Enhances the recognition accuracy of the complex text for online production labels by improving convolutional recurrent neural network
Journal of Intelligent & Fuzzy Systems(2024)
Abstract
In the process of production, the label on the product provides the basic product information. Due to the complex text contained on the product labels, the high accuracy recognition for online production labels has always been a challenging problem. To address this issue, a more effective method for complex text detection by improving the convolutional recurrent neural network has been proposed to enhance the recognition accuracy of complex text. Firstly, the SE-DenseNet feature extraction network has been introduced for feature extraction, aiming to improve the model’s depth and feature extraction capacity. Then, the Bi-GRU network is utilized to learn and model the hidden states and spatial features extracted by SE-DenseNet, anticipate preliminary sequence results, reduce model parameters, and improve the model’s calculation performance. Finally, the CTC network is employed for transcription to convert each feature sequence prediction output by Bi-GRU into a label sequence, achieving complex text recognition. Experimental results on the SVT, IIIT-5K, ICDAR2013 public dataset, and a self-built dataset demonstrate that the proposed model achieves superior outcomes on both public and self-built datasets. Remarkably, the model exhibits the highest recognition accuracy of 93.2% on the ICDAR2013 public dataset, demonstrating its potential to support complex text recognition for online production labels.
MoreTranslated text
AI Read Science
Must-Reading Tree
Example
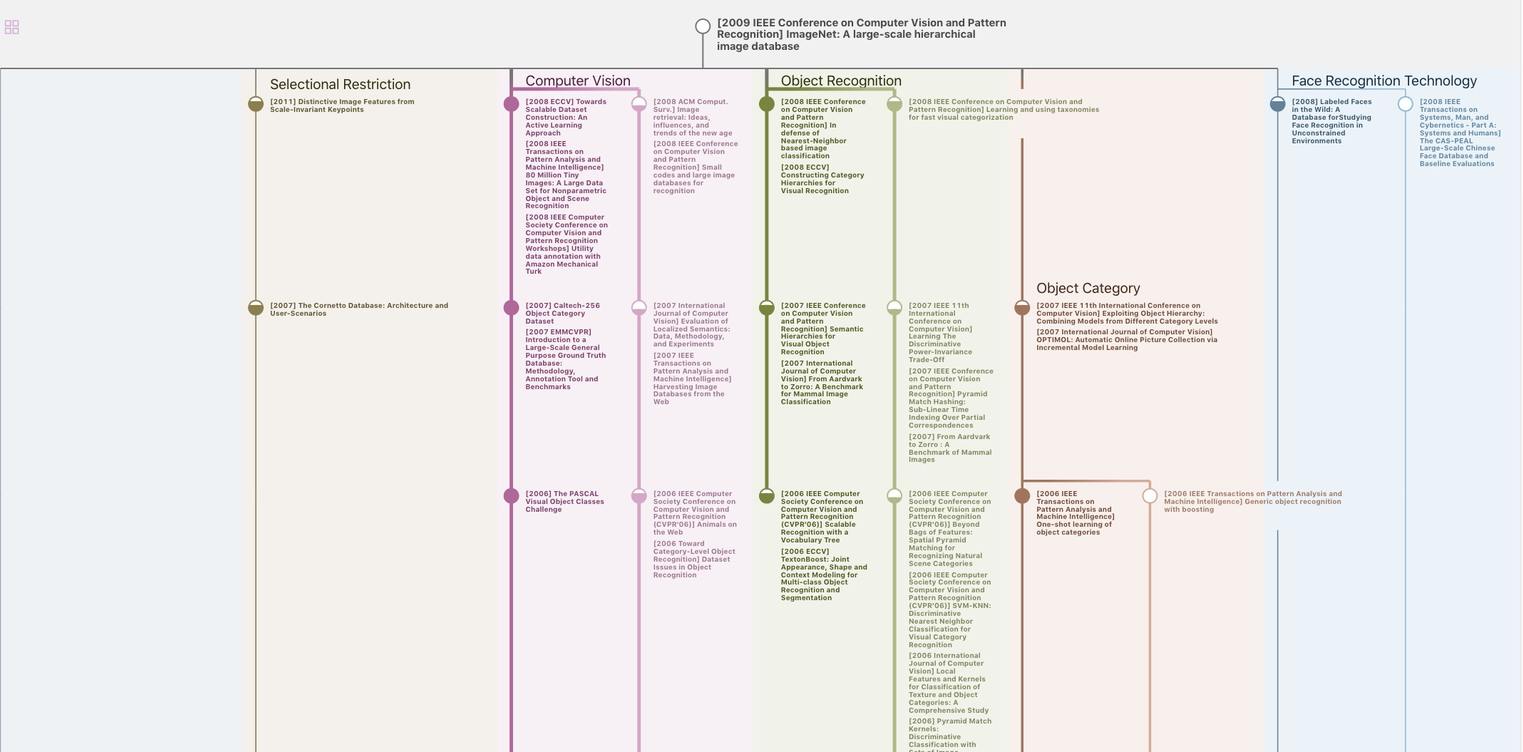
Generate MRT to find the research sequence of this paper
Chat Paper
Summary is being generated by the instructions you defined