Towards Robust Ferrous Scrap Material Classification with Deep Learning and Conformal Prediction
Eng Appl Artif Intell(2025)
关键词
Scrap classification,Conformal prediction,Deep learning,Explainable artificial intelligence,Uncertainty quantification
AI 理解论文
溯源树
样例
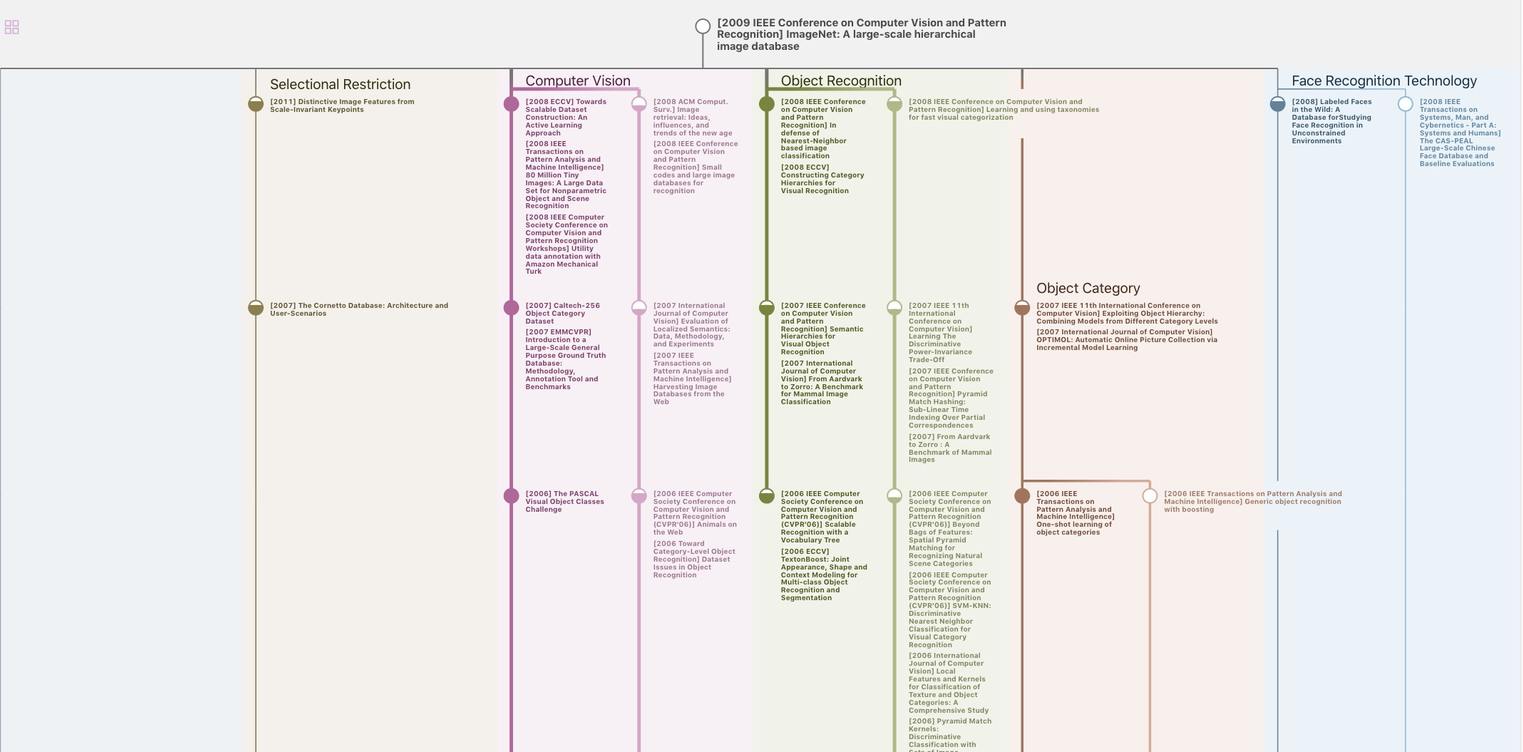
生成溯源树,研究论文发展脉络
Chat Paper
正在生成论文摘要