A Method of Robot Picking Citrus Based on 3D Detection.
IEEE Instrum. Meas. Mag.(2024)
Abstract
It is important to monitor the maturity of citrus fruits during the growth period by using deep learning to implement visual detection technology to identify their state of ripeness in their natural environment. The problem is that the ripeness of citrus fruits is mainly judged manually, and there is a lack of fast and accurate automatic detection methods for citrus fruit ripeness. In this study, the optimal weight model was trained from 1688 images of citrus fruits collected in the natural environment based on the lightweight YOLOv4-Tiny model. The experimental results show that the model has 92.4% mAP for citrus fruit detection, and the detection accuracy of Faster R-CNN is slightly higher than that of the improved YOLOv4 Tiny compared with the current mainstream two-stage detection algorithm Faster R-CNN. Although the improved YOLOv4 Tiny has better detection speed, compared with the harvesting performance of the harvesting robot, the improved YOLOv4 Tiny is better. YOLOv5 and YOLOv4 are generally weaker than the improved YOLOv4-Tiny, and the model has more parameters and occupies more memory. Meanwhile, the model can achieve accurate recognition of fruits under different shooting distances, different shading situations and different lighting situations, and can quickly and effectively identify the ripeness of citrus fruits under complex backgrounds with strong robustness. The system can provide technical support for yield estimation of citrus fruit orchards and the location detection of picking machines and has reference value for recognition and ripeness detection of other fruits.
MoreTranslated text
AI Read Science
Must-Reading Tree
Example
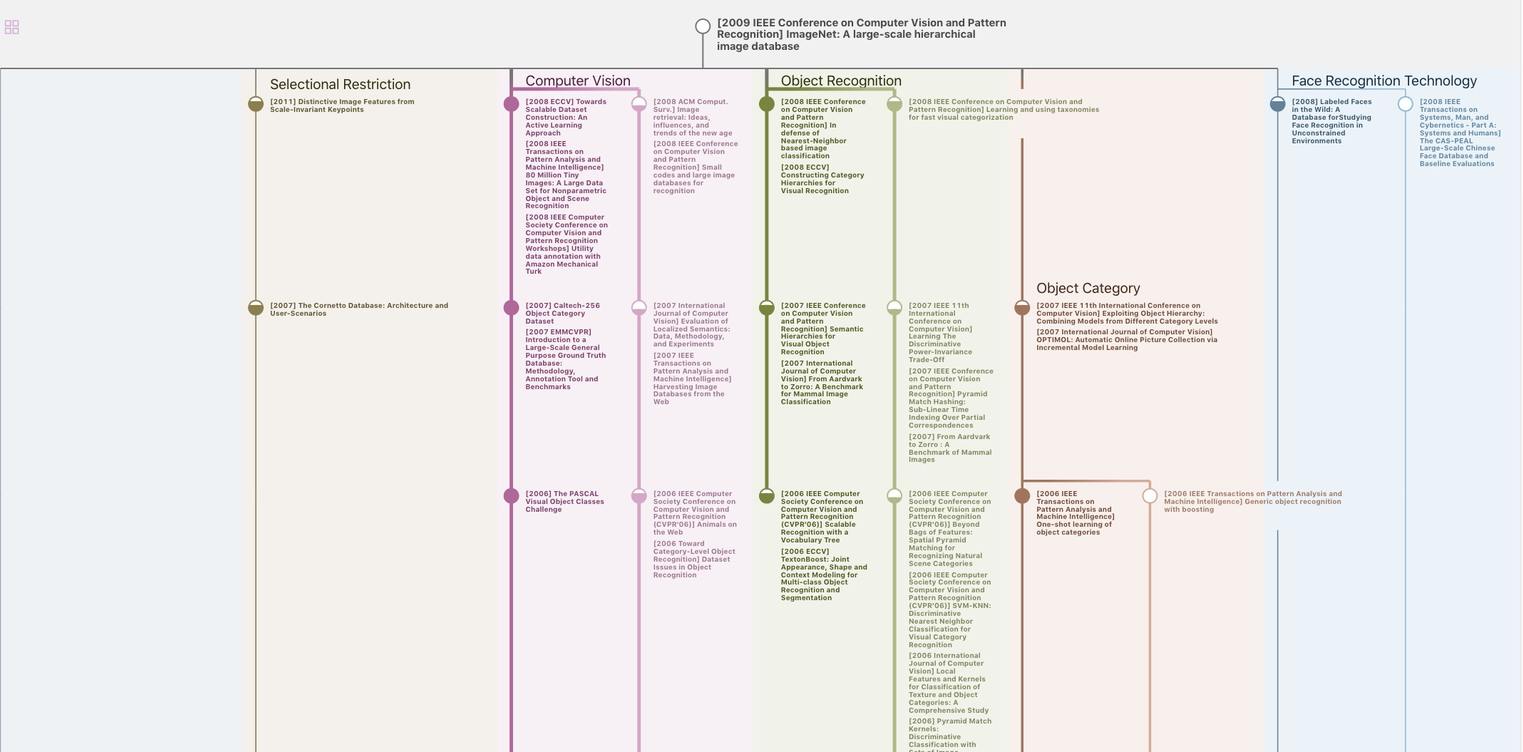
Generate MRT to find the research sequence of this paper
Chat Paper
Summary is being generated by the instructions you defined