An Improved Bayesian Learning Method for Distribution Grid Fault Location and Meter Deployment Optimization.
IEEE Trans. Instrum. Meas.(2024)
摘要
With the development of both distribution automation and management system in distribution grid, the fault location methods based on wide-area measurement show great potential. Timely fault location based on wide-area measurements depends largely on the computing time of the algorithm. In addition, the meters layout method also affects the cost and accuracy of fault location. In this article, the efficiency of sparse Bayesian learning algorithm and the meter configuration strategy for distributed grid fault location have been improved. Different from the previous calculation for all parameters in the multi-dimensional vector of Bayesian learning, the proposed method uses the K-means algorithm to extract partial parameters in the vector for update, which improves the computational efficiency without reducing the reconstruction accuracy. Then, for the sparse meter configuration using the principle of sparse recovery, which can be optimized by improving the coherence principle of the sensing matrix. The simulation results show that the improved Bayesian learning of the proposed K-means algorithm significantly improves the computational efficiency, regardless of low-dimensional or high-dimensional signals. At the same time, use the low coherence principle of sparse recovery to guide the meter configuration in distribution grid, which could increase the overall fault location accuracy about 4 % compared to recent work. To verify the effectiveness, the performance of the method is tested on IEEE standard 69 bus distribution grids. Simulation results indicate that the suggested strategy performs well for various scenarios.
更多查看译文
关键词
Fault location,wide-area sparse measurement,Bayesian learning,meter deployment,coherence
AI 理解论文
溯源树
样例
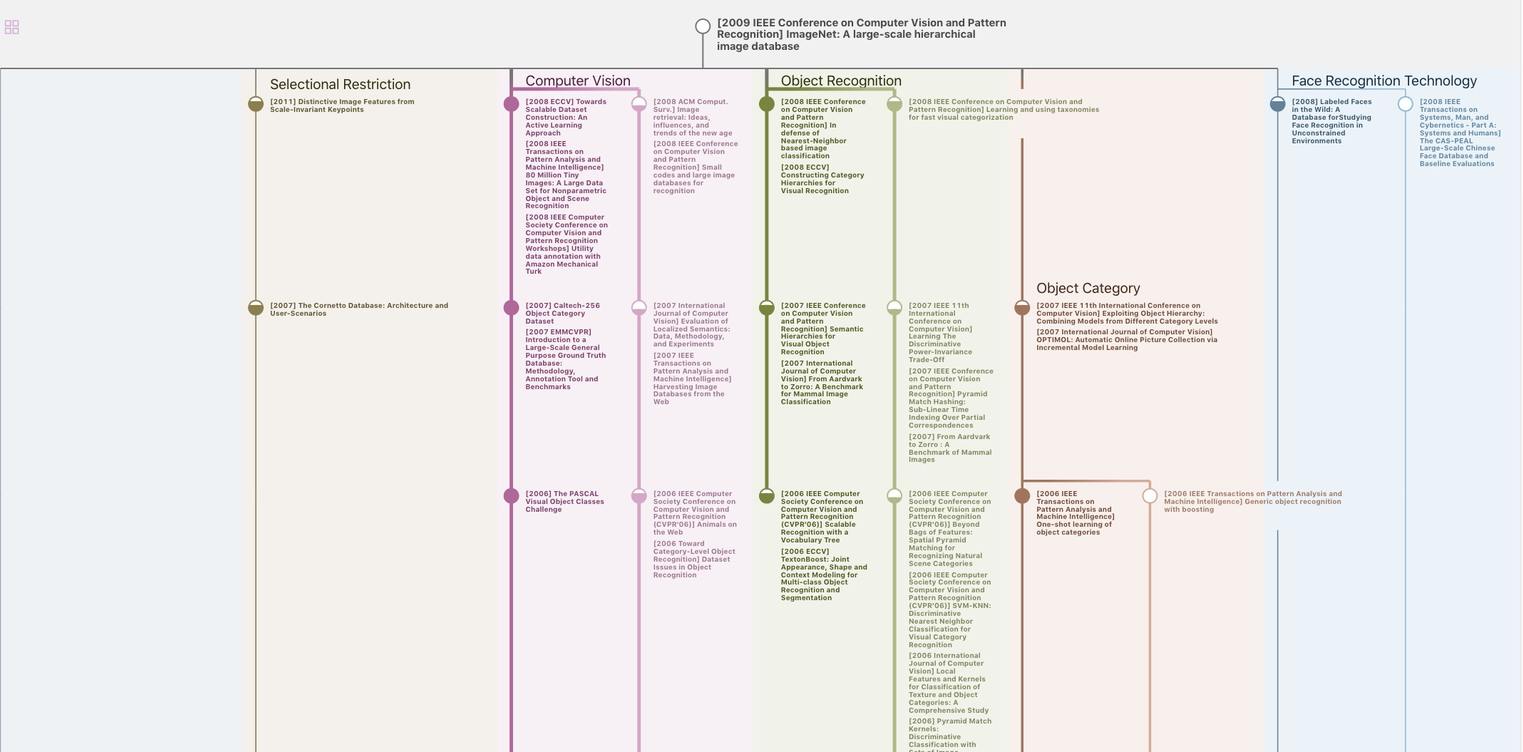
生成溯源树,研究论文发展脉络
Chat Paper
正在生成论文摘要