A Probabilistic Structural Damage Identification Method with a Generic Non-Convex Penalty
MATHEMATICS(2024)
摘要
Due to the advantage that the non-convex penalty accurately characterizes the sparsity of structural damage, various models based on non-convex penalties have been effectively utilized to the field of structural damage identification. However, these models generally ignore the influence of the uncertainty on the damage identification, which inevitably reduces the accuracy of damage identification. To improve the damage identification accuracy, a probabilistic structural damage identification method with a generic non-convex penalty is proposed, where the uncertainty corresponding to each mode is quantified using the separate Gaussian distribution. The proposed model is estimated via the iteratively reweighted least squares optimization algorithm according to the maximum likelihood principle. The numerical and experimental results illustrate that the proposed method improves the damage identification accuracy by 3.98% and 7.25% compared to the original model, respectively.
更多查看译文
关键词
damage identification,model updating,non-convex function penalty,uncertainty,sparsity
AI 理解论文
溯源树
样例
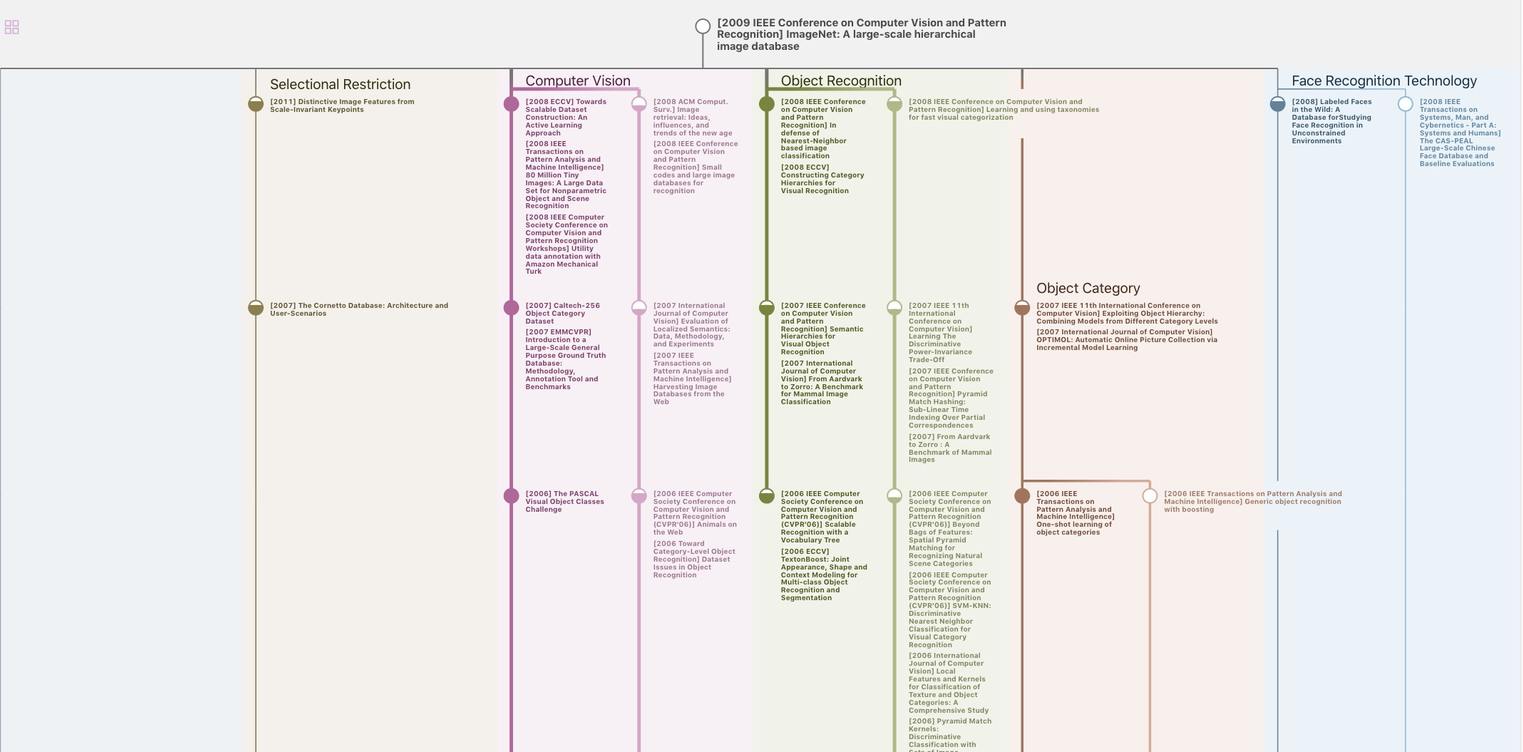
生成溯源树,研究论文发展脉络
Chat Paper
正在生成论文摘要