Privacy-Enhanced Personalized Federated Learning With Layer-Wise Gradient Shielding on Heterogeneous IoT Data
IEEE Internet of Things Journal(2024)
Abstract
Federated learning (FL) enables multiple IoT devices to collaboratively train a global model without centralizing raw data. However, achieving optimal performance for each device is challenging due to the heterogeneity of IoT data. Moreover, IoT devices, constrained in computational power and energy, necessitate innovative privacy protection solutions in FL. In this paper, we propose privacy-enhanced FL methods to address data heterogeneity in IoT. Our approach includes a dual-model framework for each device, combining a shared model to facilitate inter-client communication with a personalized model. This personalized model is developed from local aggregation, utilizing layer-wise aggregation weights generated by local hypernetworks. This integration effectively balances collective intelligence with client-specific knowledge, addressing data heterogeneity for improved personalization. Additionally, we propose a Local Gradient Shielding (LGS) method to protect training data. This method conceals gradients in locally trained personalized models, preventing data reconstruction and ensuring client privacy with minimal resource consumption. Extensive evaluations on benchmark datasets, showcasing various types and degrees of data heterogeneity, demonstrate the superiority of our approaches over current baselines.
MoreTranslated text
Key words
Privacy,federated learning,hypernetworks,local gradient shielding,data heterogeneity,IoT
AI Read Science
Must-Reading Tree
Example
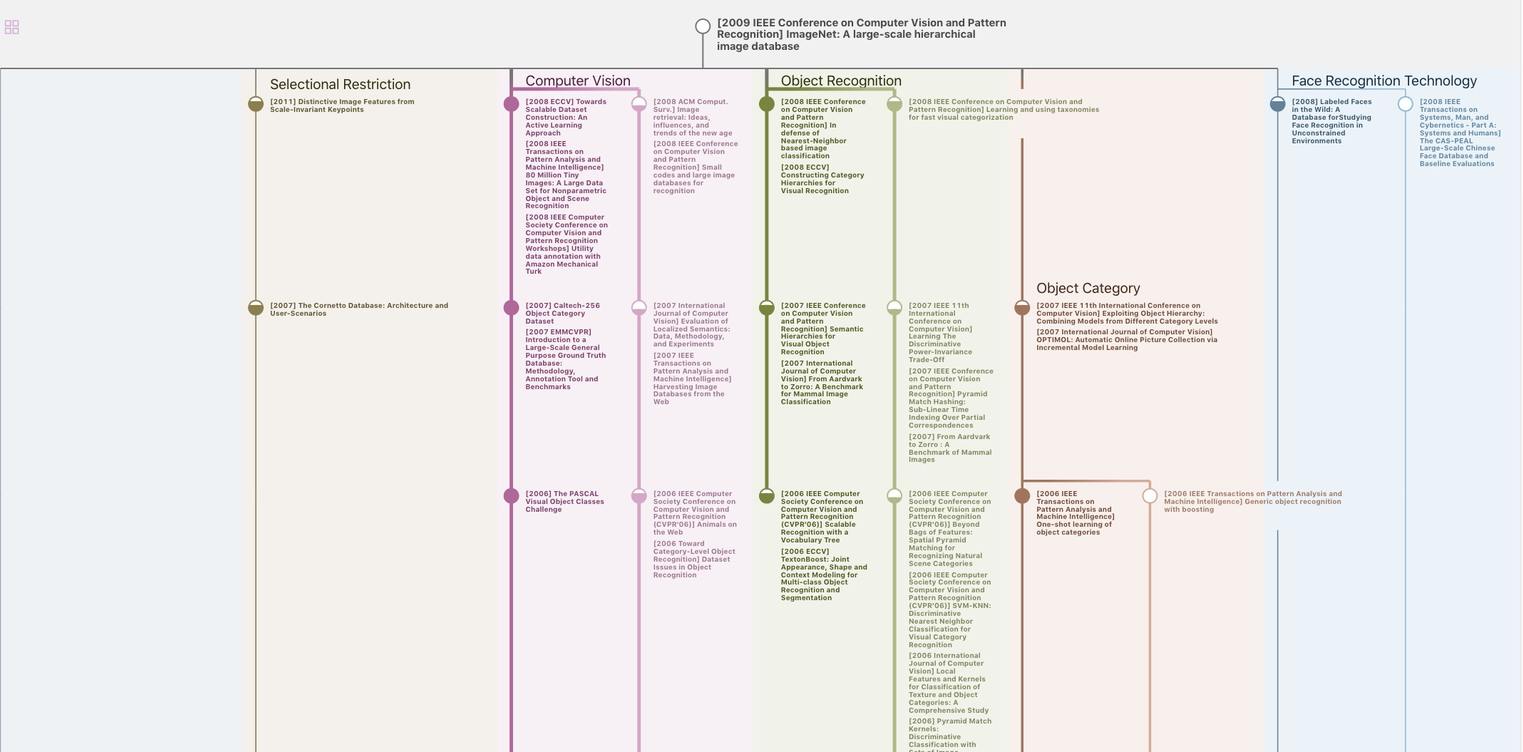
Generate MRT to find the research sequence of this paper
Chat Paper
Summary is being generated by the instructions you defined