Learning an Enhanced TCN-LSTM Network for Temperature Process Modeling in Rotary Kilns
IEEE Transactions on Automation Science and Engineering(2024)
Abstract
Accurate prediction of reaction temperature in rotary kiln is essential to realize its advanced process control and operational optimizations. However, the complexity of the physical and chemical reactions in the rotary kiln makes it difficult for the traditional mechanism model to characterize the dynamic kiln process. In this study, a deep learning-based temperature prediction model is proposed to accurately track the temperature changes during the production process of rotary kiln. The proposed model integrates a temporal convolutional network (TCN) with a long short-term memory (LSTM) network. The former enables the proposed model to be aware of local context and can effectively compute local characteristics, whereas the latter with its time-memory capability can better capture the long-term dependencies of data to extract deep-level features. To enhance the prediction accuracy, the proposed model is further improved by introducing a novel feature fusion and hybrid pooling layer to merge the original input with TCN output features, which efficiently preserve the detailed information and significantly reduce model complexity. Attention mechanism is also incorporated after the LSTM network to concentrate on the key moment information and improve the model performance. Monitoring data from a zinc rotary kiln at a field site is used for model training and testing. Results demonstrate that the proposed model can achieve the best mean square error, 0.165, exhibiting a promising prediction accuracy of the rotary kiln’s temperature. It outperforms the state-of-the-art machine learning-based prediction models such as LSTM, Gated Recurrent Unit (GRU) and TCN.
Note to Practitioners
—This work considers high-accuracy prediction of the temperature changes during the production process of rotary kiln. It is critical to well predict kiln tail temperature by capturing long-term dependencies and extracting global and local features from time series data. Yet, this is an extremely challenging task because rotary kiln operation is a complex thermal process with multivariate, pure hysteresis, strong interference, nonlinearity and strong coupling characteristics. As a result, these issues inhibit the establishment of a model that can accurately characterize the production process of the rotary kiln and impedes the realization of automation in volatile kiln production. Current prediction methods usually adopt mechanism-based and data-driven, there are suffer from long computation time, unknown kinetic parameters and poor accuracy. Thus, they fail to effectively learn features and accurately establish model. This work proposes a temperature prediction dynamic model named enhanced TCN-LSTM, which combines a temporal convolutional network (TCN) with a long short-term memory (LSTM) network, incorporating a unique feature fusion technique along with a hybrid pooling layer and attention layer. This integration enables the model to extract both global and local features, thereby preserving detailed information efficiently while significantly reducing model complexity and enhance the stability and robustness. Experimental results based on the zinc rotary kiln dataset demonstrate that the proposed model exhibits a promising prediction accuracy of the rotary kiln’s temperature. In the future, it can be readily implemented and applied in many industrial areas such as systems modeling, time series forecasting, and predictive maintenance.
MoreTranslated text
Key words
Attention mechanism,dynamic temperature prediction,feature fusion,temporal convolutional network (TCN),long short-term memory (LSTM)
AI Read Science
Must-Reading Tree
Example
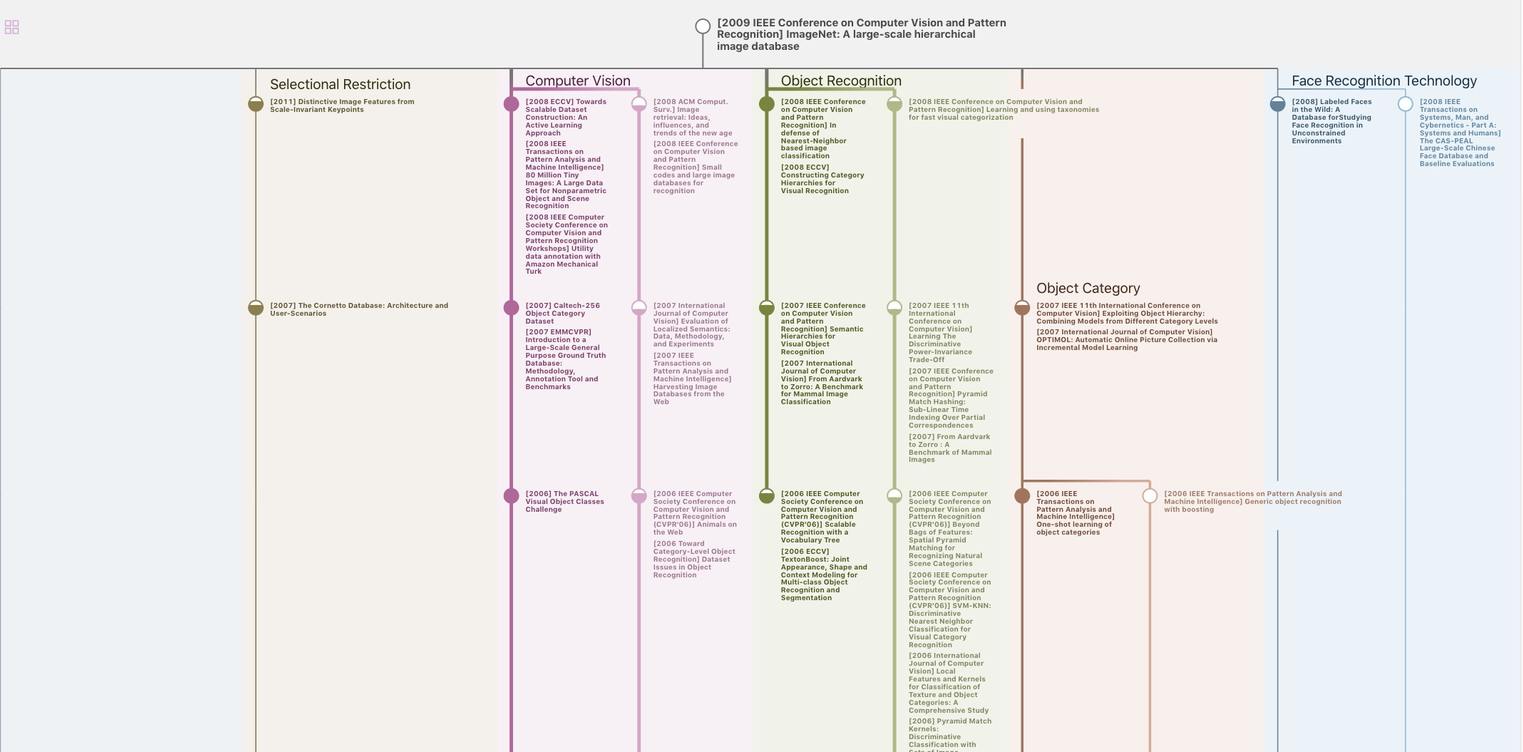
Generate MRT to find the research sequence of this paper
Chat Paper
Summary is being generated by the instructions you defined