Other tokens matter: Exploring global and local features of Vision Transformers for Object Re-Identification
Computer Vision and Image Understanding(2024)
摘要
Object Re-Identification (Re-ID) aims to identify and retrieve specific objects from images captured at different places and times. Recently, object Re-ID has achieved great success with the advances of Vision Transformers (ViT). However, the effects of the global-local relation have not been fully explored in Transformers for object Re-ID. In this work, we first explore the influence of global and local features of ViT and then further propose a novel Global-Local Transformer (GLTrans) for high-performance object Re-ID. We find that the features from last few layers of ViT already have a strong representational ability, and the global and local information can mutually enhance each other. Based on this fact, we propose a Global Aggregation Encoder (GAE) to utilize the class tokens of the last few Transformer layers and learn comprehensive global features effectively. Meanwhile, we propose the Local Multi-layer Fusion (LMF) which leverages both the global cues from GAE and multi-layer patch tokens to explore the discriminative local representations. Extensive experiments demonstrate that our proposed method achieves superior performance on four object Re-ID benchmarks.
更多查看译文
关键词
Object Re-Identification,Vision Transformer,Global-local feature,Multi-layer fusion,Local-aware representation
AI 理解论文
溯源树
样例
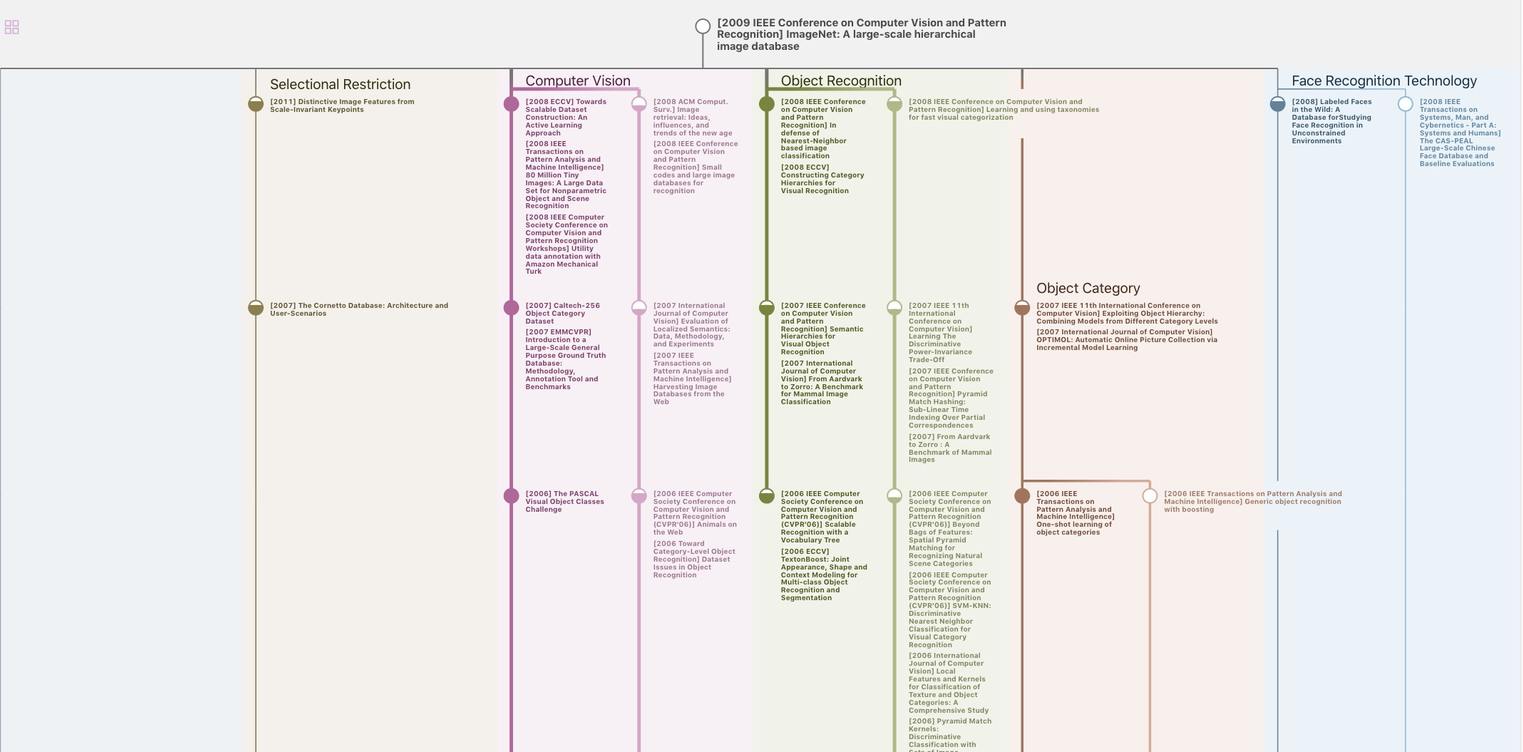
生成溯源树,研究论文发展脉络
Chat Paper
正在生成论文摘要