Rate Analysis of Coupled Distributed Stochastic Approximation for Misspecified Optimization
arxiv(2024)
摘要
We consider an n agents distributed optimization problem with imperfect
information characterized in a parametric sense, where the unknown parameter
can be solved by a distinct distributed parameter learning problem. Though each
agent only has access to its local parameter learning and computational
problem, they mean to collaboratively minimize the average of their local cost
functions. To address the special optimization problem, we propose a coupled
distributed stochastic approximation algorithm, in which every agent updates
the current beliefs of its unknown parameter and decision variable by
stochastic approximation method; and then averages the beliefs and decision
variables of its neighbors over network in consensus protocol. Our interest
lies in the convergence analysis of this algorithm. We quantitatively
characterize the factors that affect the algorithm performance, and prove that
the mean-squared error of the decision variable is bounded by
𝒪(1/nk)+𝒪(1/√(n)(1-ρ_w))1/k^1.5+𝒪(1/(1-ρ_w)^2)1/k^2, where k is the iteration count and (1-ρ_w) is the
spectral gap of the network weighted adjacency matrix. It reveals that the
network connectivity characterized by (1-ρ_w) only influences the high
order of convergence rate, while the domain rate still acts the same as the
centralized algorithm. In addition, we analyze that the transient iteration
needed for reaching its dominant rate 𝒪(1/nk) is
𝒪(n/(1-ρ_w)^2). Numerical experiments are carried out to
demonstrate the theoretical results by taking different CPUs as agents, which
is more applicable to real-world distributed scenarios.
更多查看译文
AI 理解论文
溯源树
样例
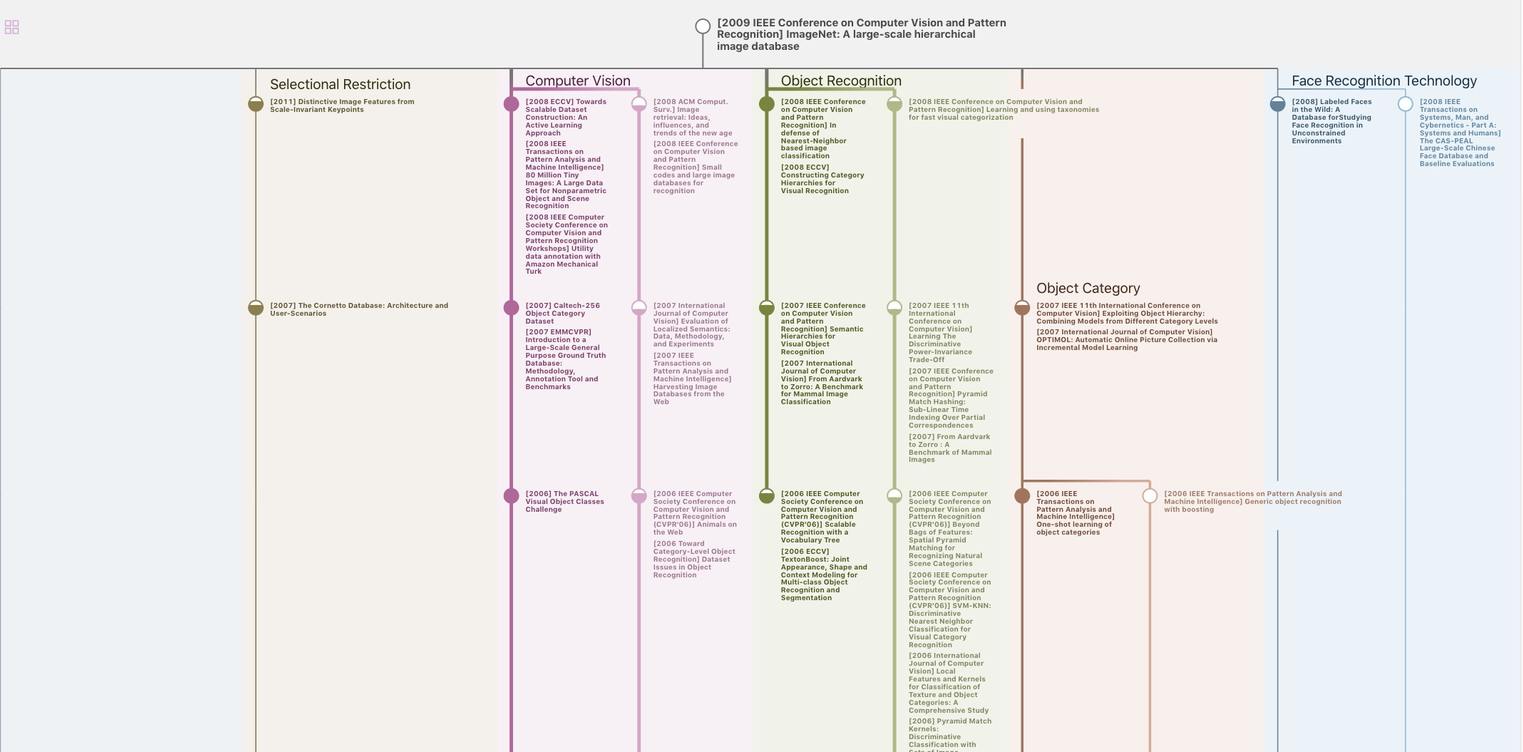
生成溯源树,研究论文发展脉络
Chat Paper
正在生成论文摘要