Source bias reduction for source-free domain adaptation
Signal, Image and Video Processing(2024)
Abstract
Source-free domain adaptation (SFDA) mainly aims to the problem of not being able to access the source domain data during the model migration process. Although significant breakthroughs have been achieved, the current works meet performance ceiling. The key problem is that the source bias of the adapted model is difficult to be eliminated. In this work, we propose a novel SFDA method named rectiFication Upon SEmantic information (FUSE). The key idea is to reduce source bias of the adapted model with the help of pre-trained vision–language model (e.g., CLIP). Two strategies are adapted. The first is named source bias reduction, which is to restrict the impact of the samples with inconsistent predictions between the source and pre-trained models. The samples with high confidence classification based on pre-trained model automatically assume the task of supervision. Another one adjusts the pre-trained model to fit the distribution of the target domain. The features that better represent class centers are extracted. Except these two strategies, we also adapt pseudo-labeling method to further improve the performance of the adapted model. Experiments on three benchmark datasets show that our method achieves the state-of-the-art results.
MoreTranslated text
Key words
Source-free domain adaptation,Vision–language models,Source bias reduction
AI Read Science
Must-Reading Tree
Example
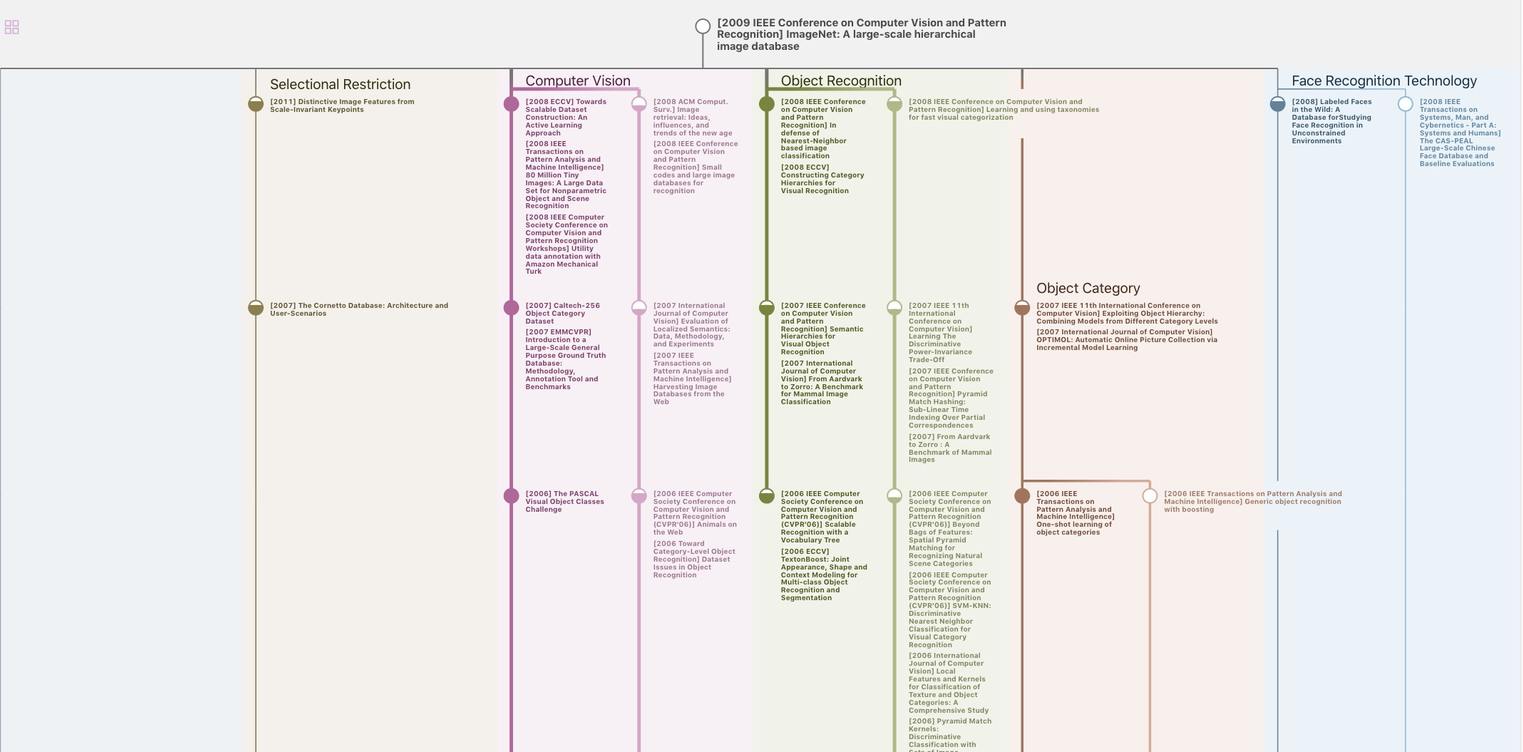
Generate MRT to find the research sequence of this paper
Chat Paper
Summary is being generated by the instructions you defined