0277 Robust Identification of Sleep States and Their Transitions Using Whole-Night fMRI Data
SLEEP(2024)
Abstract
Abstract Introduction Polysomnography (PSG)-based sleep staging has a relatively poor (30s) temporal resolution, and as a result does not capture any sleep structure that may exist on finer time-scales. For example, brain activity patterns involved with transitions or changes within and between classical sleep stages are not captured. Here we used whole-night functional magnetic resonance imaging (fMRI) to address this shortcoming. Methods Data acquisition was conducted as part of a well-controlled sleep experiment with two consecutive nights of concurrent fMRI-EEG recording from 23:00 to 7:00. Twelve participants (18-35 years old, 8 females) had at least one complete sleep cycle during both Night 1 and 2. We analyzed the fMRI data using an unsupervised learning approach - Hidden Markov Model (HMM) to reveal recurrent structure (“states”). The HMM, applied to Night 2 data, encompassed 21 whole-brain states based on various model evaluation indexes. Each state was characterized as a multivariate Gaussian distribution including a mean fMRI activation distribution,a functional connectivity (FC) matrix, and a transition probability matrix. Results Modular analysis of the extracted transition probability matrix (between each pair of states) revealed five modules, each containing a set of states that had similar preferences to occur during a specific sleep stage or part thereof. Remarkably, the trained HMM predicted brain sleep states during Night 1 that had highly similar sleep stage preference (r = 0.94, p < 0.001). In Wake-related HMM states, the FCs between DMN and Salience Network (SAL) / Control Network (CON) were negative, while during N3-related HMM states, these FCs were positive. Similar patterns were found for mean fMRI activation. Conclusion These observations suggest that HMM may provide valuable information about sleep-state-specific brain activity patterns that extend well beyond the information provided by classical sleep staging. For example, cursory inspection of the HMM results suggests a subdivision of N2 in two groups of multiple states with different transition probabilities to Wake; analysis of brain activation and FC patterns of HMM states indicated that the connections between DMN and SAL/CON may play a critical role in the transition from wake to light sleep and, to deep sleep. Support (if any)
MoreTranslated text
AI Read Science
Must-Reading Tree
Example
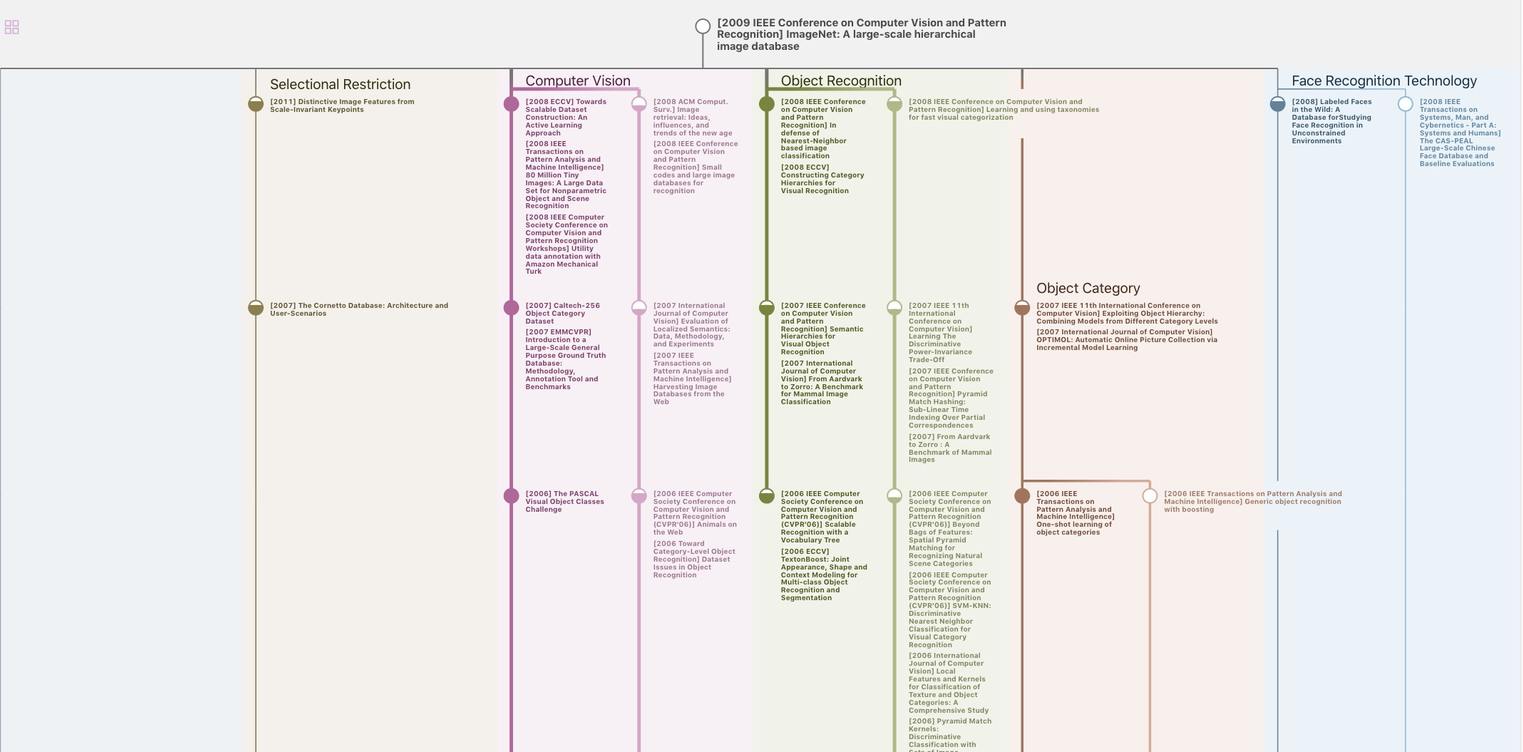
Generate MRT to find the research sequence of this paper
Chat Paper
Summary is being generated by the instructions you defined