Physics-guided spatio-temporal neural network for predicting dissolved oxygen concentration in rivers
INTERNATIONAL JOURNAL OF GEOGRAPHICAL INFORMATION SCIENCE(2024)
摘要
The prediction of river water quality is key in water resource management. Data-driven machine learning models have been widely used for predicting river water quality. However, these models seldom consider the physical mechanisms of water quality variation, which degrades the accuracy and stability of the prediction results. Hence, we develop a physics-guided spatio-temporal neural network (PGSTNN) model to predict a critical parameter for water quality assessment, i.e. dissolved oxygen. Physical information regarding spatio-temporal interactions in a hydrological network is explicitly considered to construct the architecture of PGSTNN. Two physical rules of dissolved oxygen variation (i.e. Henry's law and power-scaling law) are established for the loss function of PGSTNN to guarantee the physical consistency of the prediction results. Experiments on the 2020-2021 water quality dataset in Atlanta, USA show that PGSTNN outperforms seven baseline neural network models in terms of prediction accuracy and stability. PGSTNN typically brings at least 10% accuracy (e.g. root mean square error and mean absolute error) improvement over the comparison methods. The proposed PGSTNN may not only improve the emergency response ability of water resource management, but also provide useful ideas for integrating scientific knowledge with machine learning.
更多查看译文
关键词
Water quality prediction,gated recurrent unit,physics mechanism,dissolved oxygen,machine learning
AI 理解论文
溯源树
样例
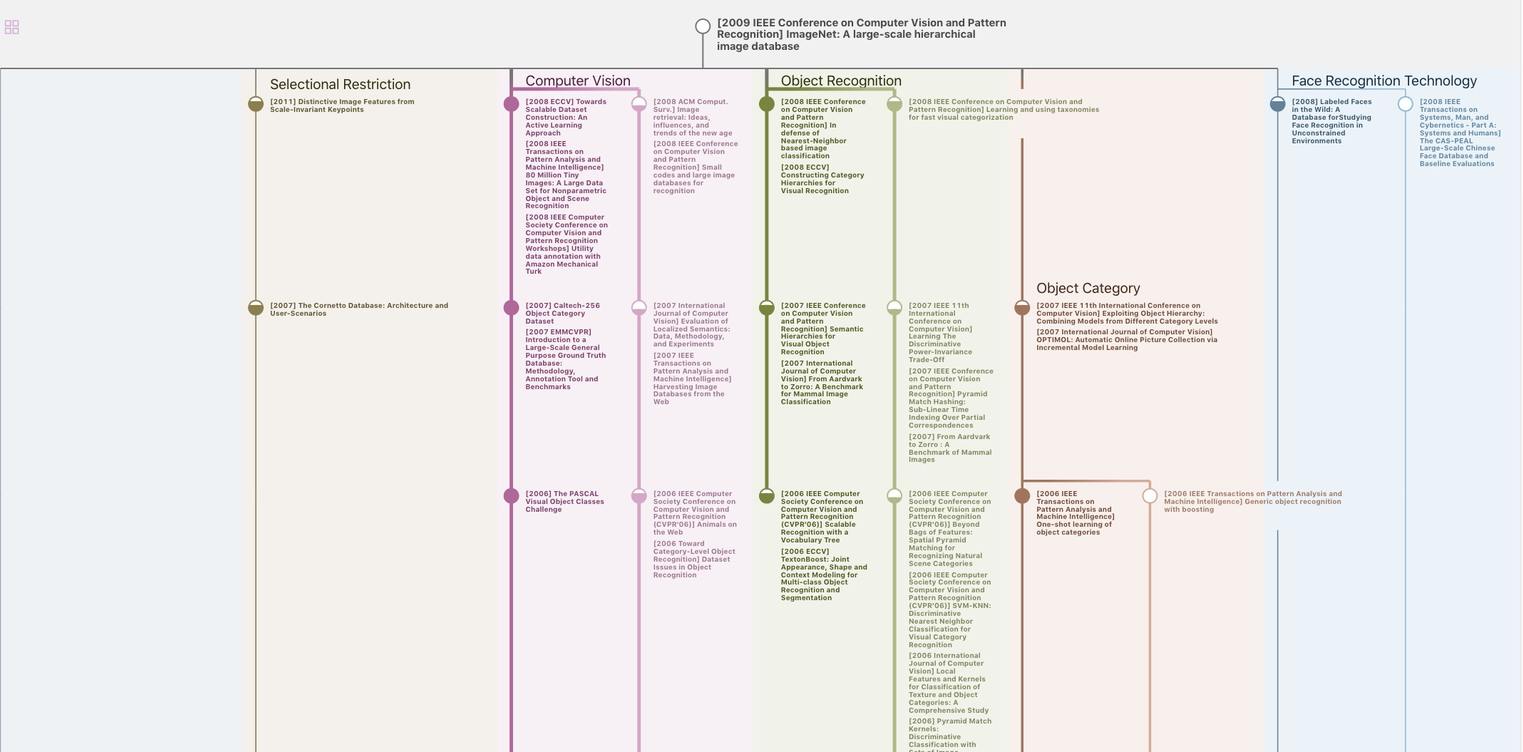
生成溯源树,研究论文发展脉络
Chat Paper
正在生成论文摘要