Spam Text Detection Over Social Media Usage: A Supervised Sampling Approach for the Social Web of Things
IEEE Systems, Man, and Cybernetics Magazine(2024)
摘要
A downsampling strategy based on negative selection density clustering (NSDC-DS) is proposed to improve classifier performance while employing random downsampling for unbalanced communication text. The discovery of self-anomalies via negative selection enhances traditional clustering. The detector and self-set are the sample center point and the sample to be clustered, respectively; anomalous matching is performed on the two; and the NSDC technique analyzes sample similarity. To improve on the traditional downsampling method, we use the Naïve Bayes Support Vector Machine (NBSVM) classifier to identify garbage in sampled communication samples, use principal component analysis (PCA) to evaluate sample information content, propose an improved PCA-signed directed graph (SGD) algorithm to optimize model parameters, and complete semisupervised communication spam text recognition over the Social Web of Things. Several datasets, including unbalanced communication text, were used to compare the improved approach against NSDC, NSDC-DS, PCA-SGD, and standard models. According to the trials, the improved model has a quicker and more consistent convergence speed.
更多查看译文
关键词
Optical Character Recognition,Spam Text,Training Dataset,Convolutional Neural Network,Support Vector Machine,Standard Model,Classification Performance,Supervised Learning,Major Classes,Convergence Rate,Clustering Algorithm,Central Point,Linear Discriminant Analysis,Unsupervised Learning,Stochastic Gradient Descent,Support Vector Machine Classifier,Class Distribution,Recognition Performance,Dense Clusters,Imbalanced Data,Spectral Clustering Algorithm,Balanced Training Set,Majority Of Classes,Adverse Selection,K-means Algorithm,High-dimensional Datasets,Training Set,Semi-supervised Learning Approach,Spectral Clustering,Balanced Set
AI 理解论文
溯源树
样例
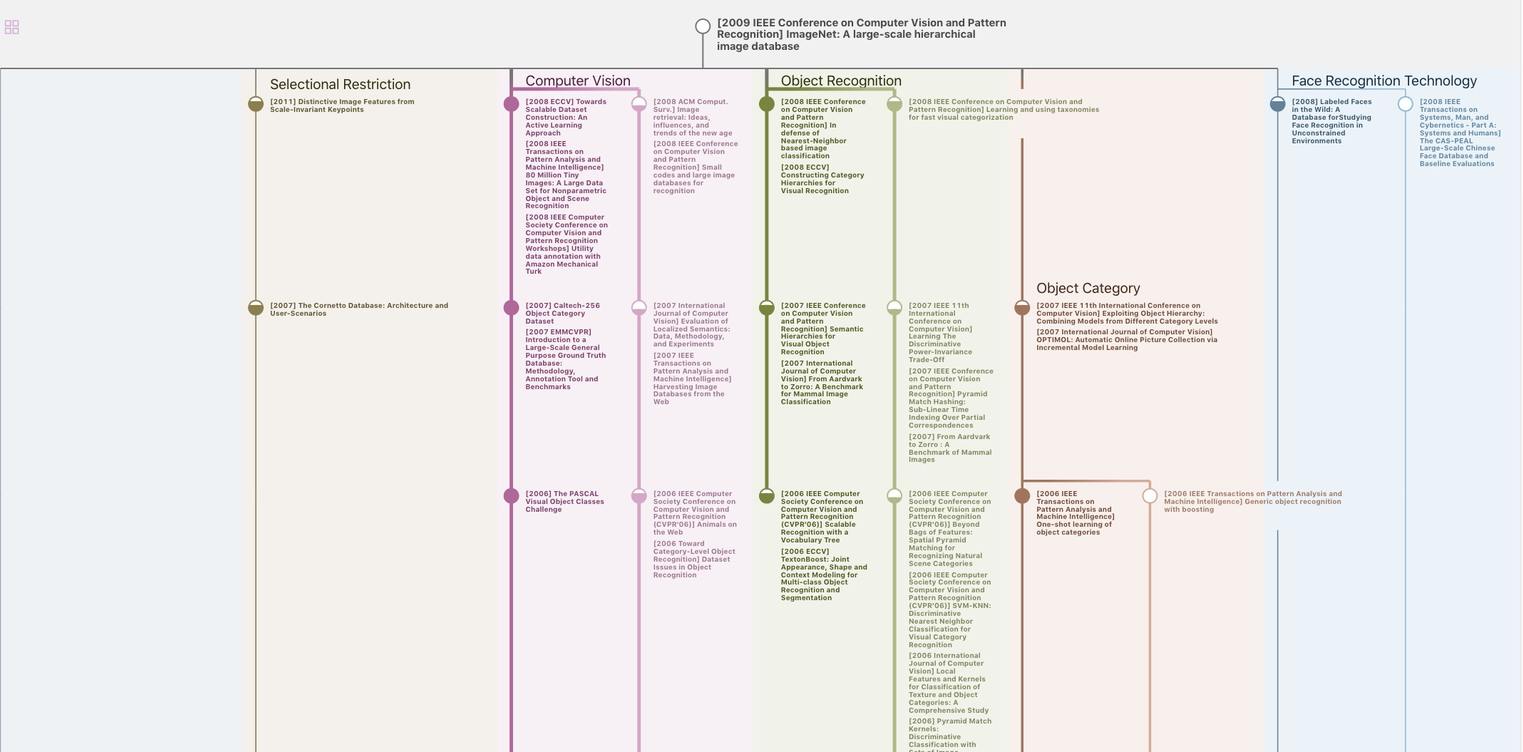
生成溯源树,研究论文发展脉络
Chat Paper
正在生成论文摘要