Few-Shot Lung Cancer Classification Using Prototypical Networks
2024 4th International Conference on Advanced Research in Computing (ICARC)(2024)
Abstract
Lung cancer, a leading cause of mortality globally, demands early and accurate detection to improve patient out-comes. Current diagnostic methods, primarily relying on CT scans, face challenges in lung cancer subtype identification, particularly due to the scarcity of extensive medical image datasets for each subtype. This study addresses this limitation by leveraging prototypical networks, an innovative few-shot learning approach, which excels in scenarios with limited data. The proposed method capitalizes on a small number of samples per category, integrating a pre-trained model for feature extraction from lung CT scans. We rigorously evaluated the model's performance, focusing on its accuracy relative to the sample size per category. Remarkably, the method achieved a 98% accuracy rate after 15 epochs, showcasing its efficacy. This research not only confirms the feasibility of using prototypical networks for lung cancer subtype classification but also opens new avenues for applying few-shot learning techniques in medical imaging. Our findings hold significant potential for enhancing lung cancer diagnostics, thereby contributing to improved patient care and survival rates.
MoreTranslated text
Key words
CT Image,U-net Architecture,VGG16,ResNet50,DenseNet
AI Read Science
Must-Reading Tree
Example
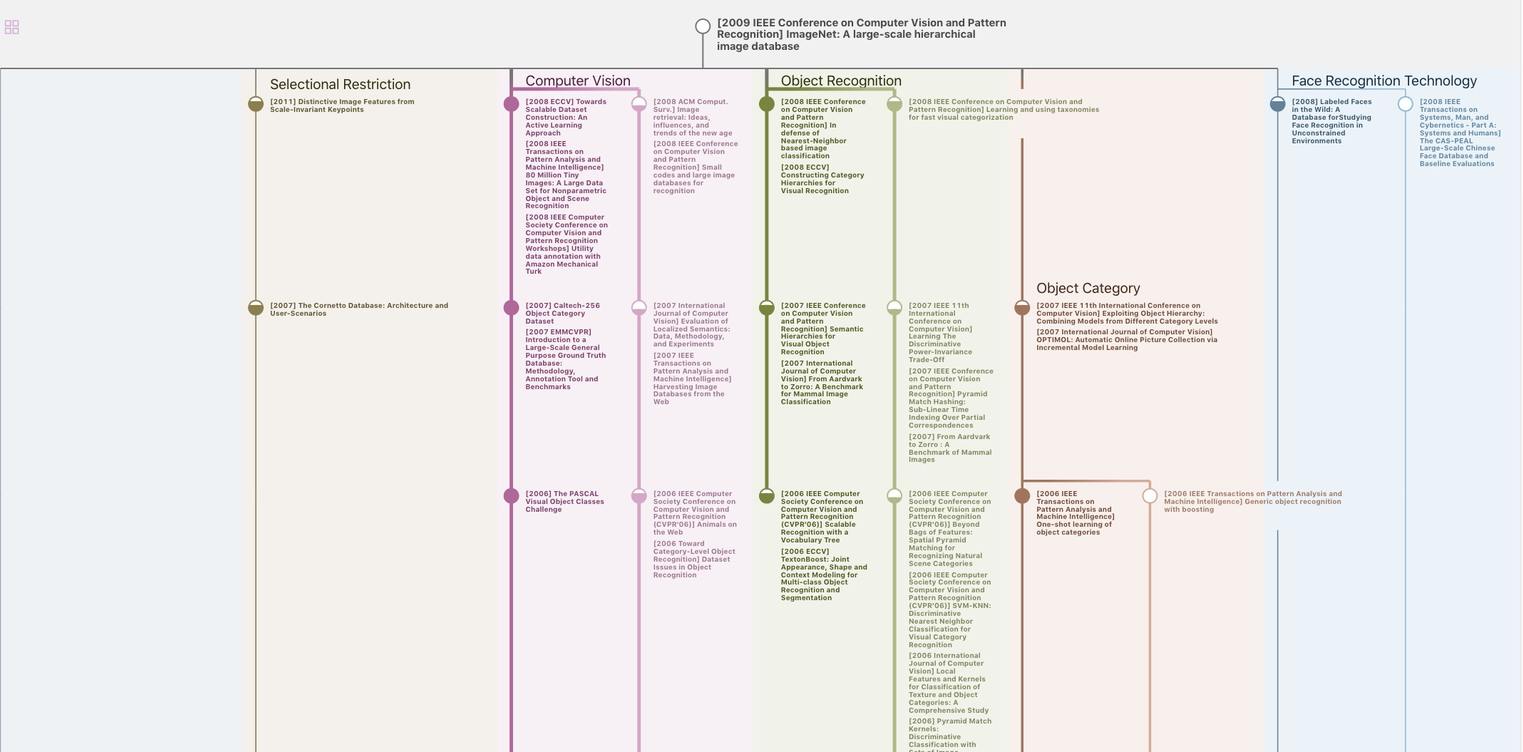
Generate MRT to find the research sequence of this paper
Chat Paper
Summary is being generated by the instructions you defined