A dynamic multi-model transfer based short-term load forecasting
APPLIED SOFT COMPUTING(2024)
Abstract
The integration of renewable energy sources in power systems has resulted in increased complexity in dispatch management, necessitating higher accuracy in short-term load forecasting. Although numerous deep learning models have been developed, their training data requirements and difficulties in effectively combining different model structures remain challenges. This study proposes a multi -model transfer -based approach for dynamic short-term load forecasting to address these issues. The method consists of two phases: (1) conducting correlation analysis to determine transferability between datasets and implementing an adaptive parameter transfer policy to optimize data utilization and training efficiency, and (2) constructing a multi -model cooperation platform to fully leverage the strengths of different models and enhance forecasting accuracy. Experimental case studies demonstrate the effectiveness of the proposed method in improving forecasting accuracy when historical load data is limited. This research contributes to advancing load forecasting techniques by leveraging transfer learning and model cooperation, facilitating efficient power system operations in the context of renewable energy integration.
MoreTranslated text
Key words
Transfer learning,Reinforcement learning,Dynamic model selection,Neural network,Short-term load forecasting
AI Read Science
Must-Reading Tree
Example
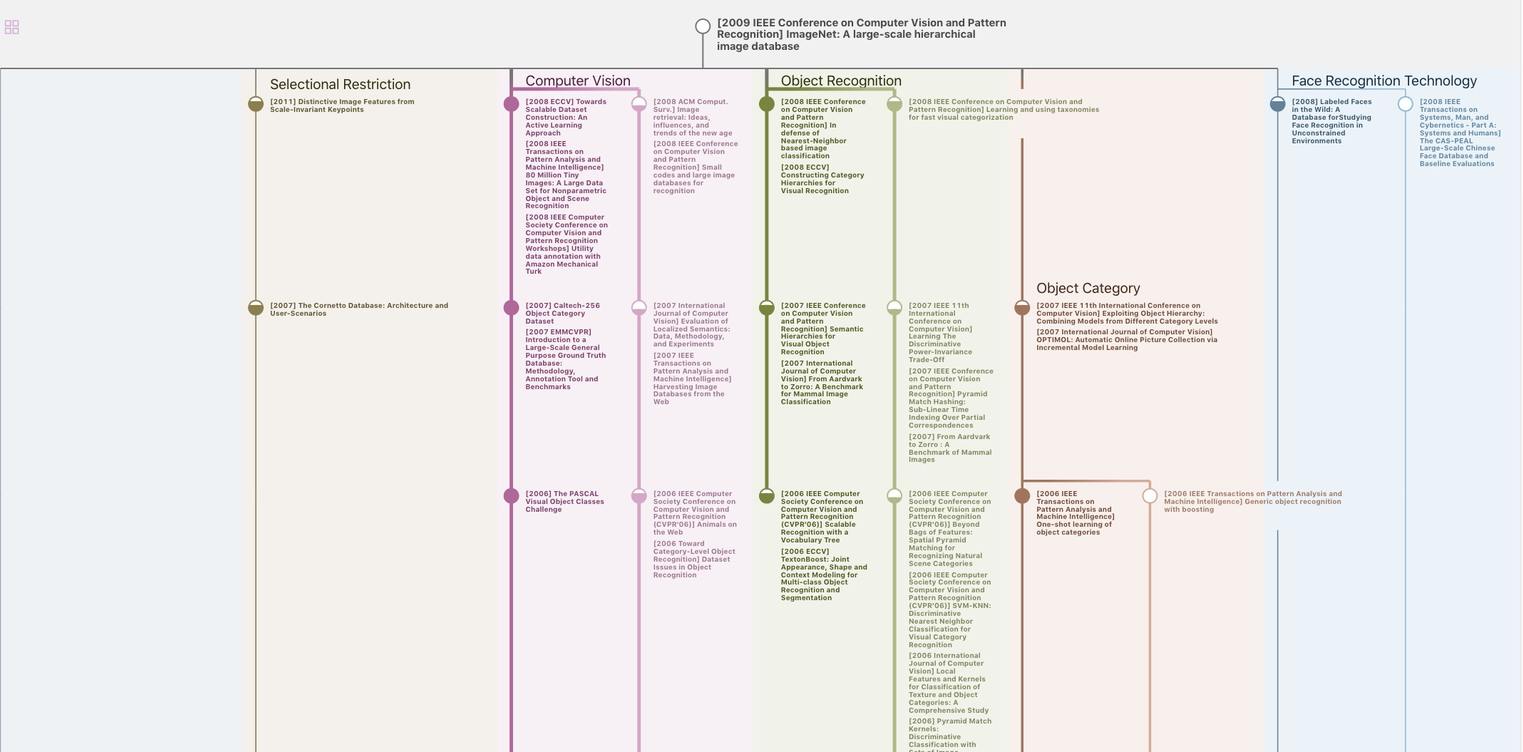
Generate MRT to find the research sequence of this paper
Chat Paper
Summary is being generated by the instructions you defined