Real-Time Semantic Segmentation of Aerial Images Based on Dual-Feature Attention Networks
2024 4th International Conference on Neural Networks, Information and Communication (NNICE)(2024)
摘要
With the widespread use of unmanned aerial vehicles (UAVs), the segmentation of their aerial images has been widely applied. However, fast semantic segmentation of high-resolution UAV aerial images still faces several challenges: limited hardware processing resources and high real-time requirements of UAV platforms, the balance between model accuracy and real-time efficiency, and the poor inference ability of models for small target classes in aerial images. To address these issues, this paper proposes a lightweight remote sensing image bilateral segmentation network (RS-BiseNet) that achieves good inference accuracy and real-time performance for aerial images. Specifically, inspired by the concept of receptive fields, a deep separable fusion pyramid pooling module (DSFPPM) is proposed to rapidly extract multi-scale contextual information from different receptive fields. Furthermore, a dual feature attention module (DFAM) and a feature fusion module (FFM) are proposed based on the channel attention mechanism to extract fine-grained details. RS-BiseNet was evaluated on the ISPRS Potsdam dataset and obtained an improvement of 2.96% MIoU and 1.59% OA over Aerial-BiSeNet.
更多查看译文
关键词
Drone aerial images,Deep learning,Real-time semantic segmentation,Two-branch network
AI 理解论文
溯源树
样例
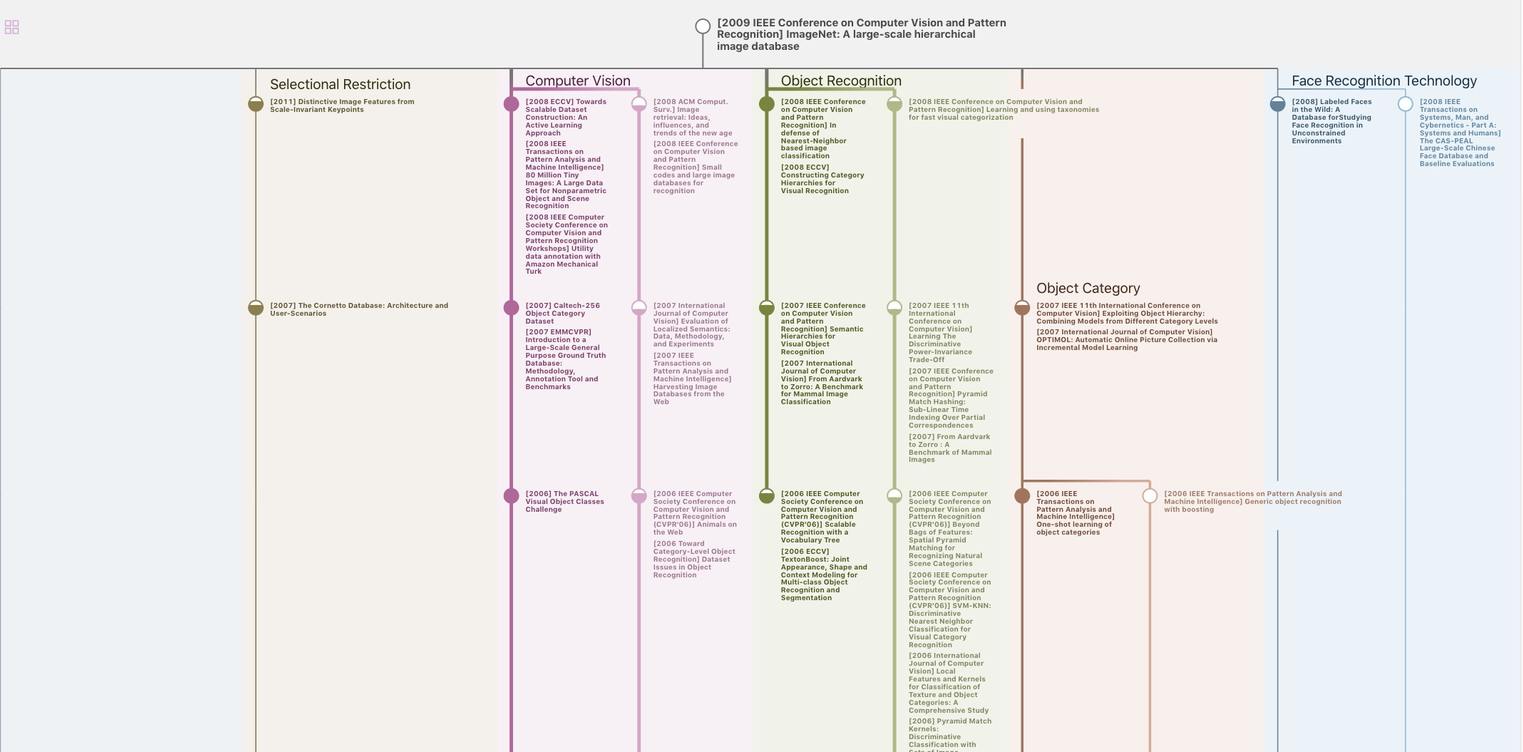
生成溯源树,研究论文发展脉络
Chat Paper
正在生成论文摘要