Automatic Signal Denoising and Multi-Component Fault Classification Based on Deep Learning Using Integrated Condition Monitoring in a Wind Turbine Gearbox
Journal of Vibration Engineering & Technologies(2024)
Abstract
This study aims to develop an automated method for signal denoising and fault classification in wind turbine gearboxes, known for operating under fluctuating loads and having significant downtime per failure. The approach leverages autoencoders to enhance the reliability and efficiency of condition monitoring (CM) systems by addressing challenges related to raw signal noise and the necessity for manual feature extraction. The proposed methodology begins with applying Variable Mode Decomposition (VMD) to raw vibration and acoustic signals to isolate noise components effectively. Subsequently, Denoising Autoencoders (DAEs) are trained to refine these signals automatically. Upon successful denoising, a Classification Autoencoder categorises the reconstructed signals into fault categories. This dual-phase autoencoder system (DAEs followed by a Classification Autoencoder) is evaluated on its precise ability to denoise and classify. The performance of the DAEs and the Classification Autoencoder is meticulously assessed based on signal-to-noise ratio (SNR), mean square error (MSE), and classification accuracy metrics. The developed DAEs demonstrated an SNR of 14.5 and 18.5 and an MSE of 0.005 and 0.46 for the validation datasets of vibration and acoustic signals, respectively. The Classification Autoencoder achieved an average test accuracy of 97.33
MoreTranslated text
Key words
Denoising,Deep learning,Autoencoders,Condition monitoring,Wind turbine gearbox
AI Read Science
Must-Reading Tree
Example
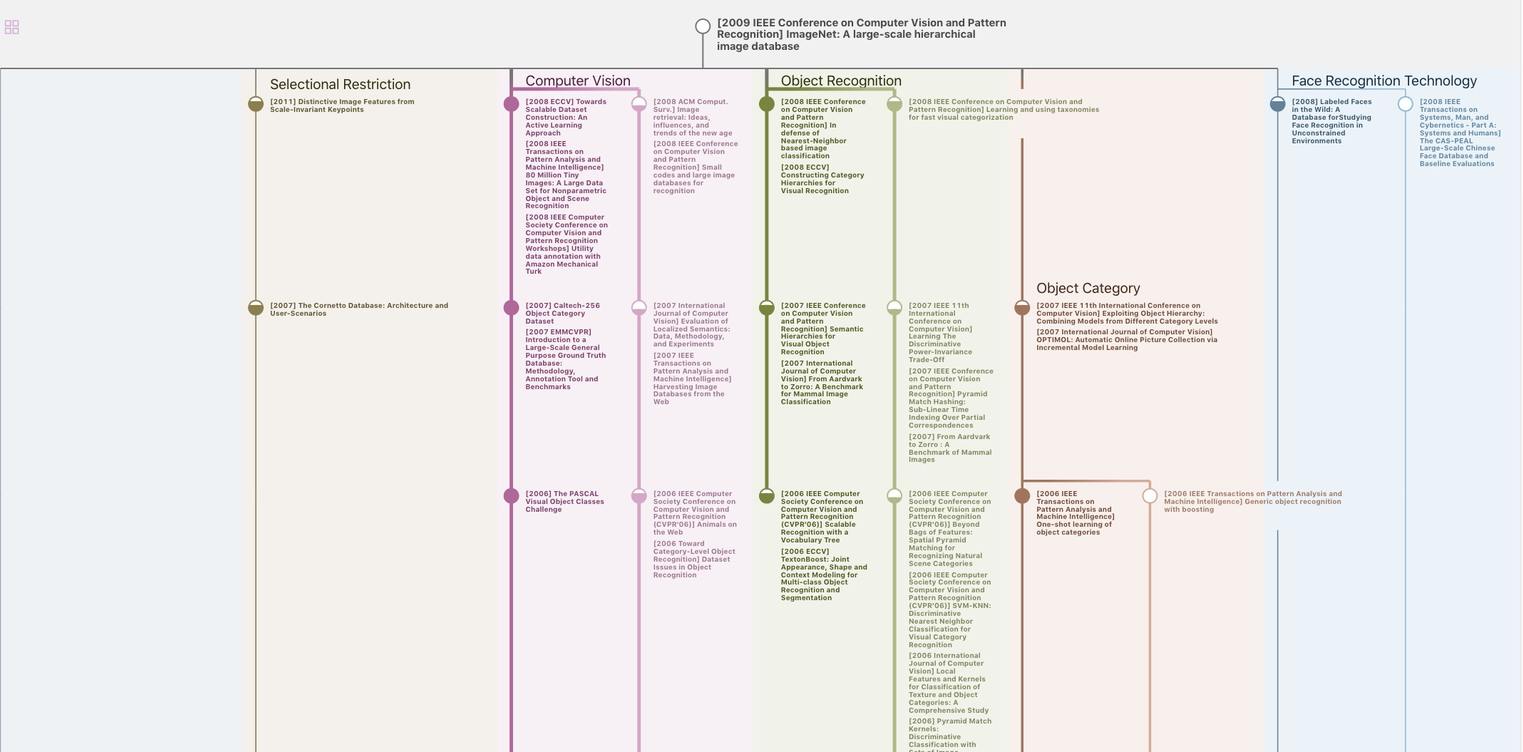
Generate MRT to find the research sequence of this paper
Chat Paper
Summary is being generated by the instructions you defined