Disentangled Relational Graph Neural Network with Contrastive Learning for Knowledge Graph Completion
Knowledge-Based Systems(2024)
摘要
Learning disentangled entity representations has garnered significant attention in the field of knowledge graph completion (KGC). However, the existing methods inherently overlook the indicative role of relations and the correlation between latent factors and relations, leading to suboptimal entity representations for KGC tasks. In the current study, we introduce the Disentangled Relational Graph Neural Network with Contrastive Learning (DRGCL) method, designed to acquire disentangled entity representations guided by relations. In particular, we first devise the factor-aware relational message aggregation approach to learn entity representations under each semantic subspace and obtain latent factor representations by attention mechanisms. Subsequently, we propose a discrimination objective for factor-subspace pairs using a contrastive learning approach, which compels the factor representations to distinctly capture the information associated with different latent factors and promote the consistency between factor representations and semantic subspaces. Through disentanglement, our model can generate relation-aware scores tailored to the provided scenario. Extensive experiments have been conducted on three benchmark datasets and the results demonstrate the superiority of our method compared with strong baseline models.
更多查看译文
关键词
Knowledge graph completion,Disentangled representation learning,Graph neural network,Contrastive learning
AI 理解论文
溯源树
样例
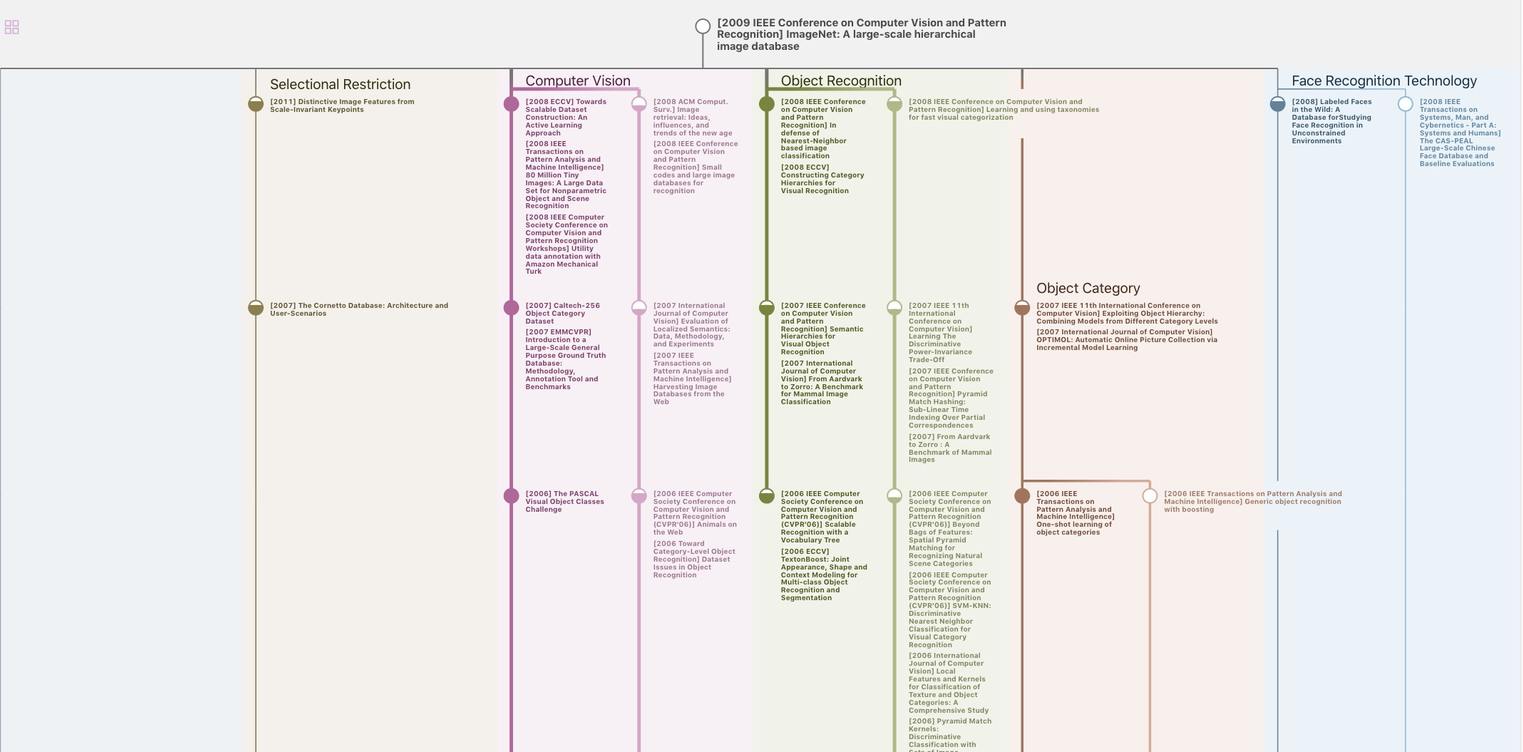
生成溯源树,研究论文发展脉络
Chat Paper
正在生成论文摘要