Dual-branch deep learning architecture enabling miner behavior recognition
Multimedia Tools and Applications(2024)
摘要
Nonstandard miner behavior can have adverse effects on coal mine safety production. Therefore, accurately capturing miner behavior in complex environments is particularly important. In the intelligent mine monitoring system, using visual perception to detect miner behavior is a challenging task due to high behavioral similarity and difficult temporal relationships. In this paper, a new deep learning framework is proposed to construct a coal miner behavior recognition model with a spatio-temporal dual-branch structure and transposed attention representation mechanism. The spatio-temporal dual-branch structure extracts rich spatial semantic information from intrinsic safety video sensor input video sequences while ensuring effective capture of rapidly changing human behavior. Subsequently, considering the discrimination of miner behavior similarity, a merged transposed weighted representation mechanism (TWR) is introduced to guide the model in extracting feature information more strongly related to the classification target, thereby effectively improving the model’s ability to classify highly similar behaviors. Experiments were conducted on UCF101, HMDB51, and a self-built miner behavior dataset, achieving significant improvements compared to other state-of-the-art methods. This collaborative structure further creates a more discriminative behavior detection model, contributing to the reliability of miner behavior detection.
更多查看译文
关键词
Unsafe behavior,Spatiotemporal dual-branch,Visual sensing,Transposed weighted representation,Discrimination
AI 理解论文
溯源树
样例
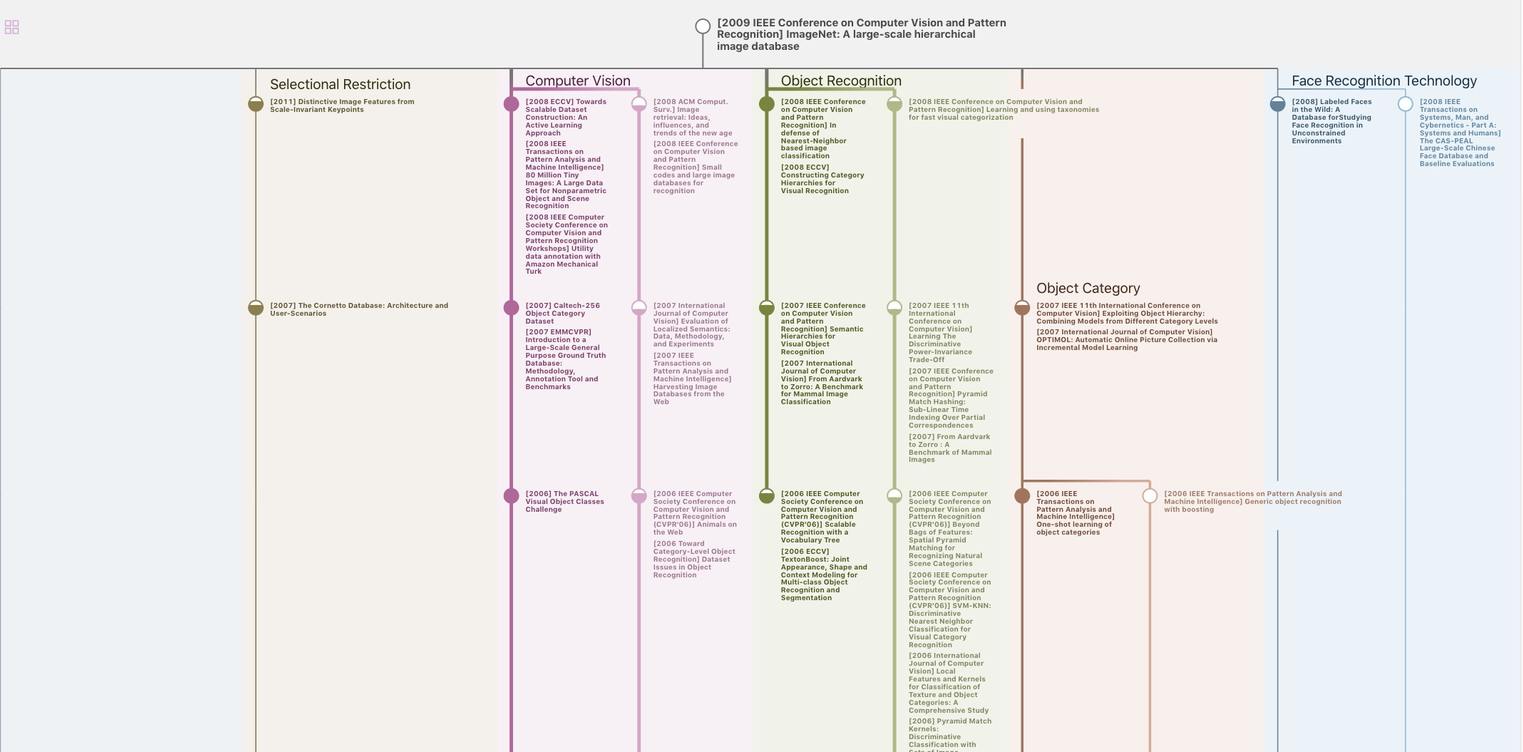
生成溯源树,研究论文发展脉络
Chat Paper
正在生成论文摘要