DSANet-KD: Dual Semantic Approximation Network via Knowledge Distillation for Rail Surface Defect Detection
IEEE Transactions on Intelligent Transportation Systems(2024)
摘要
Owing to the development of convolutional neural networks (CNNs), the detection of defects on rail surfaces has significantly improved. Although existing methods achieve good results, they incur huge computational and parameter costs associated with CNNs. The usual approach to this problem is to design lightweight models that meet the needs of real-world applications; however, the performance is often compromised. To address the aforementioned problems, we designed a dual semantic approximation network via knowledge distillation (DSANet-KD, a student model with knowledge distillation) for rail surface defect detection; it focuses on both foreground and background knowledge and obtains more accurate prediction results. This model comprises an adaptive 3D spatial integration module, feature-optimization decoding module, and dual semantic approximation knowledge-distillation framework. Specifically, we employed a thoroughly trained teacher defect detection network equipped with dual semantic approximation information as an experienced teacher to guide the training of a student defect detection network. Experimental results showed that the proposed DSANet-KD achieved better accuracy with a smaller number of parameters than the state-of-the-art methods. To demonstrate the generalizability of DSANet-KD, experiments were conducted on a publicly available RGBD-SOD dataset, whose source code is available at: https://github.com/hjklearn/DSANet-KD.
更多查看译文
关键词
Adaptive 3D spatial integration module,feature-optimization decoding module,knowledge distillation,rail surface defect detection,dual semantic approximation
AI 理解论文
溯源树
样例
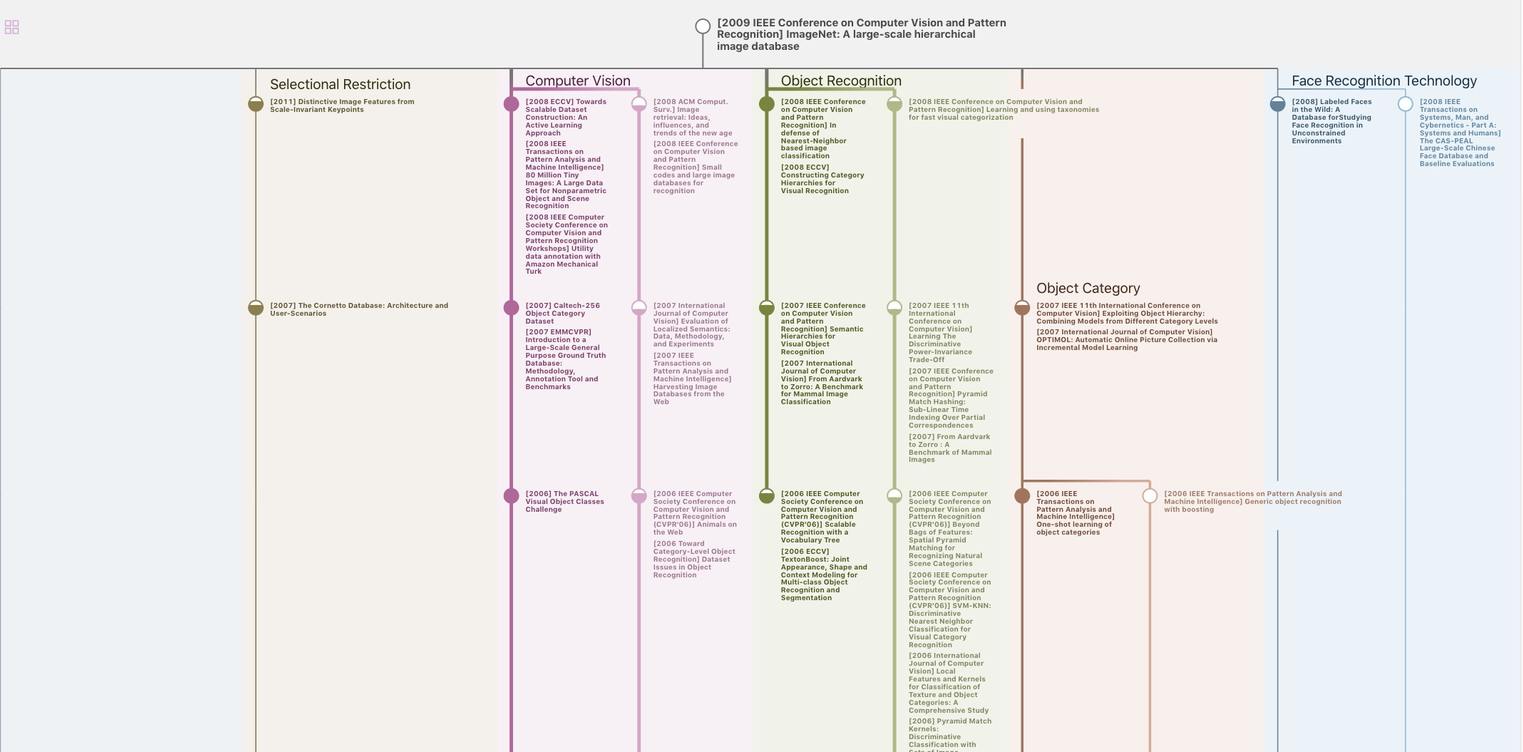
生成溯源树,研究论文发展脉络
Chat Paper
正在生成论文摘要