Dynamic Quantum Federated Learning for Satellite-Ground Integrated Systems Using Slimmable Quantum Neural Networks
IEEE ACCESS(2024)
摘要
Recent advances in low Earth orbit (LEO) satellites have made it possible to achieve zero blind spots on Earth. Considering the give locations of these devices, this makes satellite-ground links between quantum devices a practical possibility. This paper proposes the first quantum federated learning (QFL) application in satellite-ground communication. To improve communication and computing performance, this paper adopts slimmable quantum federated learning (SQFL) and slimmable quantum neural networks (sQNN), which allow for two different configurations in quantum neural networks: the angle and pole configurations. This paper also employs superposition coding and successive decoding to increase communication opportunities. Through extensive experiments, the proposed satellite-ground SQFL framework performs well and is both computationally and communicationally efficient compared to classical federated learning and QFL.
更多查看译文
关键词
Satellite-ground communication,quantum machine learning,quantum computing,federated learning
AI 理解论文
溯源树
样例
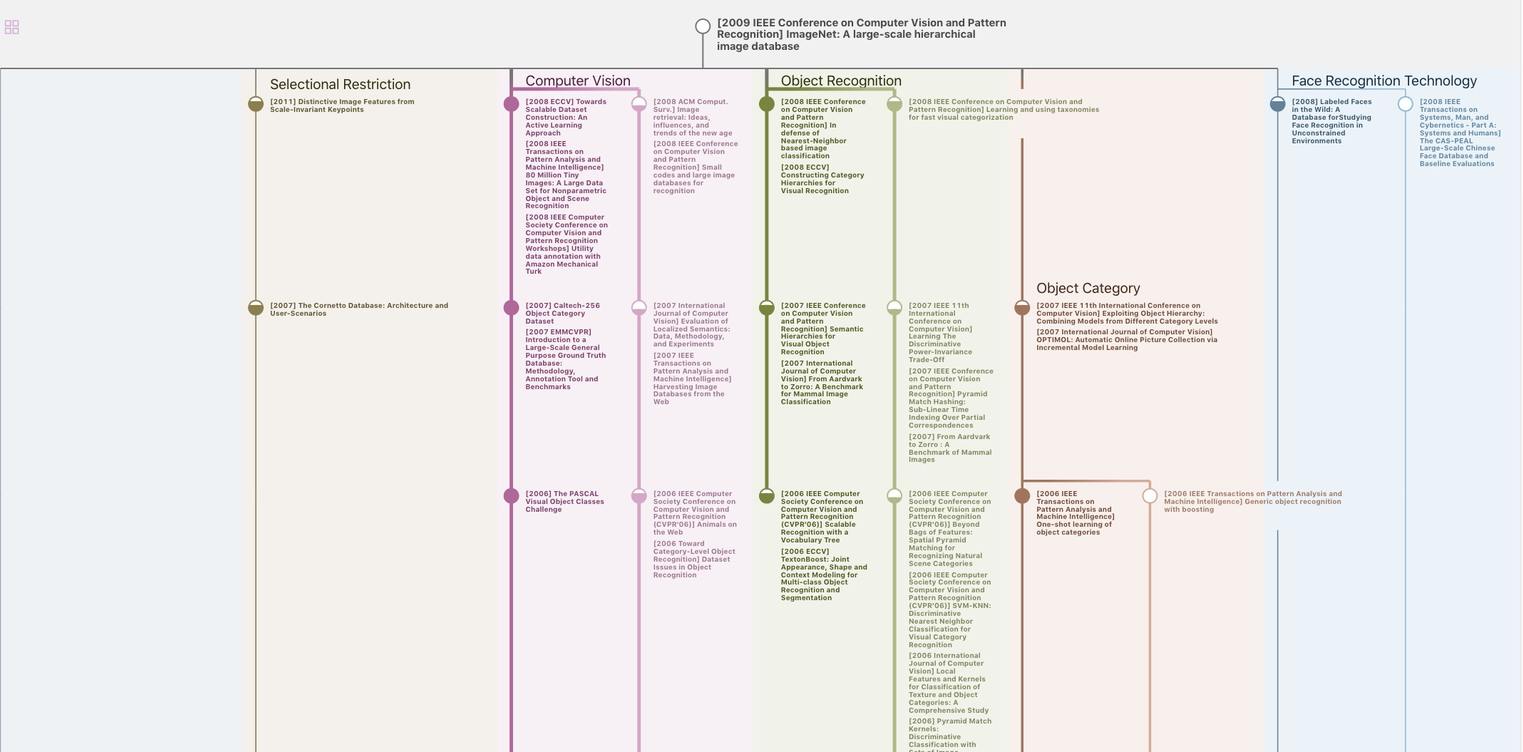
生成溯源树,研究论文发展脉络
Chat Paper
正在生成论文摘要