Improved feature extraction network in lightweight YOLOv7 model for real-time vehicle detection on low-cost hardware
Journal of Real-Time Image Processing(2024)
摘要
The advancement of unmanned aerial vehicles (UAVs) has drawn researchers to update object detection algorithms for better accuracy and computation performance. Previous works applying deep learning models for object detection applications required high graphics processing unit (GPU) computation power. Generally, object detection models suffer trade-off between accuracy and model size where the relationship is not always linear in deep learning models. Various factors such as architectural design, optimization techniques, and dataset characteristics can significantly influence the accuracy, model size, and computation cost in adopting object detection models for low-cost embedded devices. Hence, it is crucial to employ lightweight object detection models for real-time object identification for the solution to be sustainable. In this work, an improved feature extraction network is proposed by incorporating an efficient long-range aggregation network for vehicle detection (ELAN-VD) in the backbone layer. The architecture improvement in YOLOv7-tiny model is proposed to improve the accuracy of detecting small vehicles in the aerial image. Besides that, the image size output of the second and third prediction boxes is upscaled for better performance. This study showed that the proposed method yields a mean average precision (mAP) of 57.94
更多查看译文
关键词
Image,Object detection,Vehicle detection,YOLO
AI 理解论文
溯源树
样例
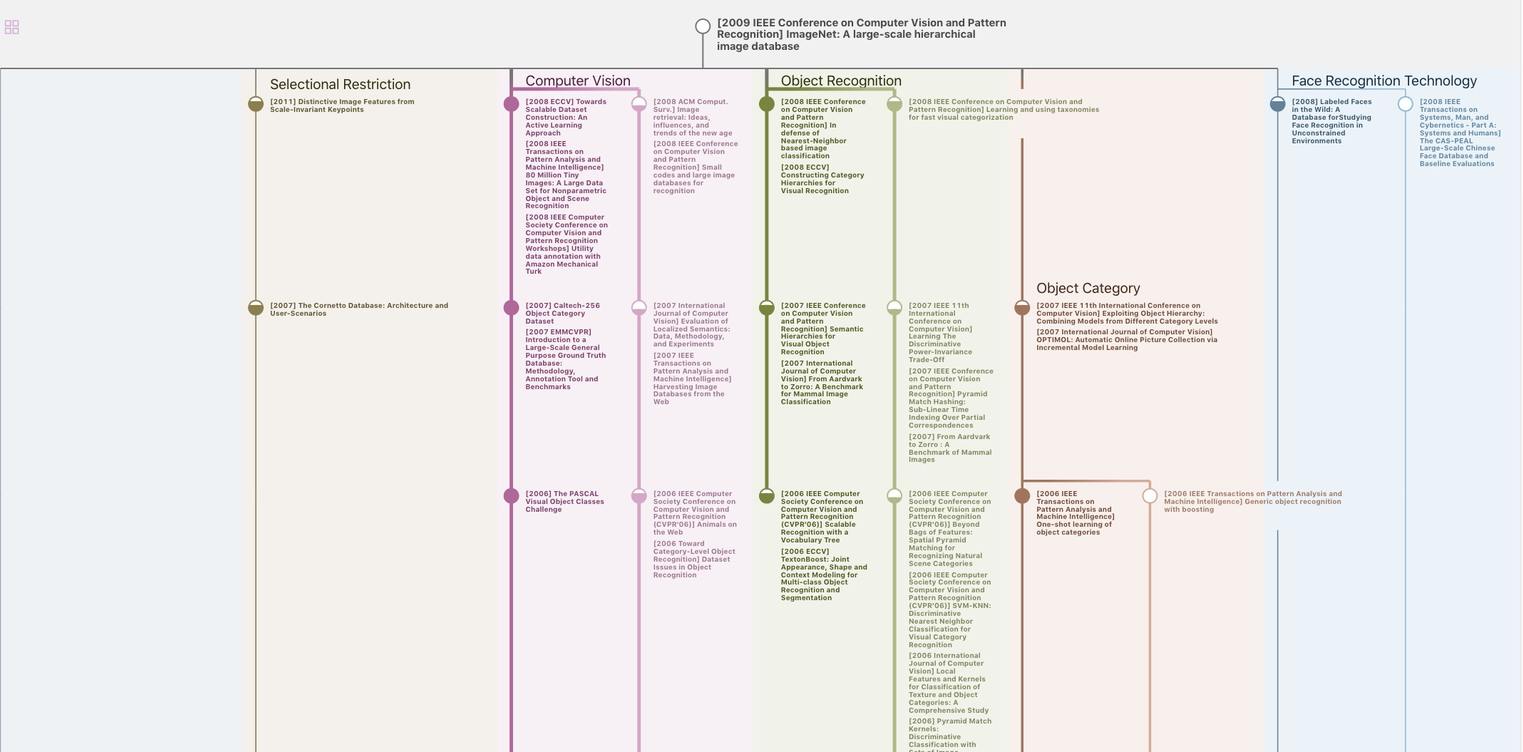
生成溯源树,研究论文发展脉络
Chat Paper
正在生成论文摘要