Deep learning and LiDAR integration for surveillance camera-based river water level monitoring in flood applications
Natural Hazards(2024)
摘要
Recently, surveillance technology was proposed as an alternative to flood monitoring systems. This study introduces a novel approach to flood monitoring by integrating surveillance technology and LiDAR data to estimate river water levels. The methodology involves deep learning semantic segmentation for water extent extraction before utilizing the segmented images and virtual markers with elevation information from light detection and ranging (LiDAR) data for water level estimation. The efficiency was assessed using Spearman's rank-order correlation coefficient, yielding a high correlation of 0.92 between the water level framework with readings from the sensors. The performance metrics were also carried out by comparing both measurements. The results imply accurate and precise model predictions, indicating that the model performs well in closely matching observed values. Additionally, the semi-automated procedure allows data recording in an Excel file, offering an alternative measure when traditional water level measurement is not available. The proposed method proves valuable for on-site water-related information retrieval during flood events, empowering authorities to make informed decisions in flood-related planning and management, thereby enhancing the flood monitoring system in Malaysia.
更多查看译文
AI 理解论文
溯源树
样例
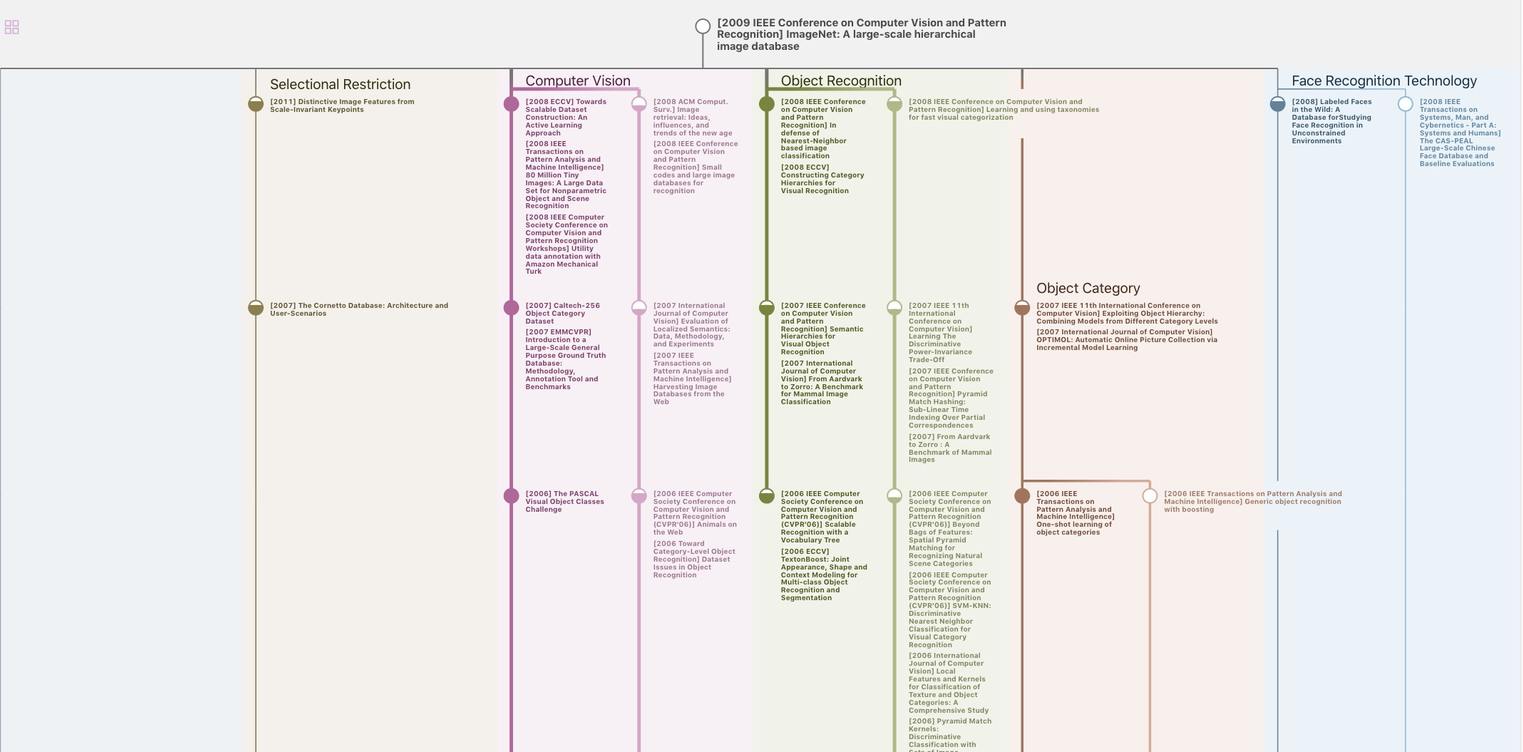
生成溯源树,研究论文发展脉络
Chat Paper
正在生成论文摘要