Activate and Reject: Towards Safe Domain Generalization under Category Shift
2023 IEEE/CVF International Conference on Computer Vision (ICCV)(2023)
摘要
Albeit the notable performance on in-domain test points, it is non-trivial for deep neural networks to attain satisfactory accuracy when deploying in the open world, where novel domains and object classes often occur. In this paper, we study a practical problem of Domain Generalization under Category Shift (DGCS), which aims to simultaneously detect unknown-class samples and classify known-class samples in the target domains. Compared to prior DG works, we face two new challenges: 1) how to learn the concept of ``unknown'' during training with only source known-class samples, and 2) how to adapt the source-trained model to unseen environments for safe model deployment. To this end, we propose a novel Activate and Reject (ART) framework to reshape the model's decision boundary to accommodate unknown classes and conduct post hoc modification to further discriminate known and unknown classes using unlabeled test data. Specifically, during training, we promote the response to the unknown by optimizing the unknown probability and then smoothing the overall output to mitigate the overconfidence issue. At test time, we introduce a step-wise online adaptation method that predicts the label by virtue of the cross-domain nearest neighbor and class prototype information without updating the network's parameters or using threshold-based mechanisms. Experiments reveal that ART consistently improves the generalization capability of deep networks on different vision tasks. For image classification, ART improves the H-score by 6.1% on average compared to the previous best method. For object detection and semantic segmentation, we establish new benchmarks and achieve competitive performance.
更多查看译文
关键词
Domain Adaptation,Transfer Learning
AI 理解论文
溯源树
样例
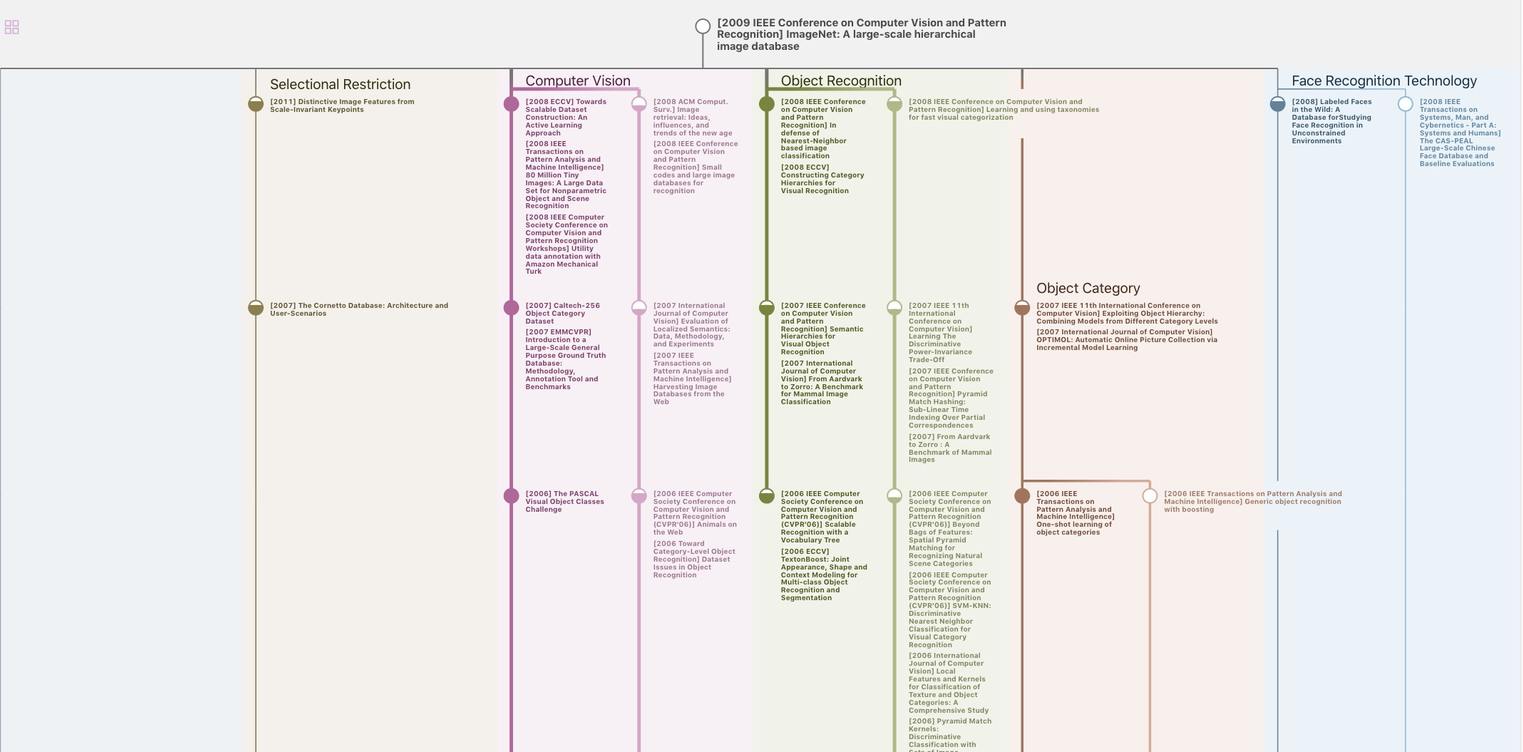
生成溯源树,研究论文发展脉络
Chat Paper
正在生成论文摘要