Adaptive prototype selection algorithm for fuzzy monotonic K-nearest neighbor
Journal of Intelligent & Fuzzy Systems(2024)
摘要
Monotonic classification is a widely applied classification task where improvements in specific input values do not lead to worse outputs. Monotonic classifiers based on K-nearest neighbors (KNN) have become crucial tools for addressing such tasks. However, these models share drawbacks with traditional KNN classifiers, including high computational complexity and sensitivity to noise. Fuzzy Monotonic K-Nearest Neighbors (FMKNN) is currently the state-of-the-art KNN-based monotonic classifier, mitigating the impact of noise to some extent. Nevertheless, there is still room for improvement in reducing computational complexity and softening monotonicity in FMKNN. In this paper, we propose a prototype selection algorithm based on FMKNN, named Condensed Fuzzy Monotonic K-Nearest Neighbors (C-FMKNN). This algorithm achieves a dynamic balance between monotonicity and test accuracy by constructing a joint evaluation function that combines fuzzy ranking conditional entropy and correct prediction. Data reduction and simplifying computations can be achieved by using C-FMKNN to filter out instance subsets under the adaptive dynamic balance between monotonicity and test accuracy. Extensive experiments show that the proposed C-FMKNN improves significantly in terms of ACCU, MAE and NMI compared with the involved KNN-based non-monotonic algorithms and non-KNN monotonic algorithms. Compared with the instance selection algorithms MCNN, MENN, and MONIPS, C-FMKNN improves the average values of ACCU, MAE, and NMI by 3.7%, 3.6% and 18.3%, respectively, on the relevant datasets. In particular, compared with the benchmark algorithm FMKNN, C-FMKNN achieves an average data reduction rate of 58.74% while maintaining or improving classification accuracy.
更多查看译文
AI 理解论文
溯源树
样例
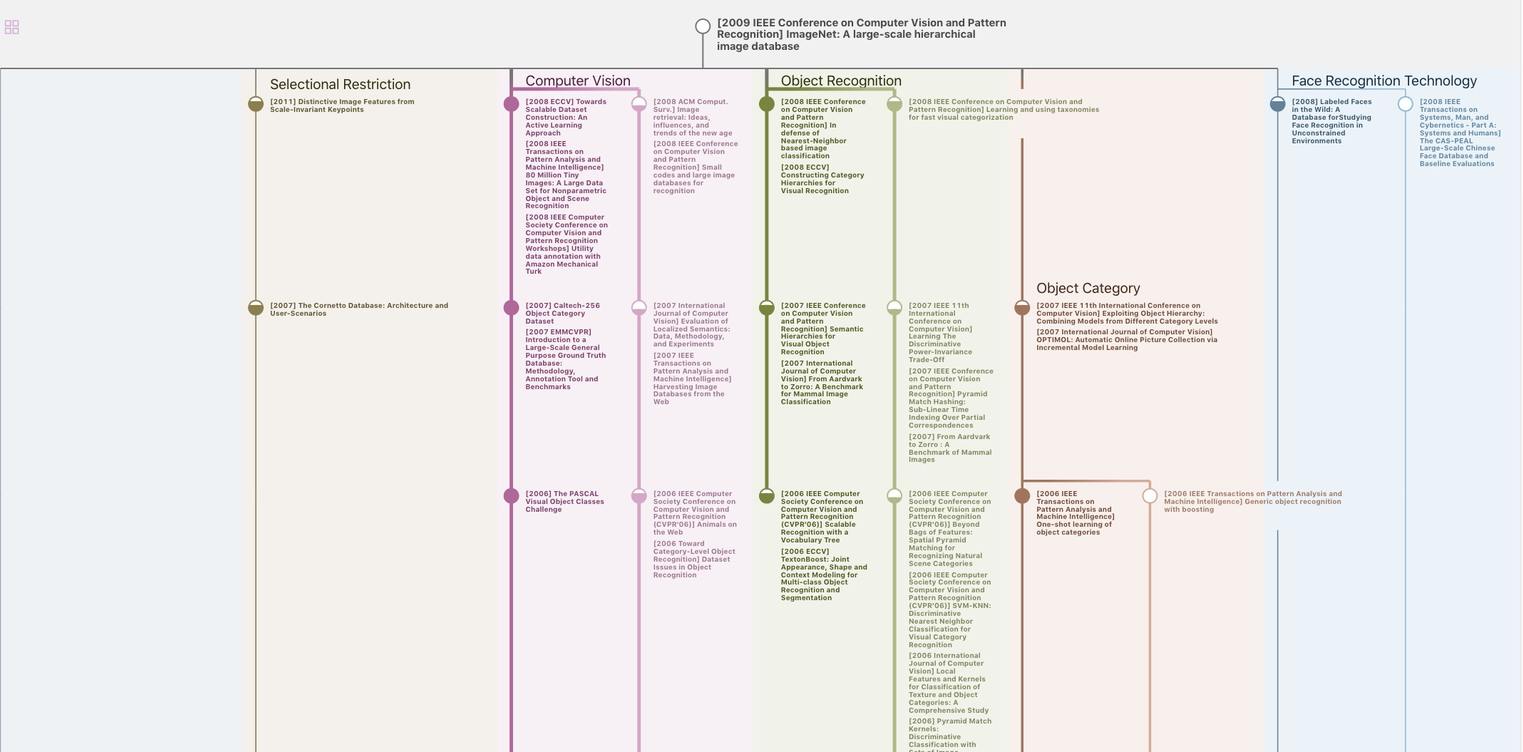
生成溯源树,研究论文发展脉络
Chat Paper
正在生成论文摘要