New Douglas-Rashford Splitting Algorithms for Generalized DC Programming with Applications in Machine Learning
arxiv(2024)
摘要
In this work, we propose some new Douglas-Rashford splitting algorithms for solving a class of generalized DC (difference of convex functions) in real Hilbert spaces. The proposed methods leverage the proximal properties of the nonsmooth component and a fasten control parameter which improves the convergence rate of the algorithms. We prove the convergence of these methods to the critical points of nonconvex optimization under reasonable conditions. We evaluate the performance and effectiveness of our methods through experimentation with three practical examples in machine learning. Our findings demonstrated that our methods offer efficiency in problem-solving and outperform state-of-the-art techniques like the DCA (DC Algorithm) and ADMM.
更多查看译文
AI 理解论文
溯源树
样例
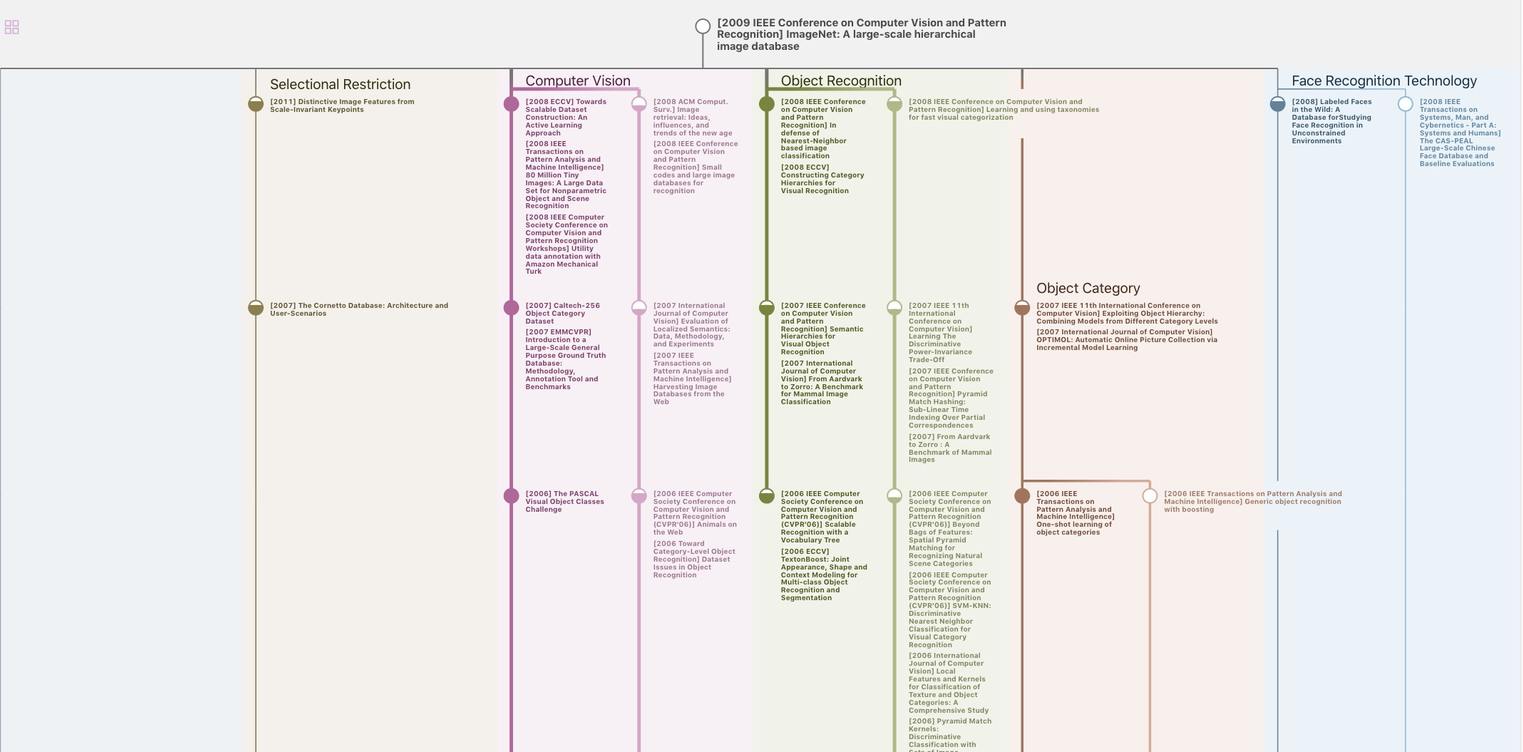
生成溯源树,研究论文发展脉络
Chat Paper
正在生成论文摘要