An Efficient Residual-Based Neural Network for Super Resolution and High-precision Quantization Method Design
2024 4th International Conference on Neural Networks, Information and Communication (NNICE)(2024)
摘要
In response to the current problems of deep layers and large model parameters in CNN for super-resolution, we propose a lightweight and efficient network model (LRSPCN) based on residuals. An efficient feature extraction module is built in model to learn effective information from row images, we induce residual connection units to achieve deep and shallow feature combination. To reduce model computational complexity, sub-pixel convolution layer is inserted in to obtain image upscale reconstruction. In our model, the amount of convolution layers and model parameters are enormously decreased. Furthermore, this paper proposes an efficient quantization method, which has the advantage of higher accuracy and lower bit-width compared to commonly used quantization methods. In our design, weight, bias and feature map are quantized uniformly to the same bit-width, which greatly compressed the storage requirements of model parameters and improved the accuracy and efficiency of hardware in edge-inference reconstruction implementation.
更多查看译文
关键词
super resolution,neural network,sub-pixel convolution,residual connection,model quantization
AI 理解论文
溯源树
样例
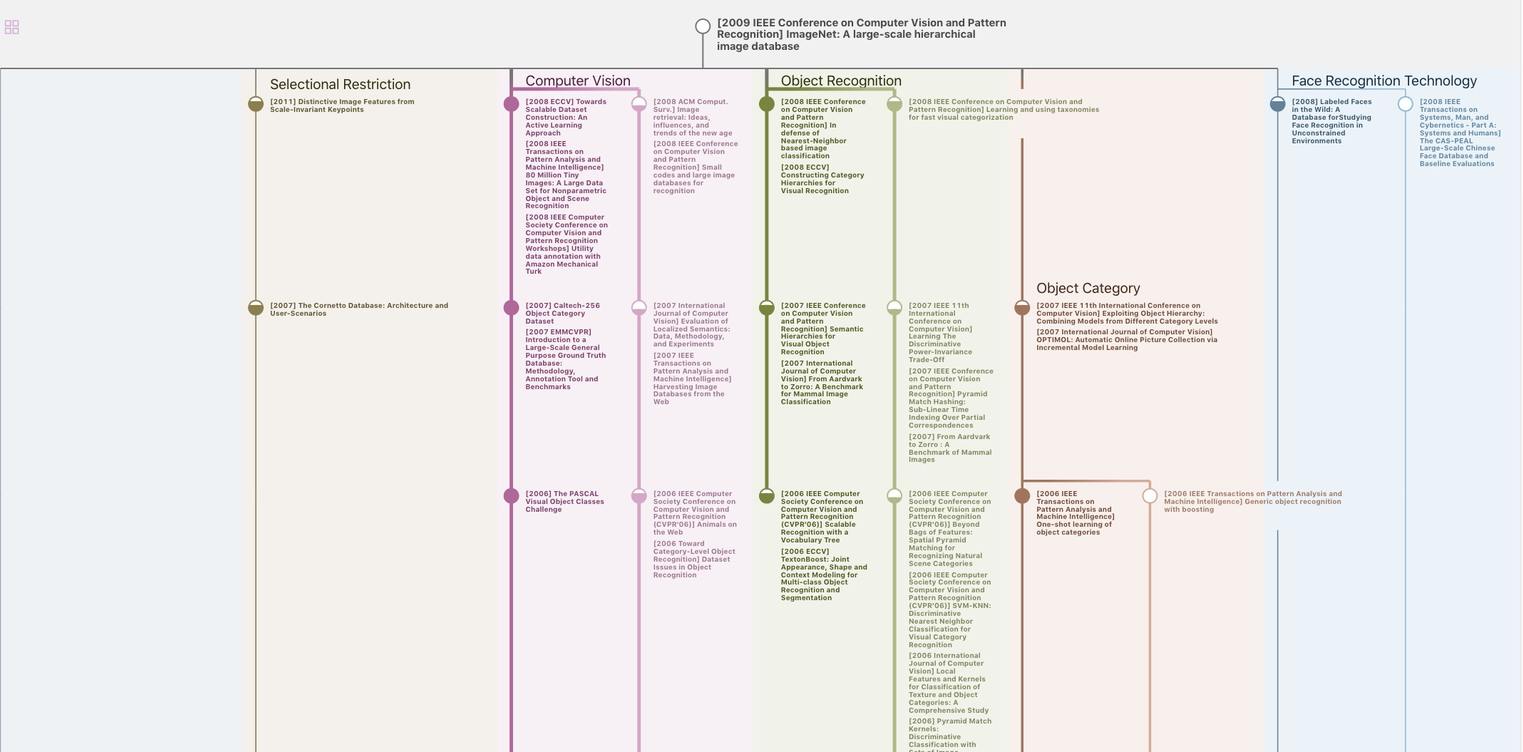
生成溯源树,研究论文发展脉络
Chat Paper
正在生成论文摘要