Energy management for scalable battery swapping stations: A deep reinforcement learning and mathematical optimization cascade approach
Applied Energy(2024)
摘要
The rapid growth of electric vehicles is driving the expansion of scalable Battery Swapping Stations (BSSs) to meet the demand for fast charging. However, existing BSS energy management struggles to adapt to the changes in battery counts online, the uncertainty of electricity prices and battery demand, as well as the complexity of demand response (DR). To address these issues, we propose a cascading approach that combines Deep Reinforcement Learning (DRL) with Mathematical Optimization (MO). Firstly, our method employs a two-layer optimization framework and establishes a BSS model. The upper controller uses DRL to make sequential decisions on total power for each time slot, addressing sequential decision-making under uncertainty. The lower controller uses MO to allocate total power to each charging bay for a single time slot, addressing space complexity. Secondly, we tailor proximal policy optimization algorithms and mixed-integer quadratic programming algorithms for BSS DR. The DRL is universally adaptable to scalable BSS, while the MO automatically generates and solves optimization models based on the working charging bay count. The proposed method is at least 33 times faster than existing methods and performs well in scalable BSSs under uncertain conditions. It can converge within a typical BSS DR scheduling slot of 15 min based on an i7-8950H CPU, even for a BSS with 3200 charging bays.
更多查看译文
关键词
Scalable battery swapping station,Energy management,Demand response,Deep reinforcement learning,Mathematical optimization,Proximal policy optimization
AI 理解论文
溯源树
样例
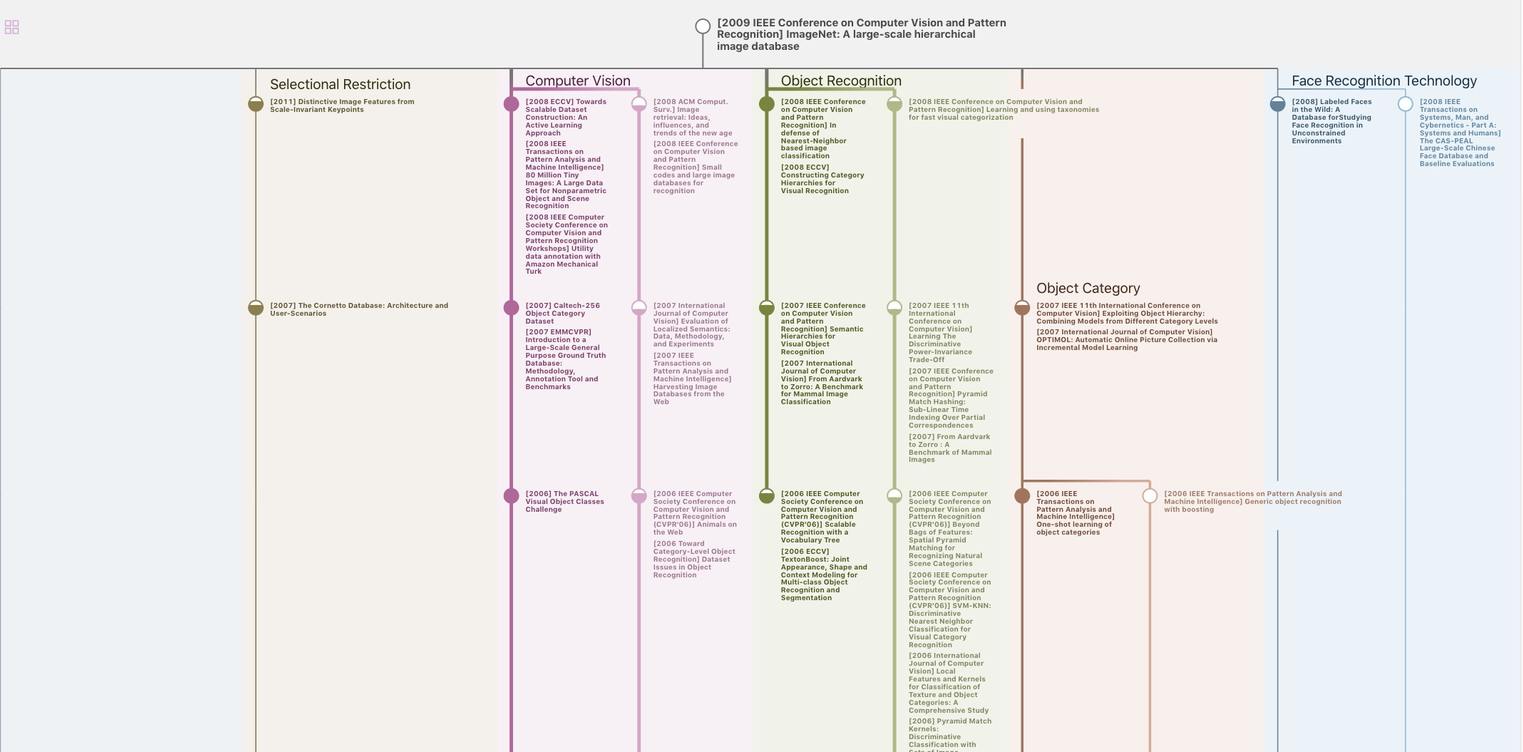
生成溯源树,研究论文发展脉络
Chat Paper
正在生成论文摘要