Optimizing Inference Capabilities in Chinese NLP: A Study on Lightweight Generative Language Models for Knowledge Question Answering
2024 4th International Conference on Neural Networks, Information and Communication (NNICE)(2024)
Abstract
Currently, most Chinese language models, such as ChatGLM-6b and Baichuan-7b, predominantly employ the structure of GPT Decoder. These models, while powerful, are characterized by an enormous number of parameters, which may not be necessary for tasks emphasizing reasoning capabilities over extensive knowledge base. To address this, we propose the LGLM model, a leaner model with a parameter size of 1.3 billion, specifically designed for Chinese text comprehension. Despite its smaller size, the LGLM model retains robust inference capabilities akin to those of ChatGLM-6b and Baichuan-7b models, and significantly reduces resource consumption. In our experiments conducted on the c3 datasets, the LGLM model demonstrated comparable performance to larger models. To further enhance the model's performance and address the issue of language model hallucination, we introduced QA-head, a modification to the original llm-head. This addition not only improved the performance of our model but also showed promising results when applied to other models. This approach, therefore, presents a more efficient and effective solution for Chinese text comprehension tasks.
MoreTranslated text
AI Read Science
Must-Reading Tree
Example
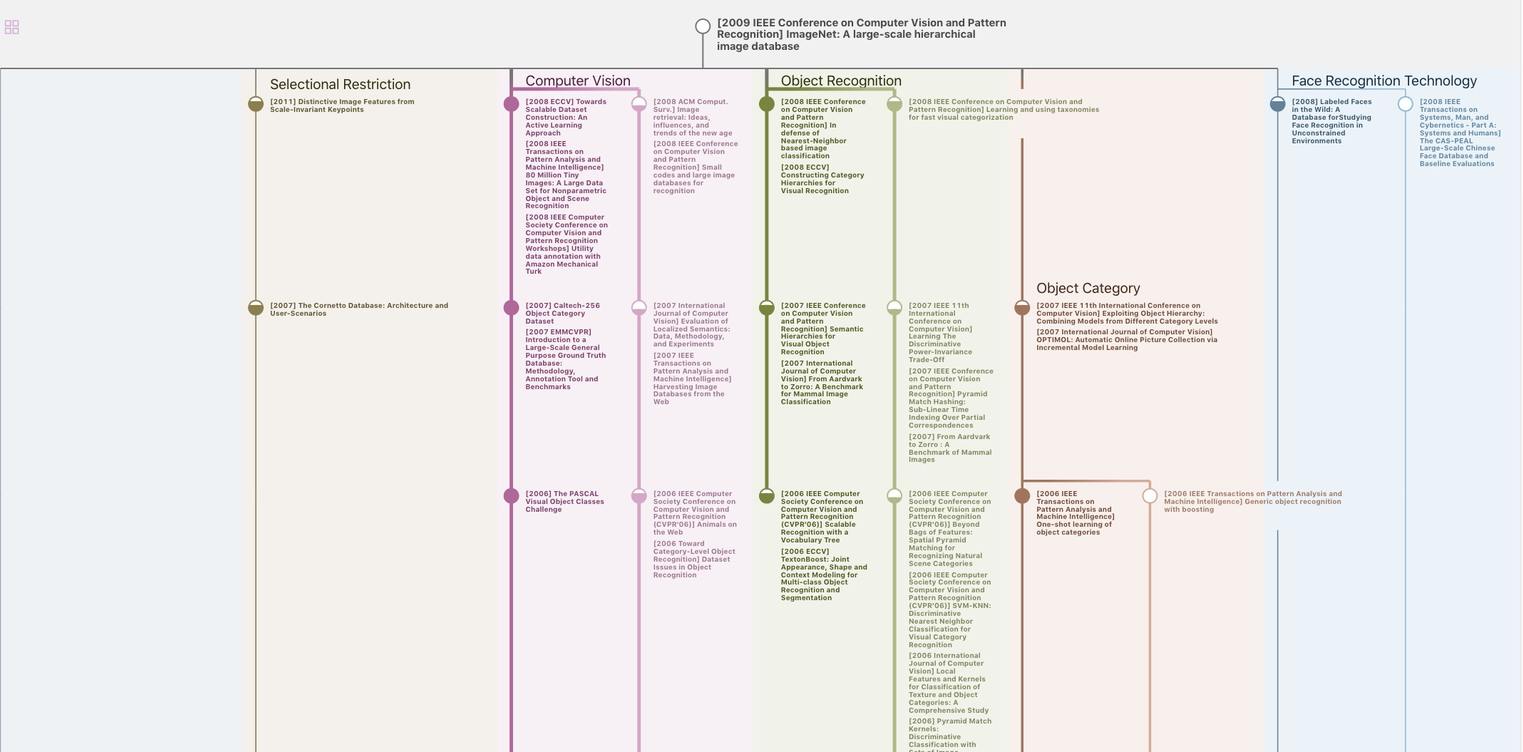
Generate MRT to find the research sequence of this paper
Chat Paper
Summary is being generated by the instructions you defined