Dense residual network for image edge detection
Multimedia Tools and Applications(2024)
摘要
The major challenge of edge detection in denoised images is smoothing the edges, thus confusing algorithms to classify a true edge with false one. Existing learning and optimization methods tends to handle the above problem with selectivity approach to avoid the smoothed edges. In this paper, an efficient model to detect an edges of fuzzy noise images is introduced. Inspired by U-Net and dense network, a feature map reuse and residual learning with Dense Residual Network structure (DRNet) is proposed. The feature maps from earlier blocks are dynamically combined into succeeding layers as input for the proposed DRNet. This process not only aids in spatial reconstruction, but also enhances learning efficiency by utilizing more consistent gradients to allocate the most appropriate true edge. Furthermore, the noised pixel will be eliminated with the training process for the targeted edges. To overcome the overfitting challenge in the network, this paper proposes an implementation of the DropBlock technique. The model is trained and tested using a renowned edge detection public datasets. The experimental results demonstrate that the proposed model outperformed other conventional and learning detection algorithms in terms of peak signal-to-noise ratio in different levels. These results confirm the efficacy of this study and offer a fresh approach to enhancing edge detection.
更多查看译文
关键词
Edge detection,U-Net,DRNet,DropBlock,DRB
AI 理解论文
溯源树
样例
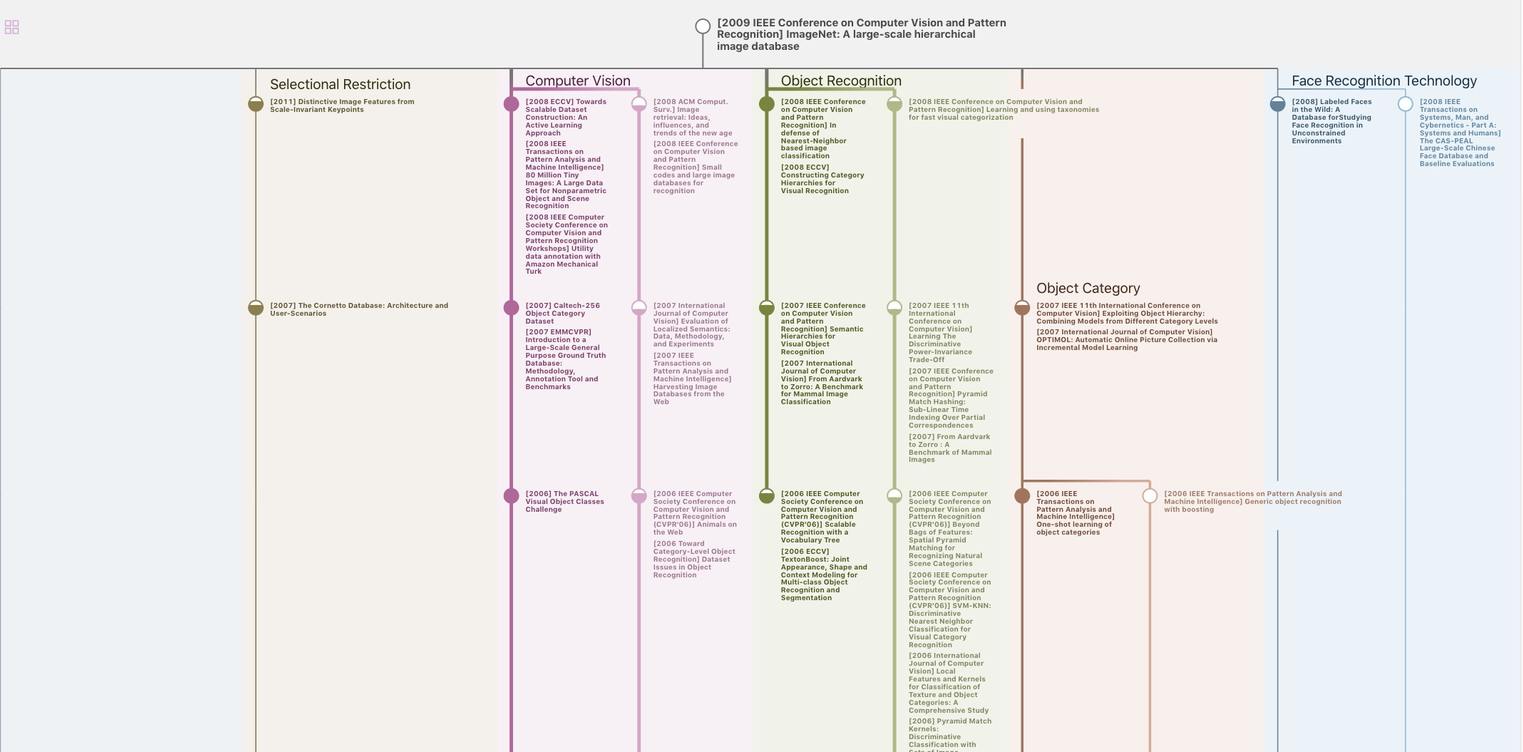
生成溯源树,研究论文发展脉络
Chat Paper
正在生成论文摘要