A novel diagnostic framework based on vibration image encoding and multi-scale neural network
EXPERT SYSTEMS WITH APPLICATIONS(2024)
摘要
Intelligent fault diagnosis employing CNN-based techniques has demonstrated promising results in rotating machinery maintenance and management. However, most approaches that rely on time-series vibration signals fail to incorporate the physical knowledge of faults into feature learning, and are subject to obtaining the correlation between input and output characteristics. Furthermore, it is challenging for the neural network to improve feature extraction due to the rigid convolution kernel and the limited receptive field. To address these issues, an intelligent fault diagnosis framework is developed based on vibration image encoding and multi-scale neural networks. Firstly, a novel image encoding rule is designed to convert time-series signals into vibration images, which incorporate the impact characteristics of faults into the model inputs to enhance fault information. Secondly, a multi-scale feature fusion network with dilatated and irregular sampling convolution block is constructed for feature extraction and classification. In addition, a new regularization term is designed to avoid training overfitting. Finally, Grad-CAM is introduced to visualize the region of interest of the model. The proposed method is verified on the datasets with different fault types from our laboratory and DIRG bearing dataset with different fault severities from Politecnico di Torino. The experimental results demonstrate that our diagnostic framework exhibits good generalization and noise robustness, and can achieve high-precision fault diagnosis.
更多查看译文
关键词
Fault diagnosis,Rolling bearing,T-GADF image encoding method,Multi -scale neural network
AI 理解论文
溯源树
样例
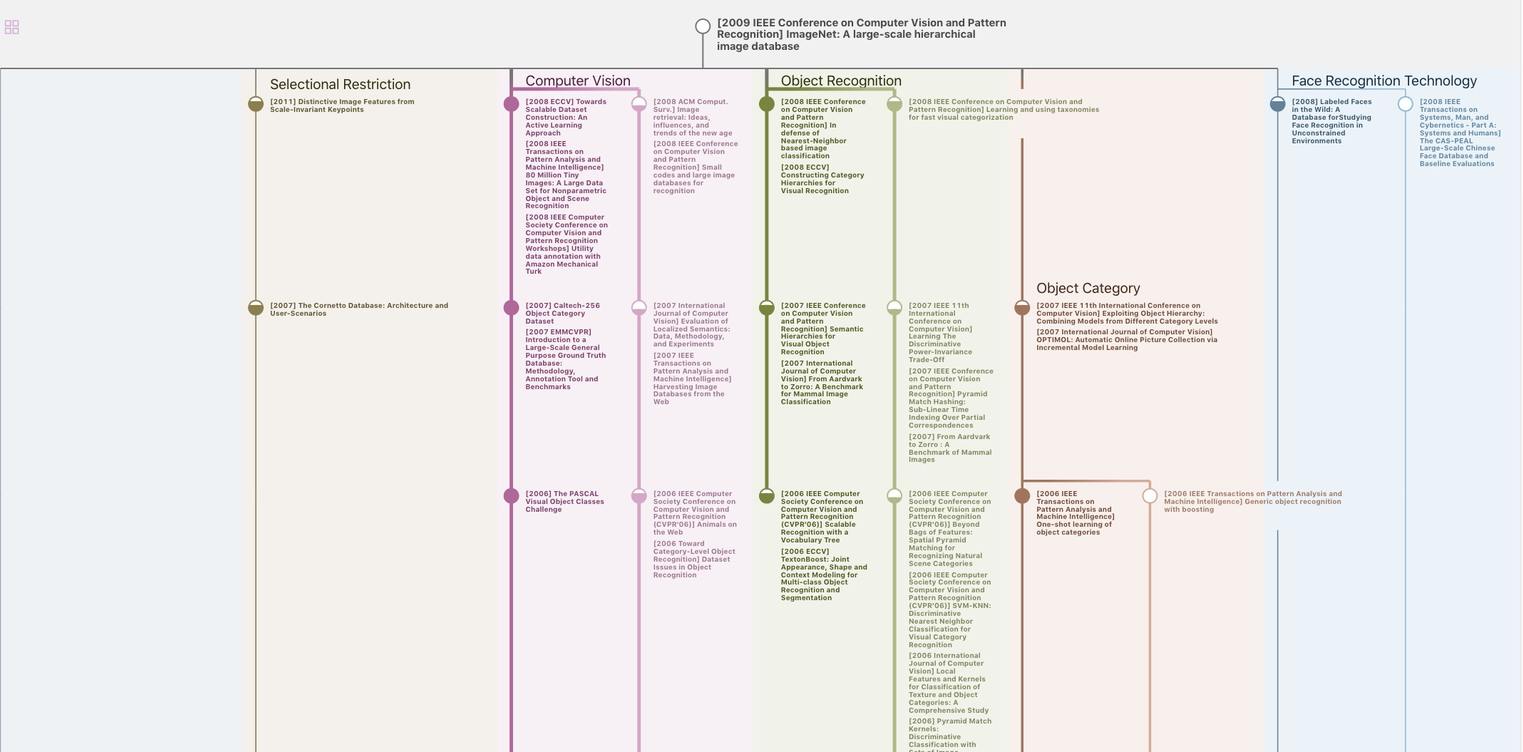
生成溯源树,研究论文发展脉络
Chat Paper
正在生成论文摘要