Application of Sample Enhancement Method Combining Superpixel Segmentation and Active Learning in MBES Seafloor Sediment Classification.
IEEE Trans. Geosci. Remote. Sens.(2024)
摘要
Seafloor sediment surveys play a crucial role in providing invaluable information and guidance for ocean monitoring and management. In recent years, the combination of multibeam echosounder (MBES) data and field samples has become one of the most widespread approaches for seafloor sediment supervised classification. However, owing to the limitations of low efficiency, high complexity and high cost of seabed in situ sampling, ground truth sample data tend to have a small size, which impedes the training and deployment of classifiers. In light of the aforementioned issues, this paper proposed a seabed sediment sample enhancement method on the basis of superpixel segmentation and active learning. First, we introduce superpixel multi-resolution segmentation to effectively expand the training sample set, which takes into account the different weights of the input features. Subsequently, poor samples in the new sample set are removed using an active learning technique to improve the quality of labels. In the end, 33 original sample points are augmented to a total of 6097 valid sample points. To validate the effectiveness of our strategy, the new sample set is employed for the supervised classification of MBES data in the Southern North Sea, UK. The experimental results show that the classification accuracy of our sample enhancement method reaches 86.67%, which is a significant improvement over that of the original sample set and traditional sample enhancement methods.
更多查看译文
关键词
sample enhancement,seafloor sediment classification,multibeam echosounder,superpixel segmentation,active learning
AI 理解论文
溯源树
样例
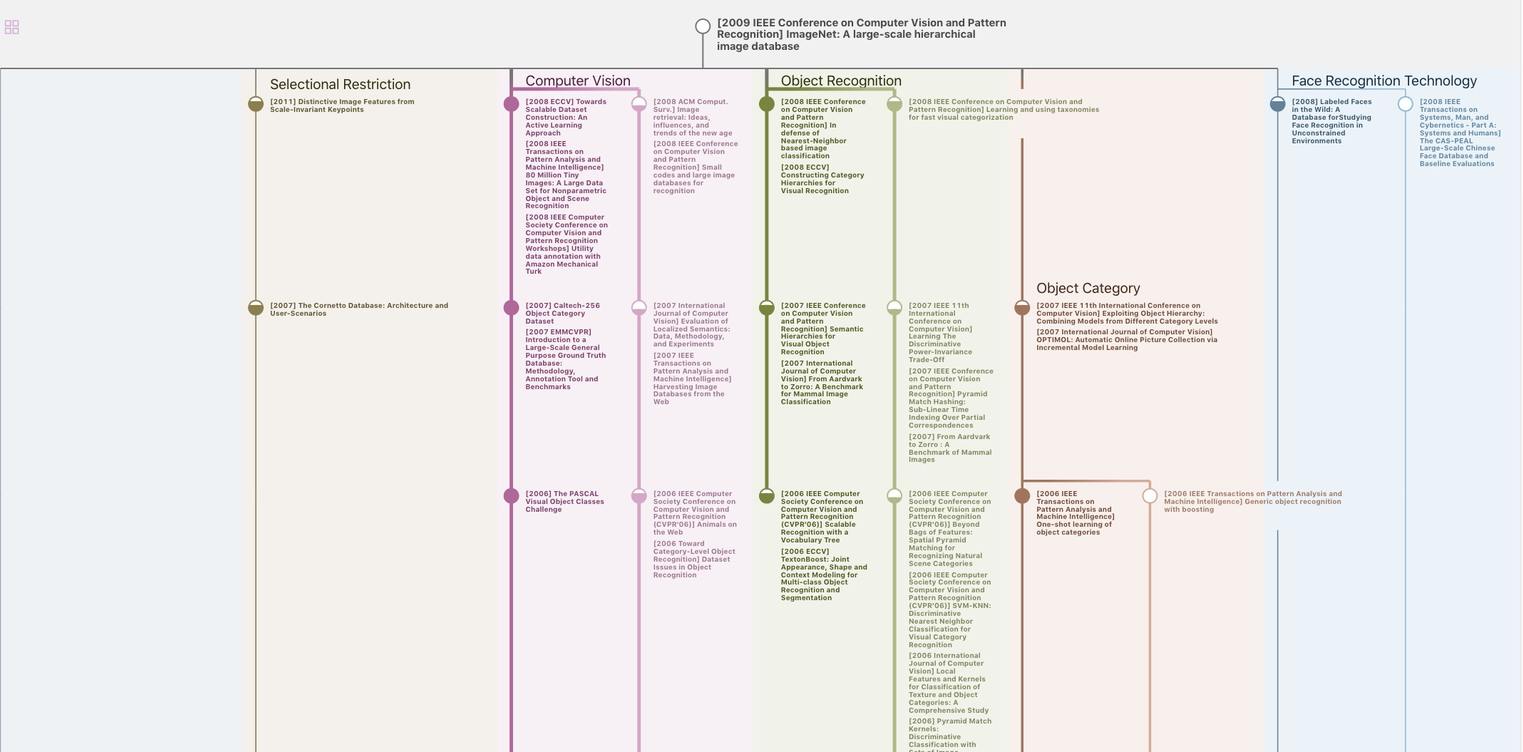
生成溯源树,研究论文发展脉络
Chat Paper
正在生成论文摘要