Canopy-Level Spectral Variation and Classification of Diverse Crop Species with Fine Spatial Resolution Imaging Spectroscopy
REMOTE SENSING(2024)
摘要
With the increasing availability and volume of remote sensing data, imaging spectroscopy is an expanding tool for agricultural studies. One of the fundamental applications in agricultural research is crop mapping and classification. Previous studies have mostly focused at local to regional scales, and classifications were usually performed for a limited number of crop types. Leveraging fine spatial resolution (60 cm) imaging spectroscopy data collected by the Global Airborne Observatory (GAO), we investigated canopy-level spectral variations in 16 crop species from different agricultural regions in the U.S. Inter-specific differences were quantified through principal component analysis (PCA) of crop spectra and their Euclidean distances in the PC space. We also classified the crop species using support vector machines (SVM), demonstrating high classification accuracy with a test kappa of 0.97. A separate test with an independent dataset also returned high accuracy (kappa = 0.95). Classification using full reflectance spectral data (320 bands) and selected optimal wavebands from the literature resulted in similar classification accuracies. We demonstrated that classification involving diverse crop species is achievable, and we encourage further testing based on moderate spatial resolution imaging spectrometer data.
更多查看译文
关键词
agriculture,crop classification,support vector machine,Global Airborne Observatory,imaging spectroscopy,hyperspectral
AI 理解论文
溯源树
样例
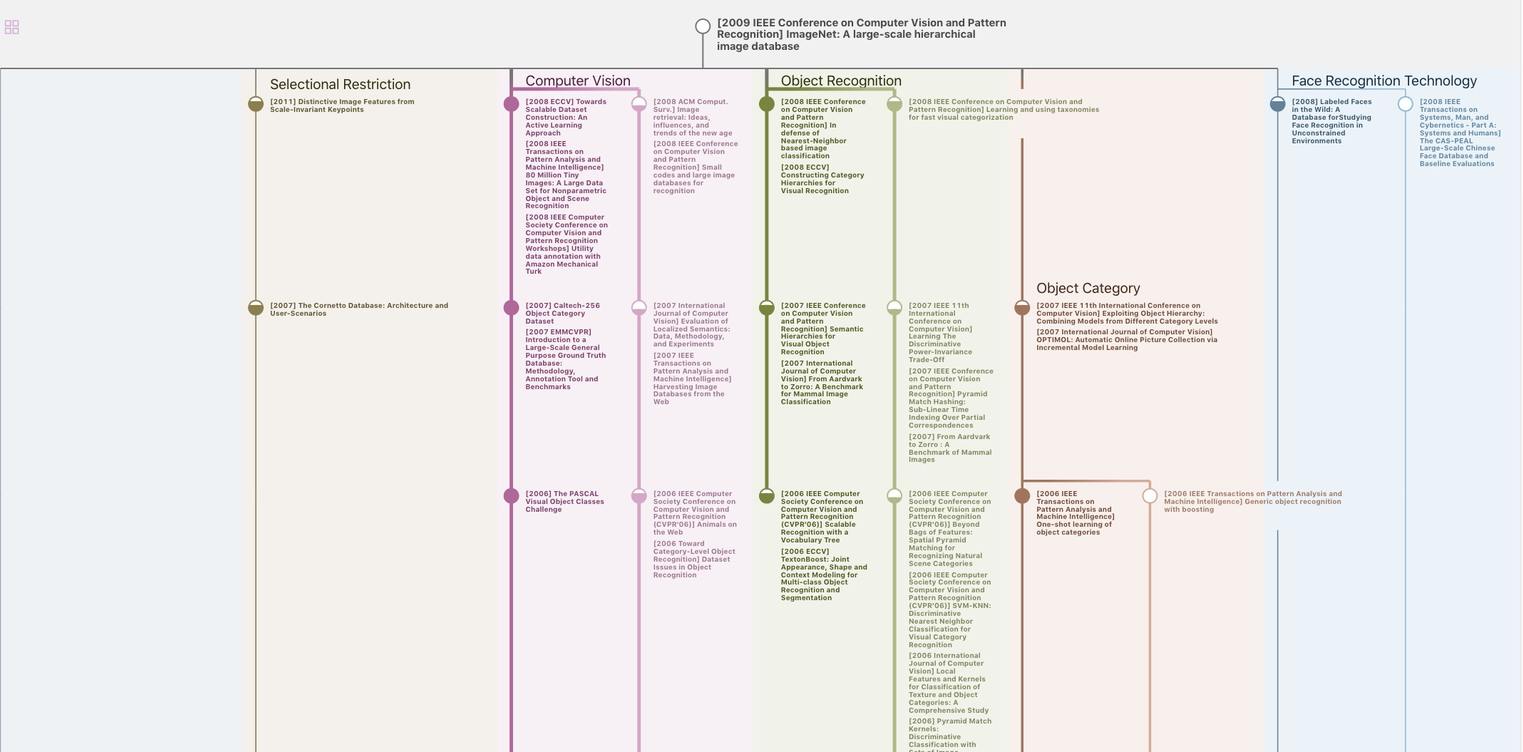
生成溯源树,研究论文发展脉络
Chat Paper
正在生成论文摘要