Exploring Dynamic Features of EEG Signals with Slow Feature Analysis for Epileptic Seizure Detection
2023 Global Conference on Information Technologies and Communications (GCITC)(2023)
摘要
Epilepsy is one of the most common disorders of the nervous system. Epileptic seizures are transient brain dysfunctions caused by sudden abnormal discharges of neurons in the brain. With computer-aided diagnosis systems, neurologists may diagnose seizure stages in Electroencephalogram (EEG) recordings more accurately. For automatic seizure detection, feature extraction is an essential stage, which is performed by handcrafted features or learning them, often by a deep neural network. In this paper, to explore the dynamic features in EEG signals, Slow Feature Analysis (SFA), a multivariate statistical approach that has been developed for process monitoring, is introduced into EEG data analysis. Several latent variables that are slowly varying and mutually independent, known as slow features, are extracted from multi-channel EEG data. Then the T
2
statistic and the S
2
statistic of a few slowest features are constructed. The series of those two statistics represent steady variations and temporal variations of the dominant slow features, respectively. Characteristics of the two series are fed into a classifier for seizure detection. To verify the role of the suggested dynamic features, the random forest algorithm is adopted as the classifier. The proposed approach is evaluated on a benchmark dataset, the CHB-MIT dataset. The results show that compared to the commonly used features in EEG analysis, the slow features represent the intrinsic characteristics of the trend of EEG signals more effectively. It is noteworthy that compared with several previous methods, ours exhibits superior performance, achieving an average accuracy, sensitivity, and specificity of 98.25%, 98.35% and 98.23%, respectively.
更多查看译文
关键词
epileptic seizure detection,multi-channel EEG,slow feature
AI 理解论文
溯源树
样例
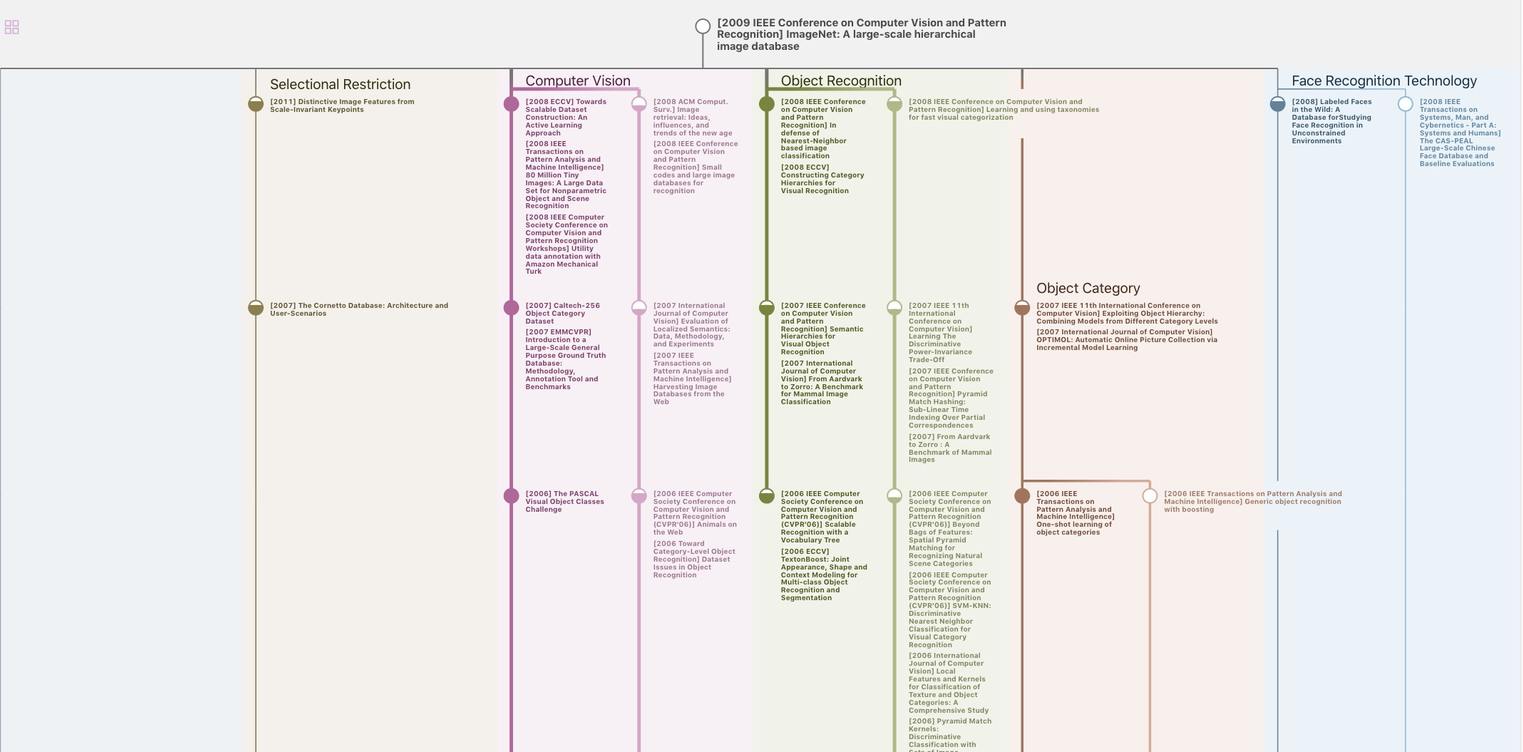
生成溯源树,研究论文发展脉络
Chat Paper
正在生成论文摘要