SOS-1K: A Fine-grained Suicide Risk Classification Dataset for Chinese Social Media Analysis
CoRR(2024)
摘要
In the social media, users frequently express personal emotions, a subset of
which may indicate potential suicidal tendencies. The implicit and varied forms
of expression in internet language complicate accurate and rapid identification
of suicidal intent on social media, thus creating challenges for timely
intervention efforts. The development of deep learning models for suicide risk
detection is a promising solution, but there is a notable lack of relevant
datasets, especially in the Chinese context. To address this gap, this study
presents a Chinese social media dataset designed for fine-grained suicide risk
classification, focusing on indicators such as expressions of suicide intent,
methods of suicide, and urgency of timing. Seven pre-trained models were
evaluated in two tasks: high and low suicide risk, and fine-grained suicide
risk classification on a level of 0 to 10. In our experiments, deep learning
models show good performance in distinguishing between high and low suicide
risk, with the best model achieving an F1 score of 88.39
for fine-grained suicide risk classification were still unsatisfactory, with an
weighted F1 score of 50.89
limited dataset size, we investigated both traditional and advanced, large
language model based data augmentation techniques, demonstrating that data
augmentation can enhance model performance by up to 4.65
Notably, the Chinese MentalBERT model, which was pre-trained on psychological
domain data, shows superior performance in both tasks. This study provides
valuable insights for automatic identification of suicidal individuals,
facilitating timely psychological intervention on social media platforms. The
source code and data are publicly available.
更多查看译文
AI 理解论文
溯源树
样例
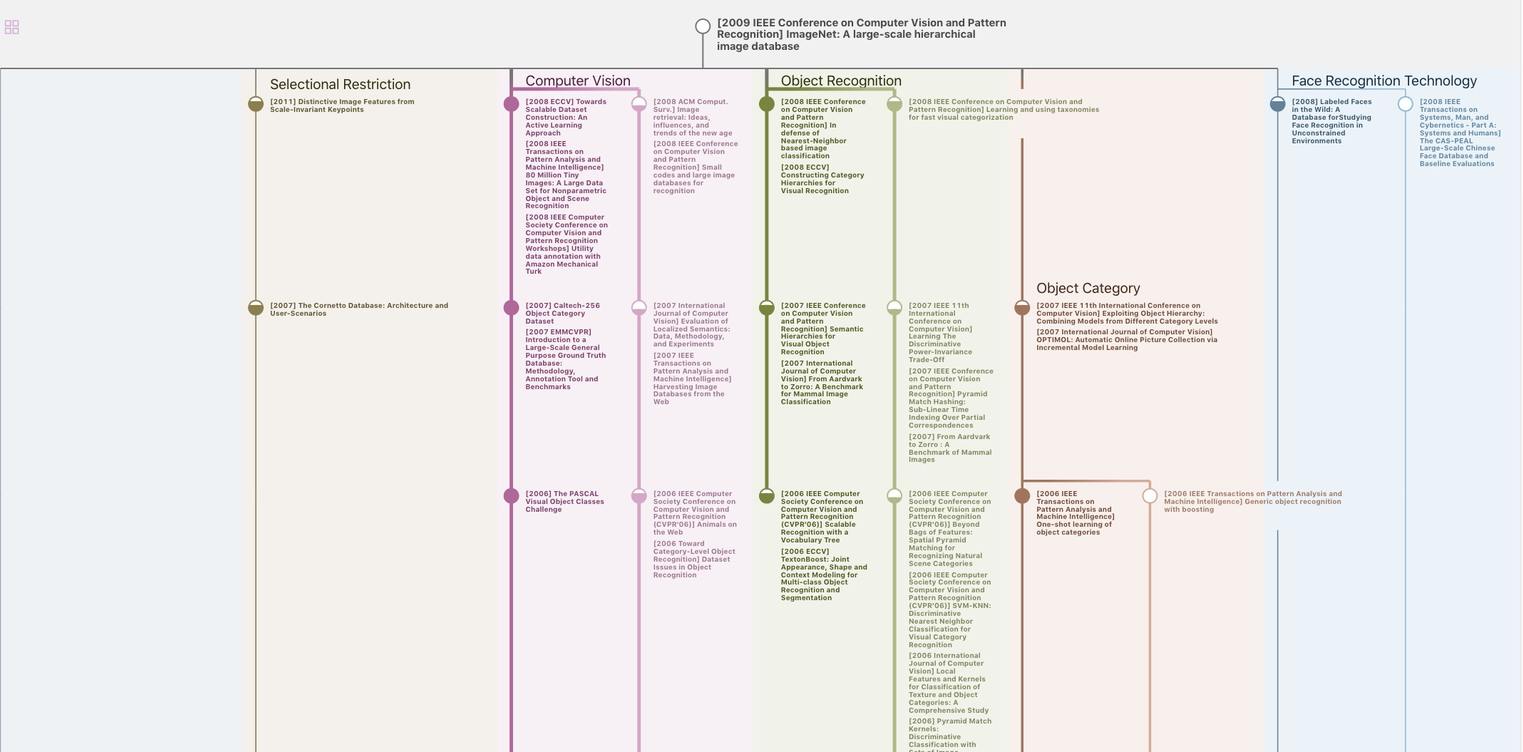
生成溯源树,研究论文发展脉络
Chat Paper
正在生成论文摘要