0306 Improving Accessibility and Minimizing Errors: Reliable Sleep Stage Classification Using Wearable Sensors
SLEEP(2024)
摘要
Abstract Introduction The widespread use of wearable devices has led to the emerging trend to quantify sleep. However, concerns arise due to the potential inaccuracies in these devices, which even can result in adverse daytime consequences. Here, we present an automatized, low-cost, deep learning alternative to PSG sleep staging that provides a reliable epoch-by-epoch classification in four classes (Wake, Light [N1 + N2], Deep, REM) and solely relies on inter-beat-interval (IBI) HRV data. Methods We trained a multi-resolution convolutional neural network (MCNN) on the IBIs of 8898 manually sleep-staged full-night (PSG) recordings and tested the MCNN for classification accuracy using two affordable consumer wearables: an optical heart rate sensor using photoplethysmography (PPG-VS) and an ECG breast belt (H10). Our most recent study tests the model on 136 self-reported poor sleepers equipped with PSG and these wearables. Results Results indicate promising outcomes with high epoch-by-epoch accuracy for ECG (accuracy: 86.3%, κ = 0.79), the H10 ECG (84.4%, κ = 0.76), and the PPG-VS wearable (84.2%, κ = 0.75). The HRV-based model exhibits substantial correlations and agreement with PSG on key sleep parameters, e.g., for Sleep Onset Latency (ρ = 0.82, p < 0.001), Sleep Efficiency (ρ = 0.84, p < 0.001), Total Sleep Time (ρ = 0.94, p < 0.001) and REM sleep (ρ = 0.78, p < 0.001). Notably, the model accurately classifies sleep also among users on heart-affecting or psychoactive medication; a critical aspect for potential clinical use. A first RCTs using this algorithm in our App “Nukkuaa” tested effects of i) daily objective sleep feedback using such sensors in combination with ii) tailored sleep training for insomnia patients using digital cognitive behavioural therapy (dCBT-I) and revealed subjective as well as objective improvements. Conclusion The present validation underscores the potential of automatic sleep stage classification algorithms based on cost-effective wearables and paves the way for scientific and clinical applications which strive for continuous and reliable sleep measurements including epoch-by-epoch sleep stage information as known from traditional PSG. Support (if any) The study was supported by the county of Salzburg (20102-F2002176-FPR) and the FWF/Austrian Science Fund (W 1233-B) for P. Topalidis.
更多查看译文
AI 理解论文
溯源树
样例
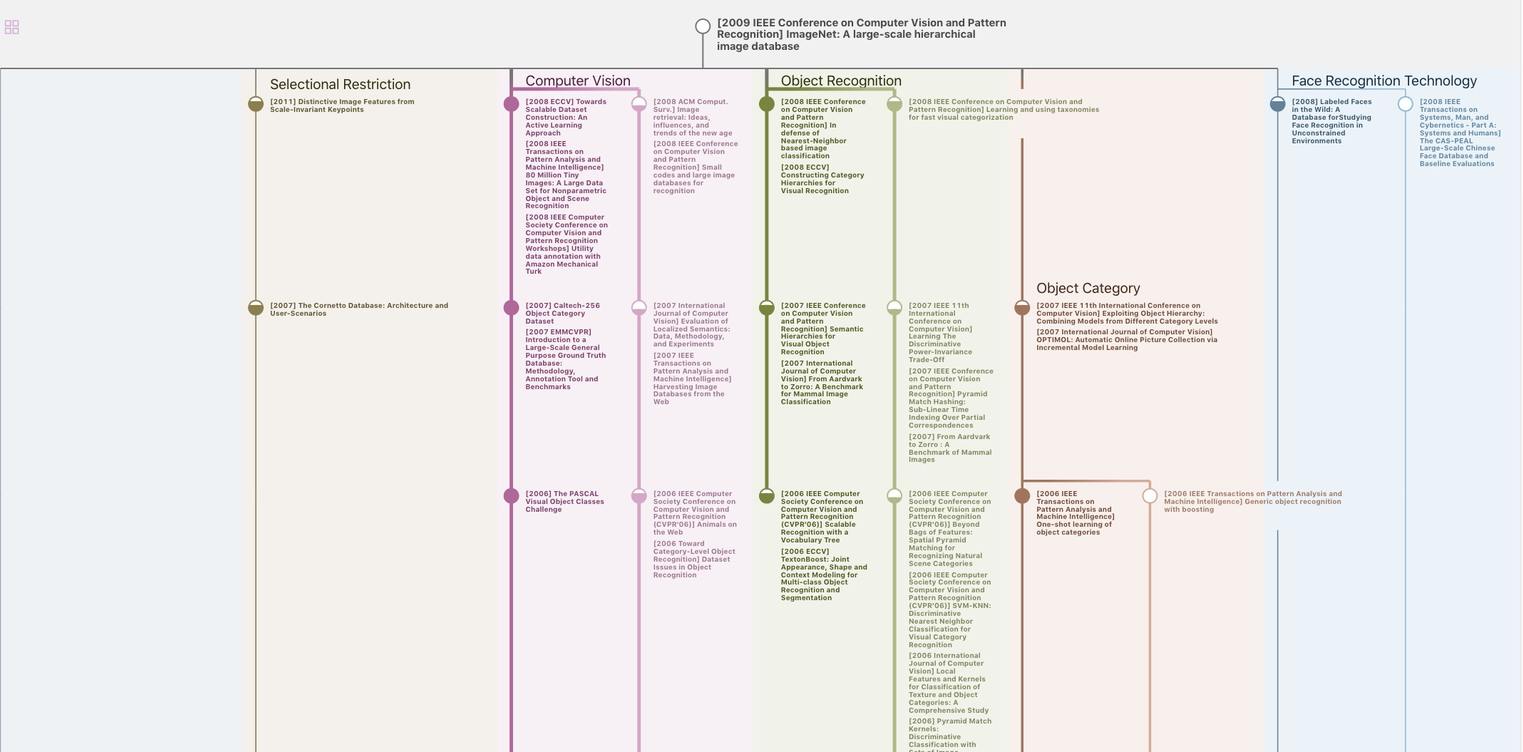
生成溯源树,研究论文发展脉络
Chat Paper
正在生成论文摘要