Decoding class dynamics in learning with noisy labels
Pattern Recognition Letters(2024)
摘要
The creation of large-scale datasets annotated by humans inevitably introduces noisy labels, leading to reduced generalization in deep-learning models. Sample selection-based learning with noisy labels is a recent approach that exhibits promising upbeat performance improvements. The selection of clean samples amongst the noisy samples is an important criterion in the learning process of these models. In this work, we delve deeper into the clean-noise split decision and highlight the aspect that effective demarcation of samples would lead to better performance. We identify the Global Noise Conundrum in the existing models, where the distribution of samples is treated globally. We propose a per-class-based local distribution of samples and demonstrate the effectiveness of this approach in having a better clean-noise split. We validate our proposal on several benchmarks - both real and synthetic, and show substantial improvements over different state-of-the-art algorithms. We further propose a new metric, classiness to extend our analysis and highlight the effectiveness of the proposed method. Source code and instructions to reproduce this paper are available at https://github.com/aldakata/CCLM/
更多查看译文
关键词
Learning with Noisy Labels,Label Noise Modelling,Class Dynamics
AI 理解论文
溯源树
样例
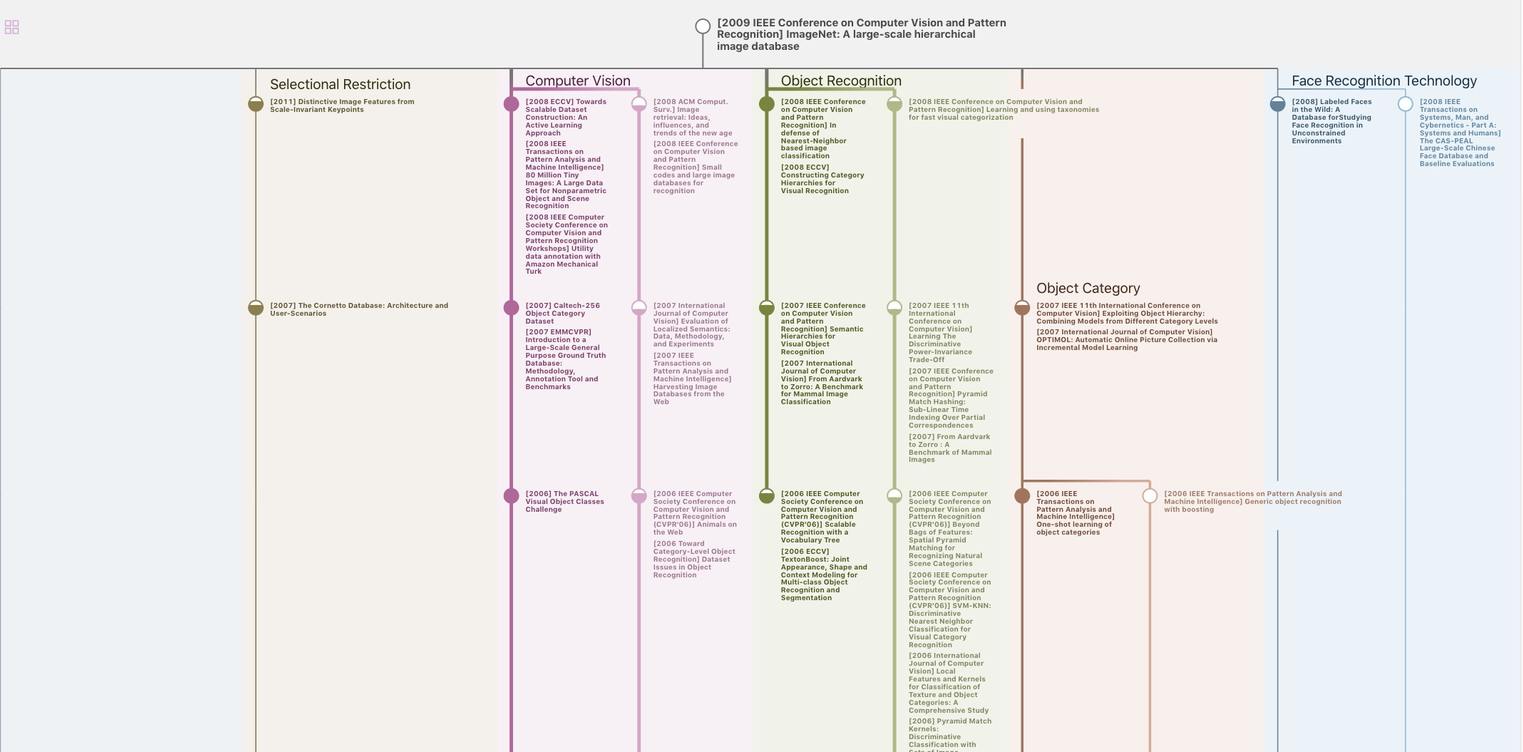
生成溯源树,研究论文发展脉络
Chat Paper
正在生成论文摘要