Neural-network-based drag force model for polydisperse assemblies of irregular-shaped particles
Powder Technology(2024)
摘要
In this study, a drag force model of irregular-shaped particles in gas-solid flows is investigated using neural network approaches. Pre-developed variational autoencoder, multi-layer perceptron model, and transpose convolutional neural-network model are utilized to obtain intermediate parameters of a pair-wise interaction point-particle (PIEP) model. These parameters are utilized to predict individual drag force coefficients of polydisperse, particulate flows. To apply the PIEP-based model to medium-concentration flows, the average drag force coefficients are obtained through an in-house particle-resolved direct numerical simulation, and a regressed model is developed to predict solid volume fraction factors. With the regression model, the PIEP-base model using the neural-network approaches shows good prediction in particulate systems from low to intermediate concentrations. This study provides computationally efficient models of practical, realistic particulate systems for large scale fluid dynamics simulation, in that it allows predicting the drag forces of polydisperse particles with a small amount of data.
更多查看译文
关键词
Neural networks,Irregular-shaped particles,Polydisperse systems,Pairwise interaction point particle model,Particle-resolved direct numerical simulation
AI 理解论文
溯源树
样例
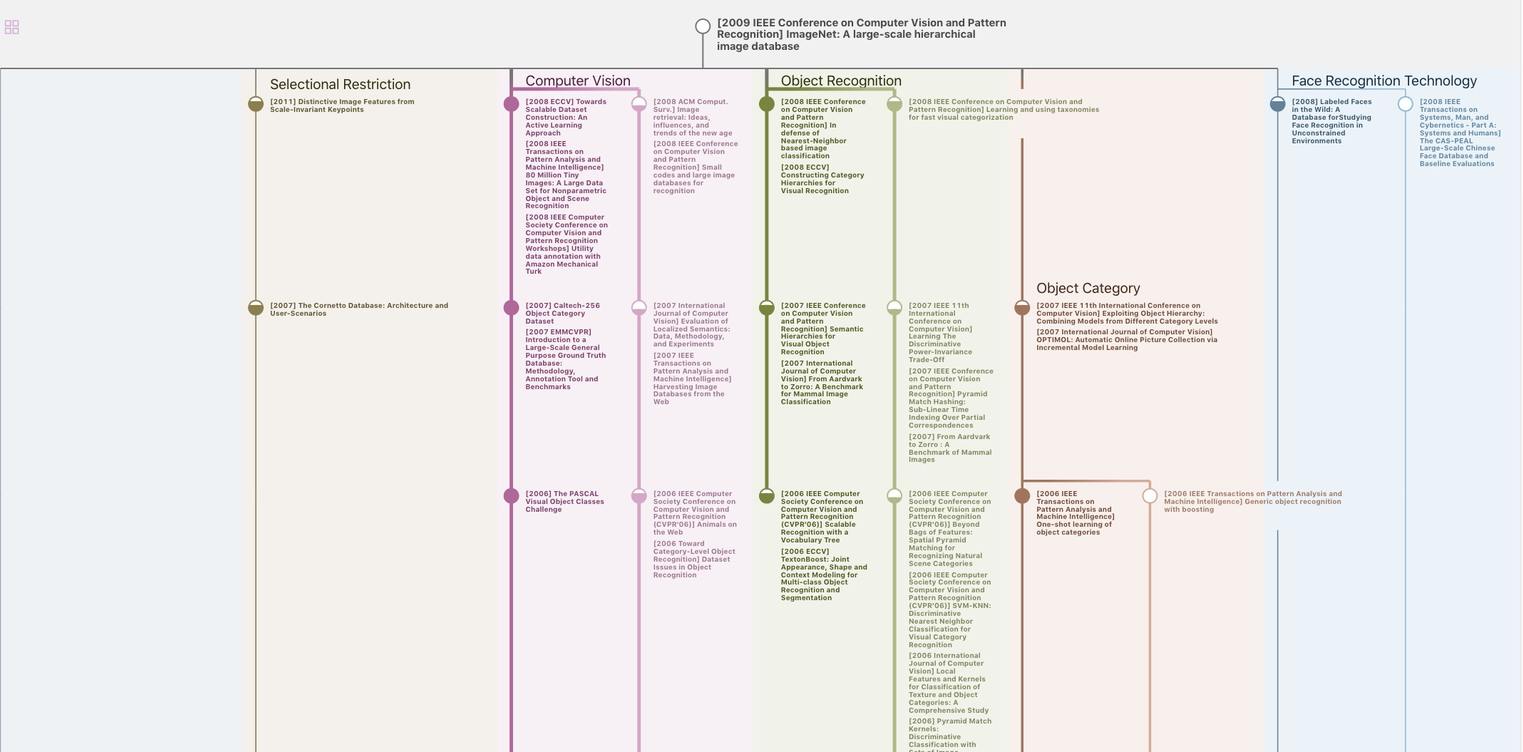
生成溯源树,研究论文发展脉络
Chat Paper
正在生成论文摘要