ABAE: Auxiliary Balanced AutoEncoder for class-imbalanced semi-supervised learning
Pattern Recognition Letters(2024)
摘要
Semi-supervised learning has achieved extraordinary success in prevalent image-classification benchmarks. However, a class-balanced distribution that differs notably from real-world data distribution is required. In general, models trained under class-imbalanced semi-supervised learning conditions are severely biased towards the majority classes. To address this issue, we propose a novel framework called ABAE by implanting an Auxiliary Balanced AutoEncoder branch into existing semi-supervised learning algorithms. Considering that adaptive feature augmentation for different classes can alleviate confirmation bias, we devise a class-aware reconstruction loss to train the AutoEncoder module. To further smooth the output, we adopt a graph-based label propagation scheme at the end of the AutoEncoder. Extensive experiments on CIFAR-10/100-LT, SVHN-LT and Small ImageNet-127 demonstrate the effectiveness of ABAE.
更多查看译文
关键词
Class-imbalanced learning,Semi-supervised learning,Autoencoder,Label propagation
AI 理解论文
溯源树
样例
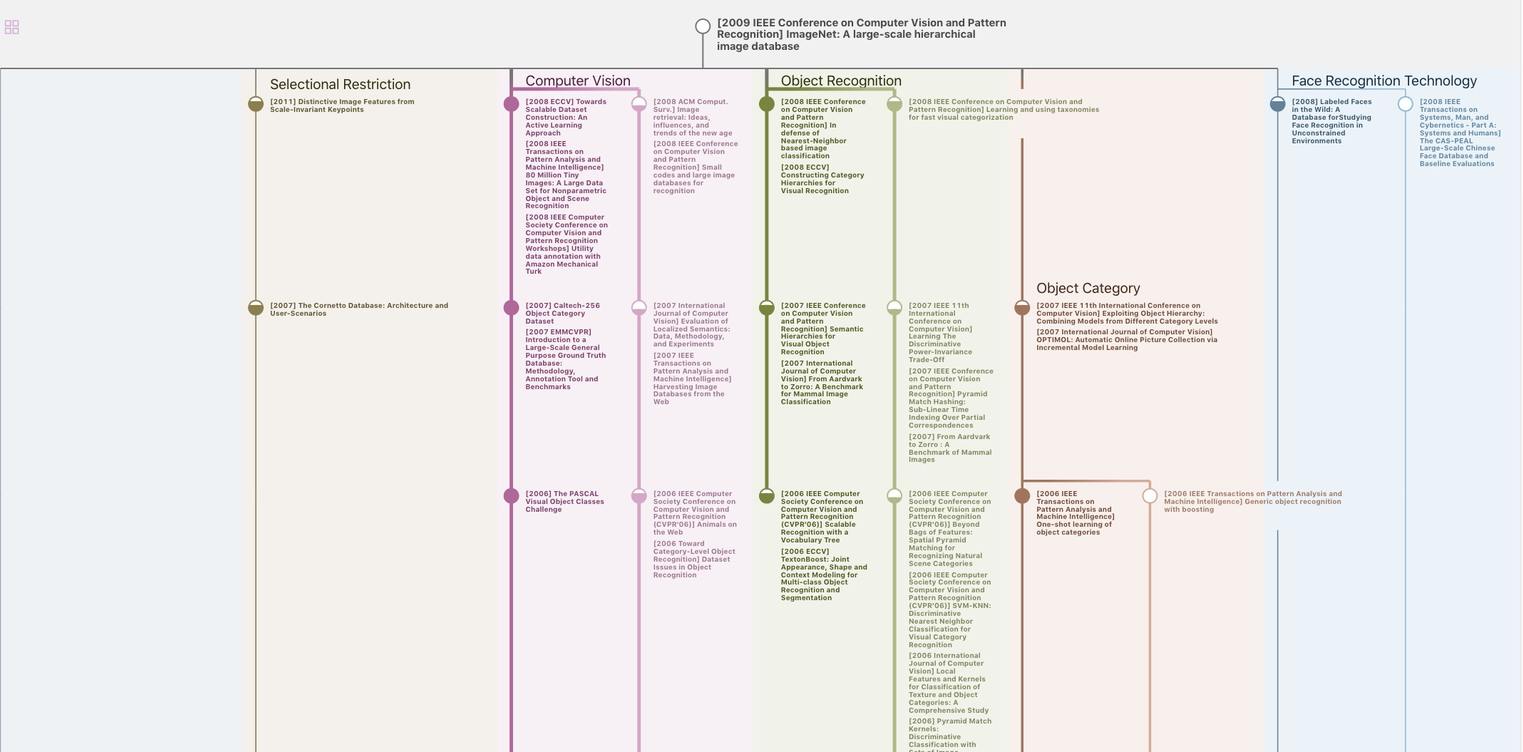
生成溯源树,研究论文发展脉络
Chat Paper
正在生成论文摘要