Neural Generative Models and the Parallel Architecture of Language: A Critical Review and Outlook
TOPICS IN COGNITIVE SCIENCE(2024)
摘要
According to the parallel architecture, syntactic and semantic information processing are two separate streams that interact selectively during language comprehension. While considerable effort is put into psycho- and neurolinguistics to understand the interchange of processing mechanisms in human comprehension, the nature of this interaction in recent neural Large Language Models remains elusive. In this article, we revisit influential linguistic and behavioral experiments and evaluate the ability of a large language model, GPT-3, to perform these tasks. The model can solve semantic tasks autonomously from syntactic realization in a manner that resembles human behavior. However, the outcomes present a complex and variegated picture, leaving open the question of how Language Models could learn structured conceptual representations. LLMs like GPT-3 show human-like behavior in solving some semantic tasks autonomously from syntax, exhibiting alignment with the parallel architecture. Yet LLMs struggle with tasks like interpreting elliptical gaps or scrambled sentences, indicating a need to further explore their ability to understand structured conceptual representations.
更多查看译文
关键词
Neural large language models,Statistical learning,Parallel architecture,Syntax-semantics interface,GPT-3 prompting,Enriched composition,Semantic composition
AI 理解论文
溯源树
样例
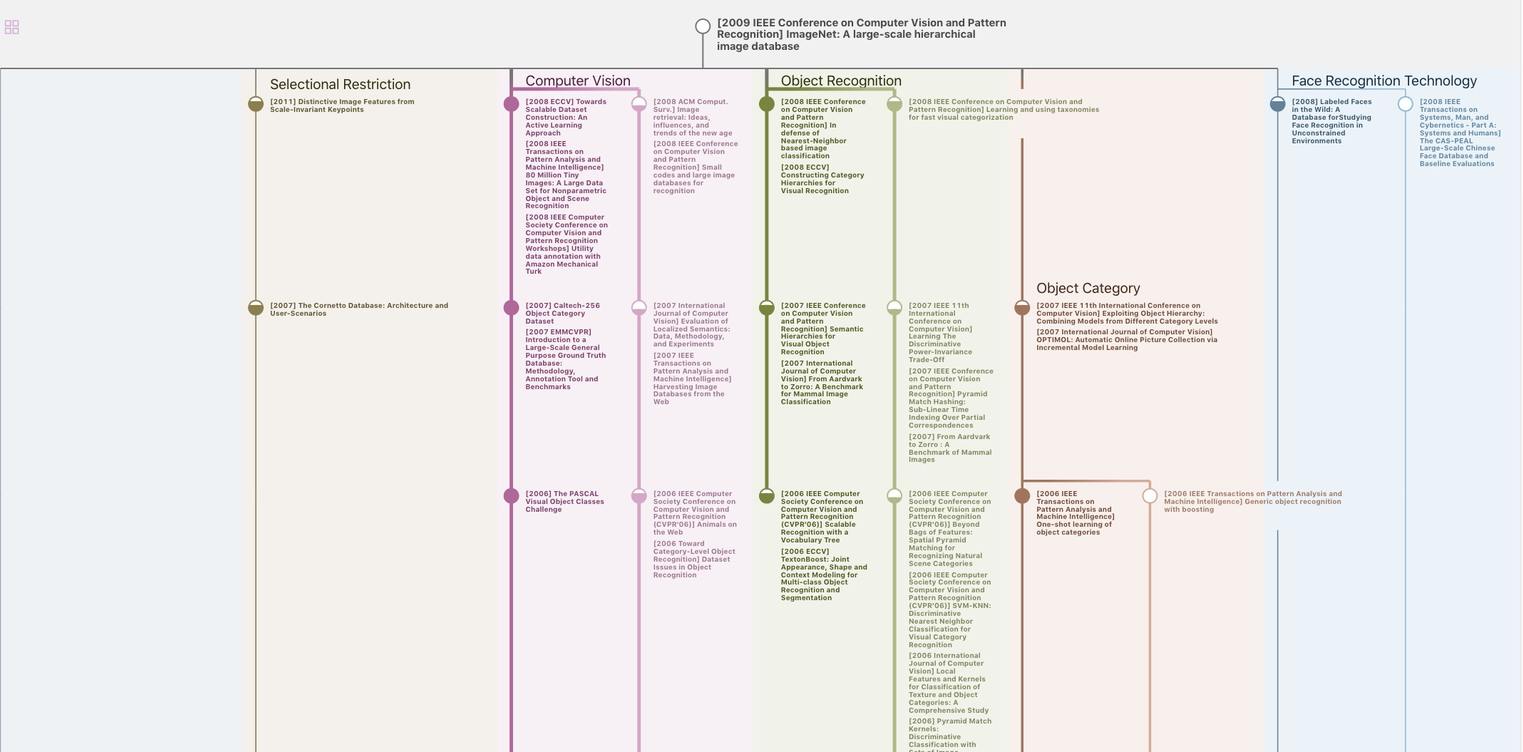
生成溯源树,研究论文发展脉络
Chat Paper
正在生成论文摘要