ER-C3D: Enhancing R-C3-D Network With Adaptive Shrinkage and Symmetrical Multiscale for Behavior Detection
IEEE TRANSACTIONS ON COMPUTATIONAL SOCIAL SYSTEMS(2024)
摘要
Behavior detection receives considerable attention in real-life human-computer interaction, where the complexity of background information and the variable durations of movements are two major factors affecting the accuracy of behavior detection. To overcome the inadequacy of these factors, this article proposes an enhancing region convolutional 3-D (ER-C3D) network with adaptive shrinkage and symmetrical multiscale for behavior detection. The improved ER-C3D network includes a feature subnet, a proposal subnet, and a classification subnet. First, a 3D-RSST unit is constructed by embedding an adaptive shrinkage structure and a soft thresholding operation. Meanwhile, a residual adaptive shrinkage mechanism, composed of multiple cascaded 3D-RSST units with different parameters, is designed to reduce redundant information of video streams in the feature subnet. Second, a spatiotemporal symmetrical multiscale structure is substituted for the single-layer convolution and embedded into the proposal subnet. Specially, contextual symmetrical multiscale motion characteristics with different levels and granularities are acquired by expanding the spatiotemporal receptive field of candidate temporal proposals. Finally, a soft-nonmaximal suppression strategy is introduced to filter high-quality temporal proposals in the classification subnet. The experimental results on the THUMOS'14 and ActivityNet1.2 datasets indicate that the mAP@0.5 of the improved ER-C3D network reaches 39.4% and 42.2%, respectively, which is 10.5% and 15.4% higher than R-C3D. Compared with related methods, the proposed method shows improvement in both the positional precision of behavioral boundary and the accuracy of behavioral classification.
更多查看译文
关键词
Behavior detection,residual adaptive shrinkage mechanism,soft-nonmaximal suppression (soft-NMS),spatiotemporal receptive field,spatiotemporal symmetrical multiscale structure
AI 理解论文
溯源树
样例
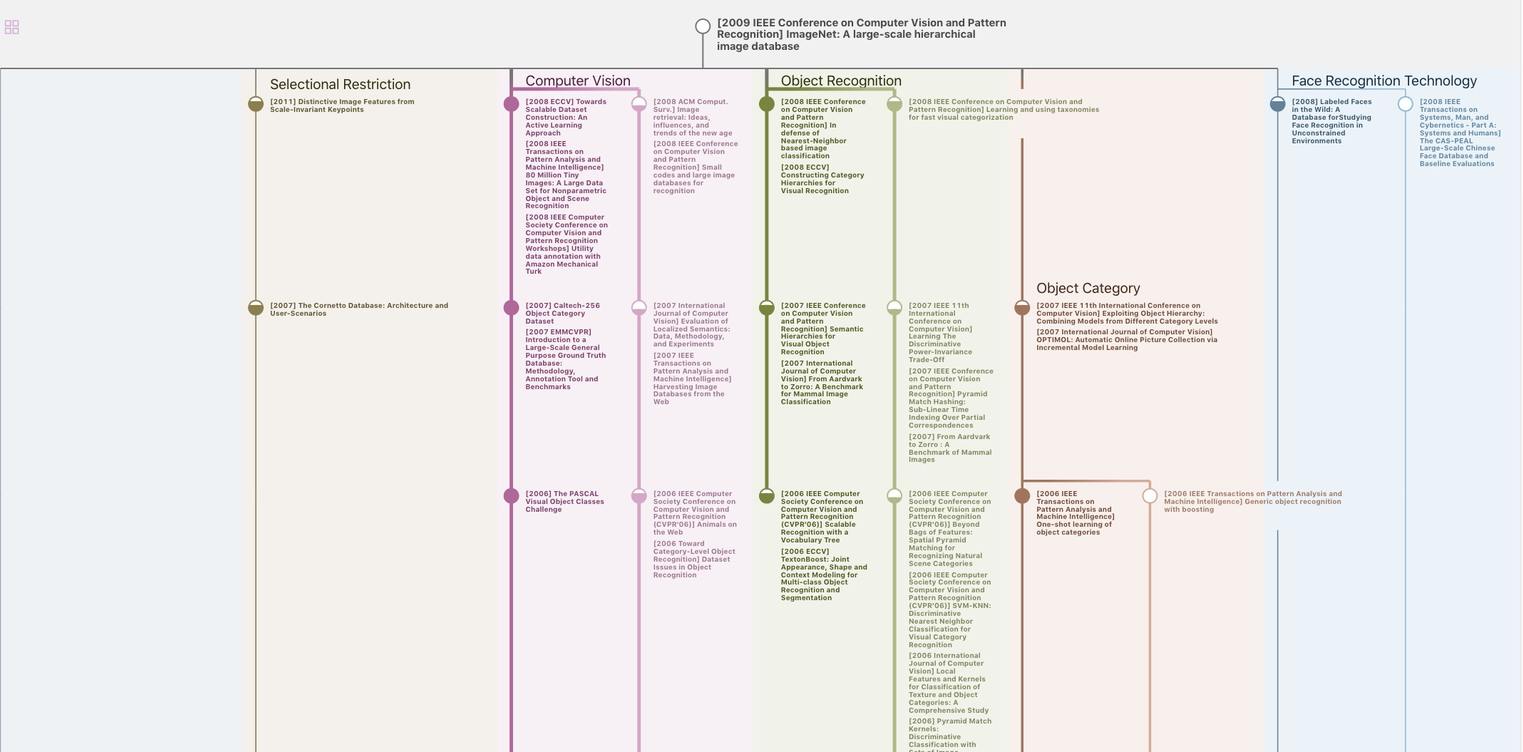
生成溯源树,研究论文发展脉络
Chat Paper
正在生成论文摘要