Multi-scale full spike pattern for semantic segmentation
NEURAL NETWORKS(2024)
Abstract
Spiking neural networks (SNNs), as the brain-inspired neural networks, encode information in spatio-temporal dynamics. They have the potential to serve as low-power alternatives to artificial neural networks (ANNs) due to their sparse and event-driven nature. However, existing SNN-based models for pixel-level semantic segmentation tasks suffer from poor performance and high memory overhead, failing to fully exploit the computational effectiveness and efficiency of SNNs. To address these challenges, we propose the multi-scale and full spike segmentation network (MFS-Seg), which is based on the deep direct trained SNN and represents the first attempt to train a deep SNN with surrogate gradients for semantic segmentation. Specifically, we design an efficient fully-spike residual block (EFS-Res) to alleviate representation issues caused by spiking noise on different channels. EFS-Res utilizes depthwise separable convolution to improve the distributions of spiking feature maps. The visualization shows that our model can effectively extract the edge features of segmented objects. Furthermore, it can significantly reduce the memory overhead and energy consumption of the network. In addition, we theoretically analyze and prove that EFS-Res can avoid the degradation problem based on block dynamical isometry theory. Experimental results on the Camvid dataset, the DDD17 dataset, and the DSEC-Semantic dataset show that our model achieves comparable performance to the mainstream UNet network with up to 31 x fewer parameters, while significantly reducing power consumption by over 13 x . Overall, our MFS-Seg model demonstrates promising results in terms of performance, memory efficiency, and energy consumption, showcasing the potential of deep SNNs for semantic segmentation tasks. Our code is available in https://github.com/BICLab/MFS-Seg.
MoreTranslated text
Key words
Spiking neural network,Semantic segmentation,Neuromorphic computing,Deep neural network,Energy efficiency,Brain-inspired computing
AI Read Science
Must-Reading Tree
Example
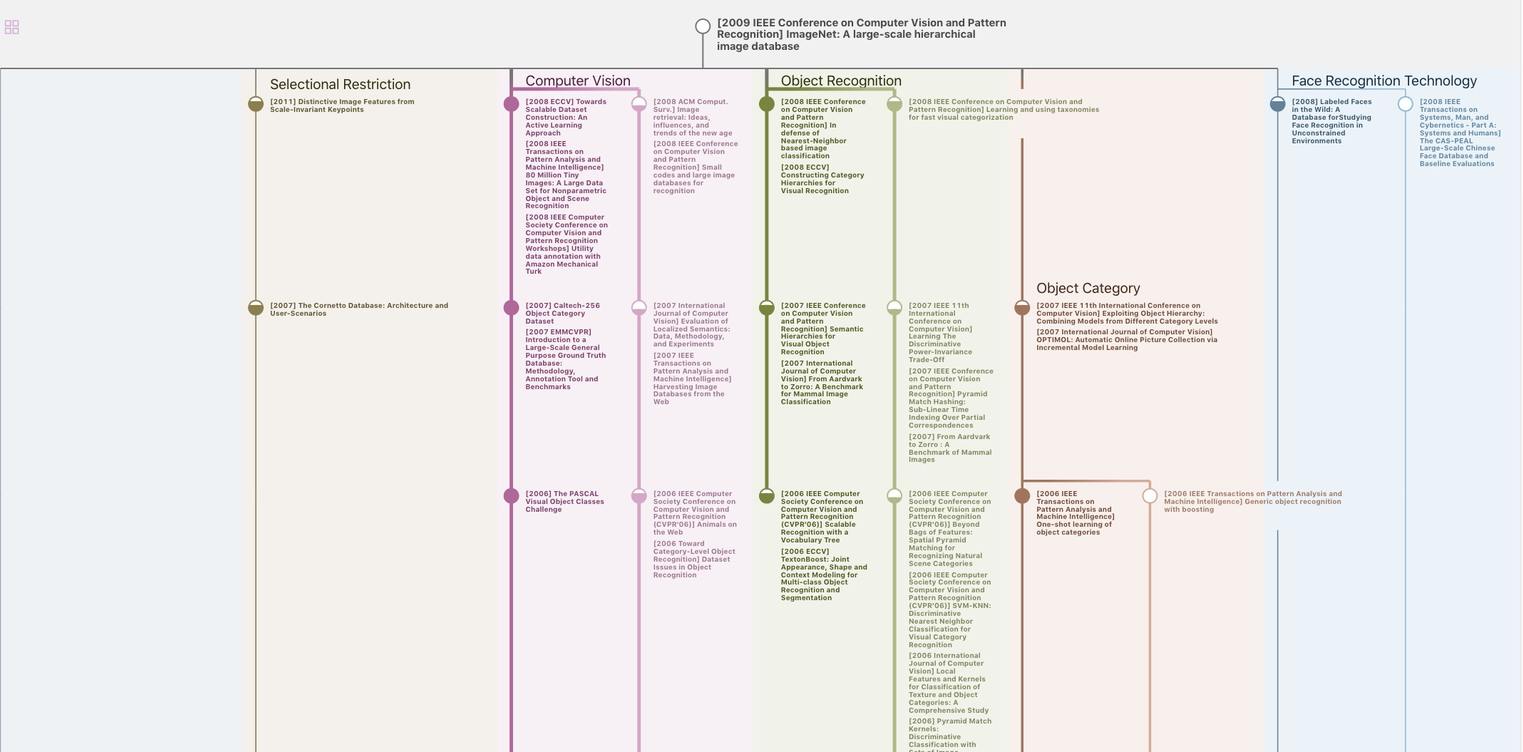
Generate MRT to find the research sequence of this paper
Chat Paper
Summary is being generated by the instructions you defined