R-Vine Copulas for Data-Driven Quantification of Descriptor Relationships in Porous Materials
ADVANCED THEORY AND SIMULATIONS(2024)
摘要
Local variations in the 3D microstructure can control the macroscopic behavior of heterogeneous porous materials. For example, the permittivity through porous sheets or membranes is governed by local high-volume pathways or bottlenecks. Due to local variations, unfeasibly large amounts of microstructure data may be needed to reliably predict such material properties directly from image data. Here it is demonstrated that a vine copula approach provides parametric models for local microstructure descriptors that compactly capture the 3D microstructure including its local variations and efficiently probe it with respect to selected, measurable properties. In contrast to common methods of complexity reduction, the proposed approach creates parametric models for the multivariate probability distribution of high-dimensional descriptor vectors that inherently contain the complex, nonlinear dependencies between these descriptors. Therein, material properties are offered in physically motivated distributions of microstructure descriptors rather than as normally distributed data. Applied to porous fiber networks (paper) before and after unidirectional compression, it is shown that the copula-based models reveal material-characteristic relationships between two or more microstructure descriptors. In this way, the presented modeling approach can provide deeper insight into the microscopic origin of effective macroscopic properties of heterogeneous porous materials. This data-driven approach uses R-vine copulas to quantify and interpret relationships between five preselected local microstructure descriptors in porous materials. The R-vine copula models the 5D multivariate distribution of the local descriptors. Once built from data, the model provides material-specific relationships between all combinations of the five descriptors. image
更多查看译文
关键词
3D microstructures,complexity reduction,local heterogeneity,multivariate statistical data analysis,porous material,vine copula
AI 理解论文
溯源树
样例
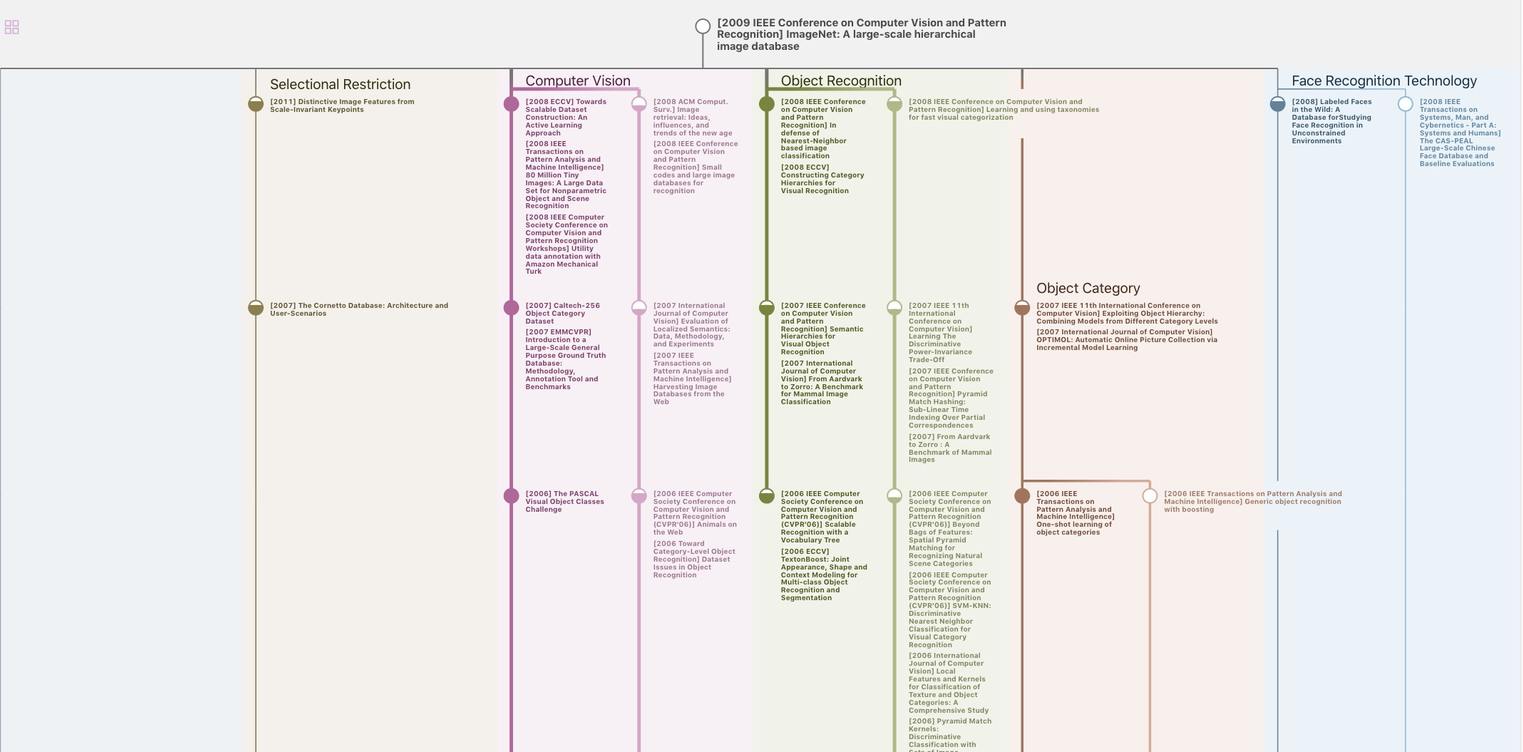
生成溯源树,研究论文发展脉络
Chat Paper
正在生成论文摘要