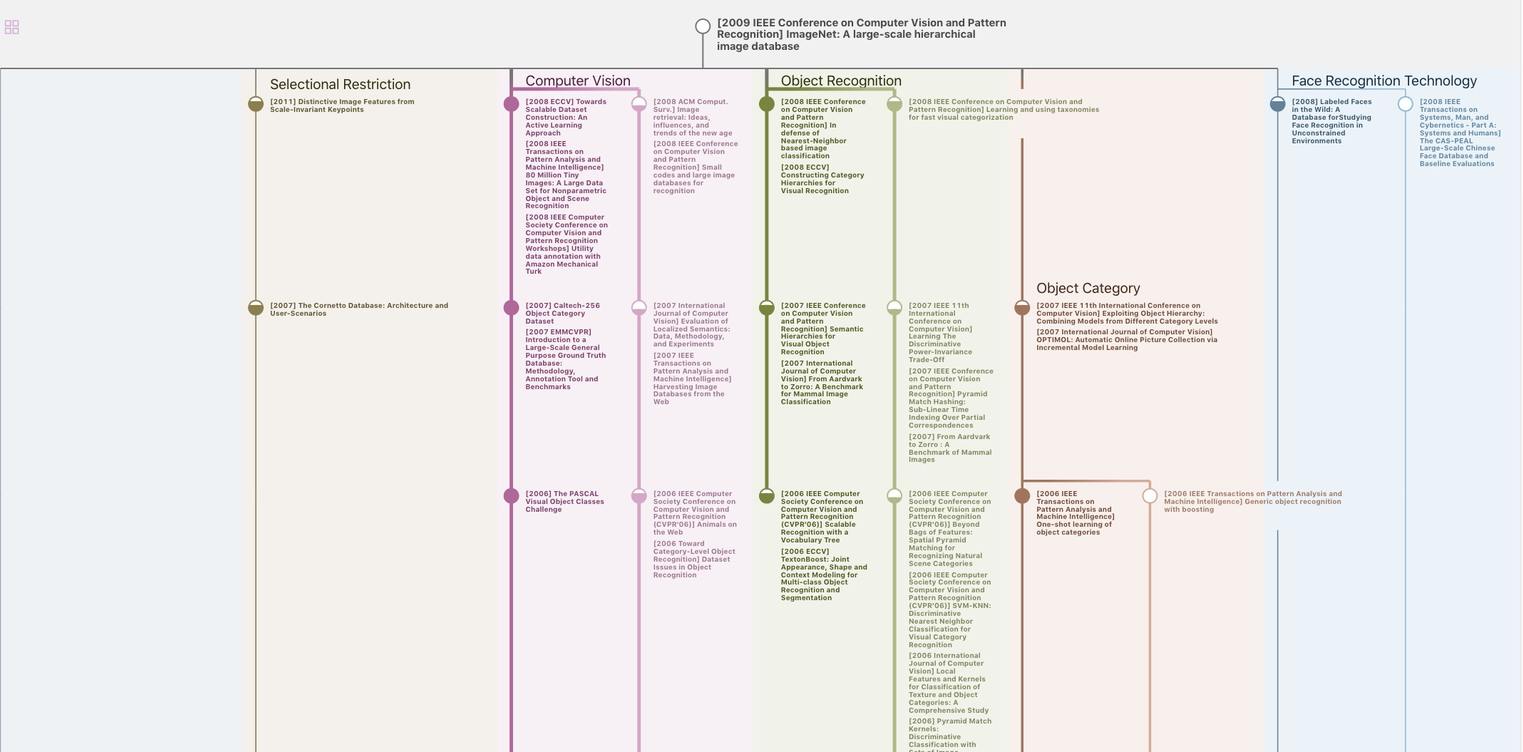
生成溯源树,研究论文发展脉络
Chat Paper
正在生成论文摘要