Prediction models for bioavailability of Cu and Zn during composting: Insights into machine learning
JOURNAL OF HAZARDOUS MATERIALS(2024)
Abstract
Bioavailability assessment of heavy metals in compost products is crucial for evaluating associated environmental risks. However, existing experimental methods are time-consuming and inefficient. The machine learning (ML) method has demonstrated excellent performance in predicting heavy metal fractions. In this study, based on the conventional physicochemical properties of 260 compost samples, including compost time, temperature, electrical conductivity (EC), pH, organic matter (OM), total phosphorus (TP), total nitrogen, and total heavy metal contents, back propagation neural network, gradient boosting regression, and random forest (RF) models were used to predict the dynamic changes in bioavailable fractions of Cu and Zn during composting. All three models could be used for effective prediction of the variation trend in bioavailable fractions of Cu and Zn; the RF model showed the best prediction performance, with the prediction level higher than that reported in related studies. Although the key factors affecting changes among fractions were different, OM, EC, and TP were important for the accurate prediction of bioavailable fractions of Cu and Zn. This study provides simple and efficient ML models for predicting bioavailable fractions of Cu and Zn during composting, and offers a rapid evaluation method for the safe application of compost products.
MoreTranslated text
Key words
Livestock and poultry waste,Heavy metal bioavailability,Back propagation neural network,Gradient boosting regression,Random forest
AI Read Science
Must-Reading Tree
Example
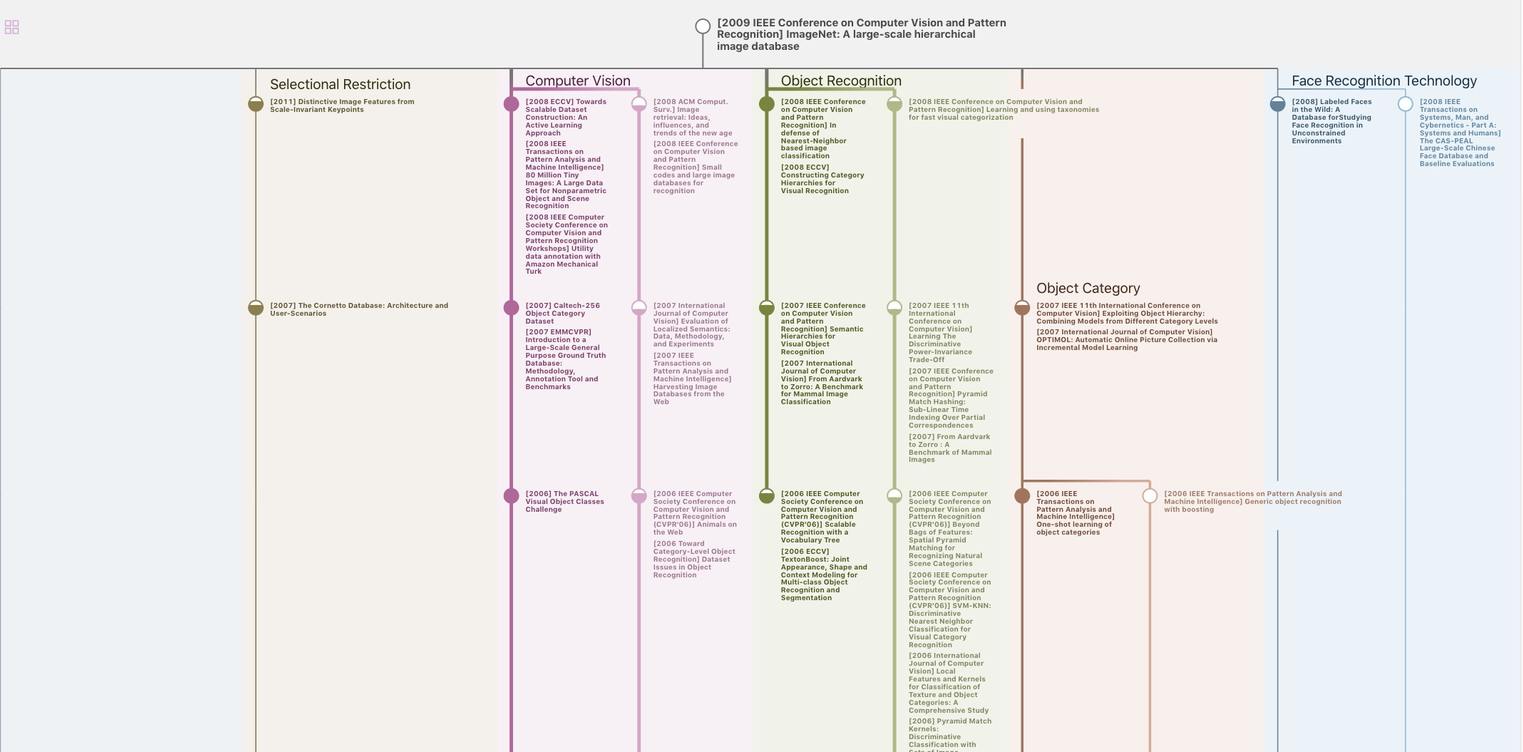
Generate MRT to find the research sequence of this paper
Chat Paper
Summary is being generated by the instructions you defined