0294 Performance of Commercial Wearable Devices for Tracking Irregular Sleep Schedules
SLEEP(2024)
摘要
Abstract Introduction Many new popular commercial wearable devices perform well at tracking sleep/wake patterns, often equaling or exceeding the sleep-tracking performance of research-grade actigraphy. However, commercial wearables have not been subjected to extensive testing under irregular sleep schedules, such as split sleep and daytime sleep/naps, common in shift-working and operational populations. Therefore, to continue uncovering their real-world strengths/limitations, we evaluated the sleep-tracking performance of commercial wearables in people with irregular sleep schedules. Methods Participants were 12 healthy young adults (6 men, 6 women; 28.3±4.8 years, mean±SD) with habitual irregular sleep schedules, often due to shift work, sleeping or napping during daytime hours at least twice weekly. They completed sleep diaries and used actigraphy (Philips Respironics Actiwatch 2) and a set of wearables for 1 week under unrestricted home sleep conditions. Wearables included the Garmin Fenix 7 (GF) and Oura Rings (OR; generations 2 and 3). Device performance was compared with an electroencephalography headband (Dreem 3; Beacon Biosignals) and sleep diaries. Analyses included epoch-by-epoch agreement (sensitivity [for sleep] and specificity [for wake]) and summary bias comparisons for time in bed (TIB) and total sleep time (TST). Results Device sensitivity and specificity, respectively, were Actiwatch (0.92, 0.44), GF (0.96, 0.35), and OR (Gen-2: 0.92, 0.64; Gen-3: 0.93, 0.60). Device mean biases for TIB and TST were low for Actiwatch and both ORs but were higher and with significant proportional bias for GF. This appeared to be driven by GF combining multiple sleep episodes into single long episodes, which produced very long sleep recordings on those days when split sleep occurred. Conclusion Overall device sensitivity was high and specificity was low to medium, similar to previously known performance limitations of actigraphy and commercial wearables. However, OR had ~20% higher specificity than in our previous testing, suggesting improved wake-tracking performance in newer OR models/algorithms. Like previous GF models we tested, GF again underperformed in specificity and summary TST tracking. This underperformance raises concerns for real-world uses of GF with its current algorithm, especially regarding the finding that extreme summary bias values sometimes occurred when GF combined multiple sleep episodes/naps during split sleep schedules. Support (if any) Office of Naval Research, Code 34
更多查看译文
AI 理解论文
溯源树
样例
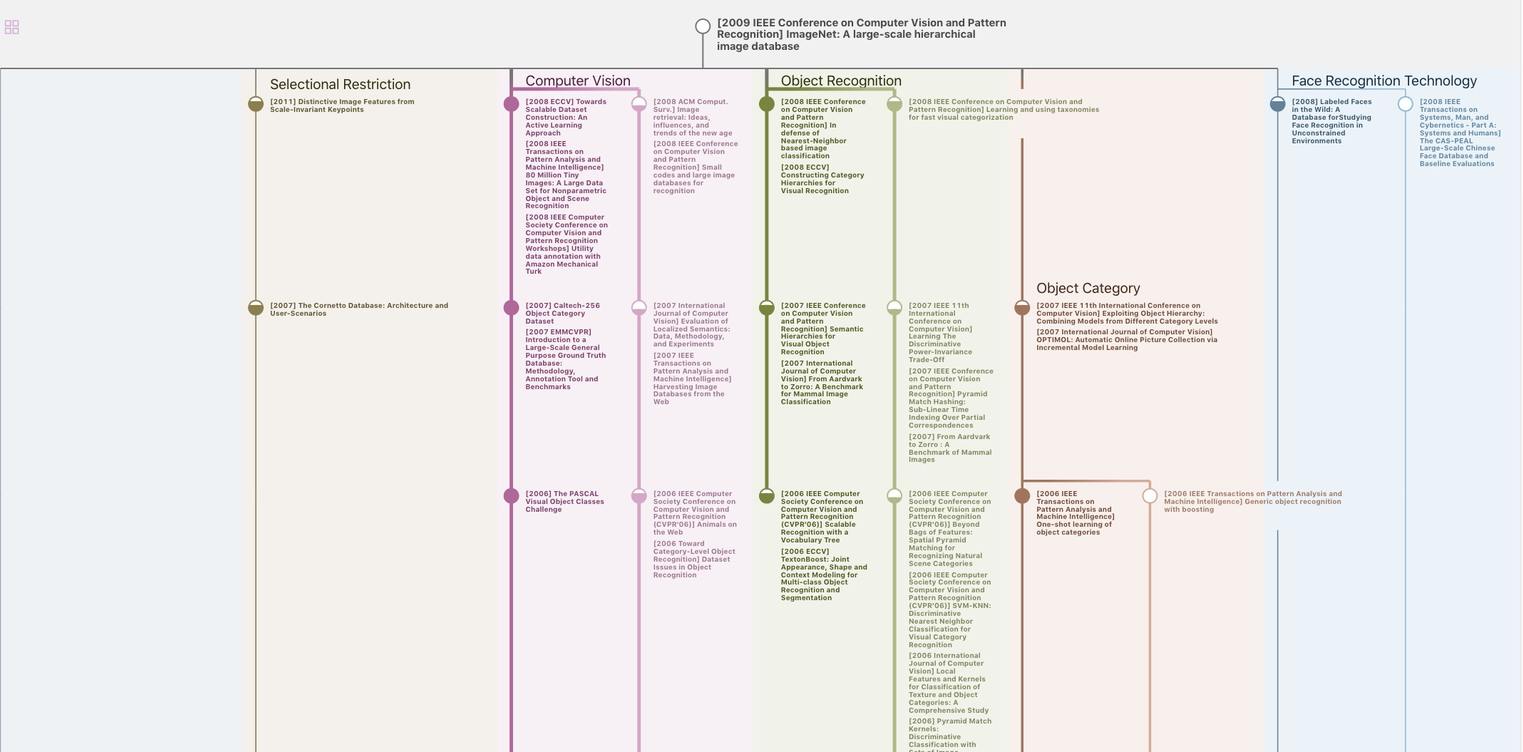
生成溯源树,研究论文发展脉络
Chat Paper
正在生成论文摘要