Simultaneous prediction of petrophysical properties and formation layered thickness from acoustic logging data using a modular cascading residual neural network (MCARNN) with physical constraints
Journal of Applied Geophysics(2024)
Abstract
Predicting petrophysical properties from logging data using deep neural networks (DNNs) improves the efficiency of subsurface exploration. Traditional methods, however, heavily rely on data pre-processing. Therefore, a novel Modular Cascading Residual Neural Network (MCARNN) is proposed to simultaneously retrieve layered thickness and petrophysical properties of porous media directly from raw acoustic logging data without pre-processing. The MCARNN mainly comprises two cascading modules: the first is tasked with retrieving layered thickness, while the second one further reconstructs petrophysical properties based on the thickness information. To more accurately simulate the uncertainties of the real world, we incorporate some known geophysical knowledge, including the distribution range of actual petrophysical parameters, physical constraints among porous medium parameters, configurations of logging instruments, and stratified geological models. During this process, all Biot's porous parameters are independently randomized to cover a wide range of geological rock types. Furthermore, to enhance MCARNN's generalization ability under different random noise distributions, we independently generate the training and testing datasets using distinct numerical algorithms, thus preventing the “inverse crime” phenomenon. Additionally, varying levels of Gaussian white noises are added to the training dataset, and the transmitter-receiver configuration of the logging instrument is varied to enhance the training dataset. Two numerical experiments are implemented to validate the reliability and superiority of the MCARNN. It is shown that the proposed MCARNN can not only accurately predict porous petrophysical parameters when the background layer configurations are known but also be able to simultaneously reconstruct layered thickness and petrophysical parameters with low model misfit. Notably, MCARNN successfully predicts the porous velocity dispersion curves within a broad frequency band based on limited frequency points—a task typically requiring auxiliary equations in traditional inversion methods, demonstrating the advanced predictive capability of the proposed MCARNN. Through this research, we not only address the deficiencies in existing real-world logging datasets in terms of sample volume and label reliability but also effectively integrate rock physics models with data-driven neural networks, providing a novel and effective pathway for reservoir parameter prediction in the field of geophysical logging.
MoreTranslated text
Key words
Acoustic logging,Porous parameters,Deep learning inversion,Noise robustness,Layered configurations
AI Read Science
Must-Reading Tree
Example
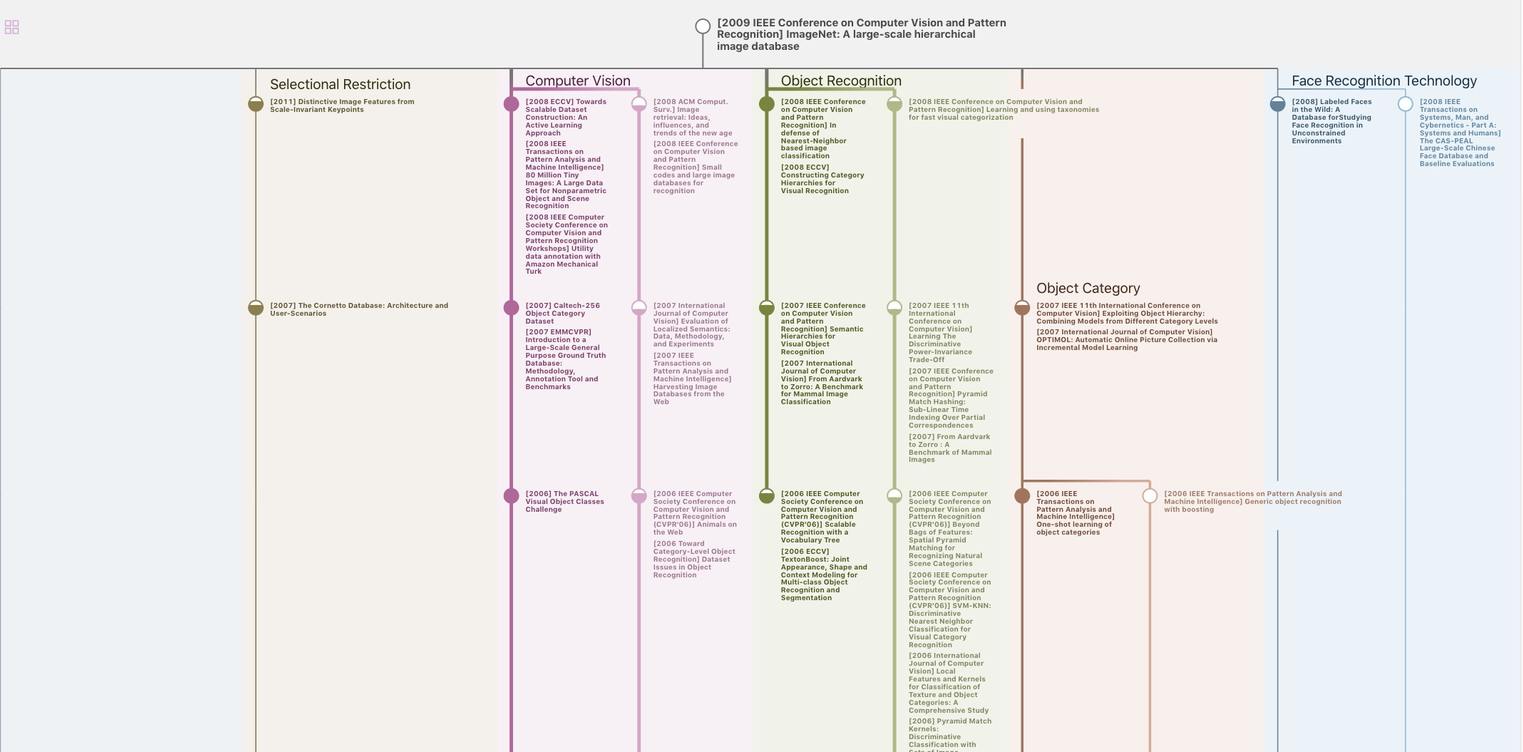
Generate MRT to find the research sequence of this paper
Chat Paper
Summary is being generated by the instructions you defined