A novel process monitoring framework combined temporal feedback autoencoder and multilevel correlation analysis for large-scale industrial processes
Measurement(2024)
摘要
Modeling and monitoring generally face numerous challenges such as complex characteristics of multi-unit, temporal correlations and strong interaction among subblocks in large-scale industrial processes. To handle those challenges, a novel process monitoring framework combined temporal feedback autoencoder and multilevel correlation analysis is proposed in this paper. Firstly, large-scale industrial processes are decomposed into multiple subblocks in spatial and temporal order based on process knowledge. Secondly, an improved autoencoder with temporal feedback mechanism is constructed as local monitoring model to capture the important latent variables of each subblock. Then, considering the sequential transmission and correlations among subblocks in series, a multilevel correlation analysis method is employed to efficiently extract the unique features of each subblock and the joint features of the whole process. Finally, the irregular contribution indices of the unique features and the joint features are designed for hierarchical process monitoring. The superiority of the proposed framework can be verified by Tennessee Eastman process and a real hot strip mill process.
更多查看译文
关键词
Hierarchical process monitoring,Large-scale industrial processes,Unique features and joint features,Temporal feedback autoencoder,Multiple subblocks
AI 理解论文
溯源树
样例
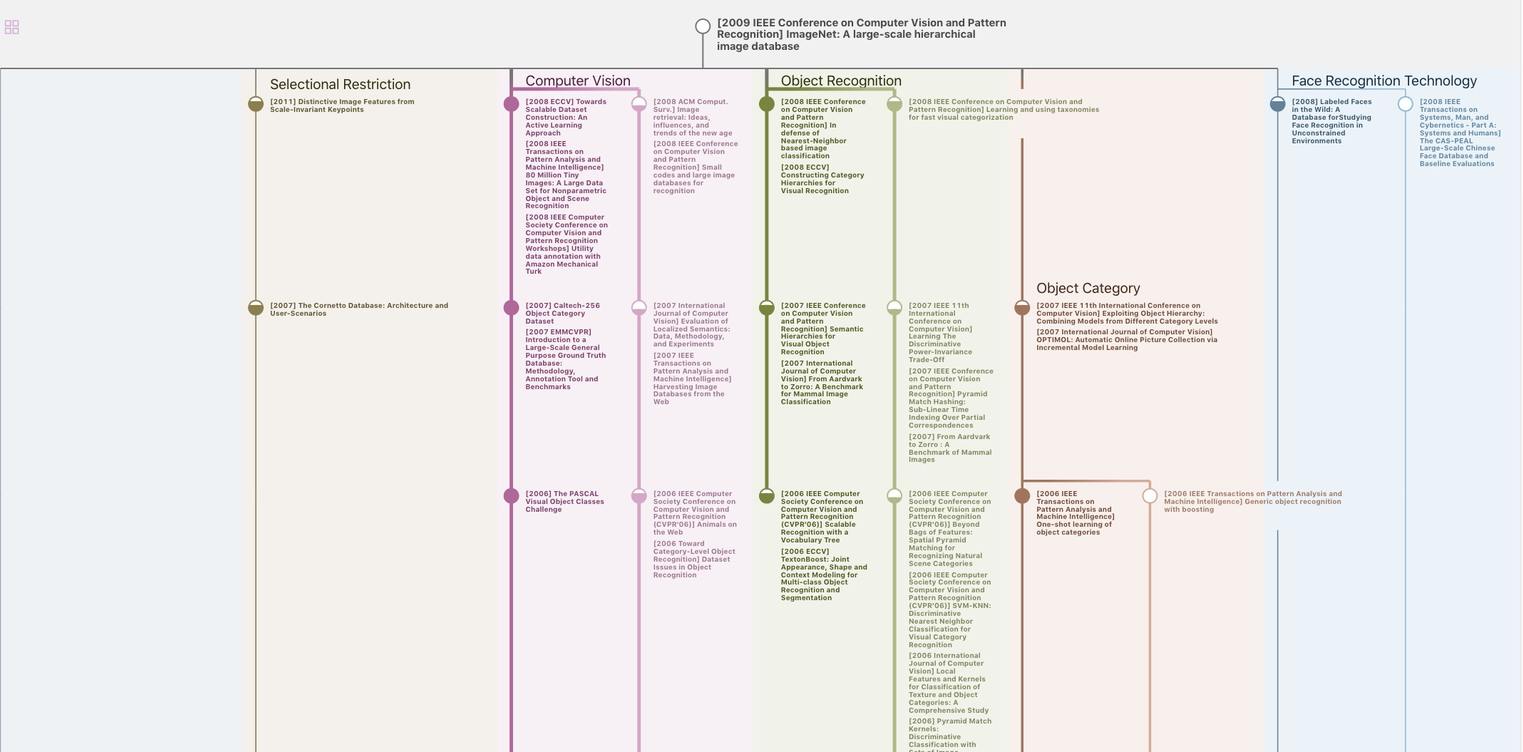
生成溯源树,研究论文发展脉络
Chat Paper
正在生成论文摘要