Improving data-efficiency of deep generative model for fast design synthesis
Journal of Mechanical Science and Technology(2024)
摘要
The convolutional neural network-based deep generative model (DGM) is a powerful tool for handling image datasets that opens up strategies for fast synthesis of optimum designs at unseen boundary conditions. Existing DGMs for design synthesis are typically based on O (10000) training data, which limits the engineering applications. This paper explores the feasibility of improving DGM data efficiency with O (100) training data through prior constraints. A two-stage data-efficient deep generative model (DE-DGM) is proposed which leverages the first-stage design synthesis from probabilistic proper orthogonal decomposition and the second-stage enhancement from encoder-decoder convolutional neural network. Four topology optimization cases have been adopted, including compliance minimization, heat conduction, airplane bearing bracket design, and three-dimensional machine tool column structure design. The proposed DE-DGMs could be trained with 100–200 data and synthesize the main features of the design at unseen boundary conditions. The overall computation cost of warm-start topology optimization leveraging DE-DGM predictions reduces to 36
更多查看译文
关键词
Design optimization,Reduced-order modeling,Data-efficient deep learning,Image processing,Topology optimization
AI 理解论文
溯源树
样例
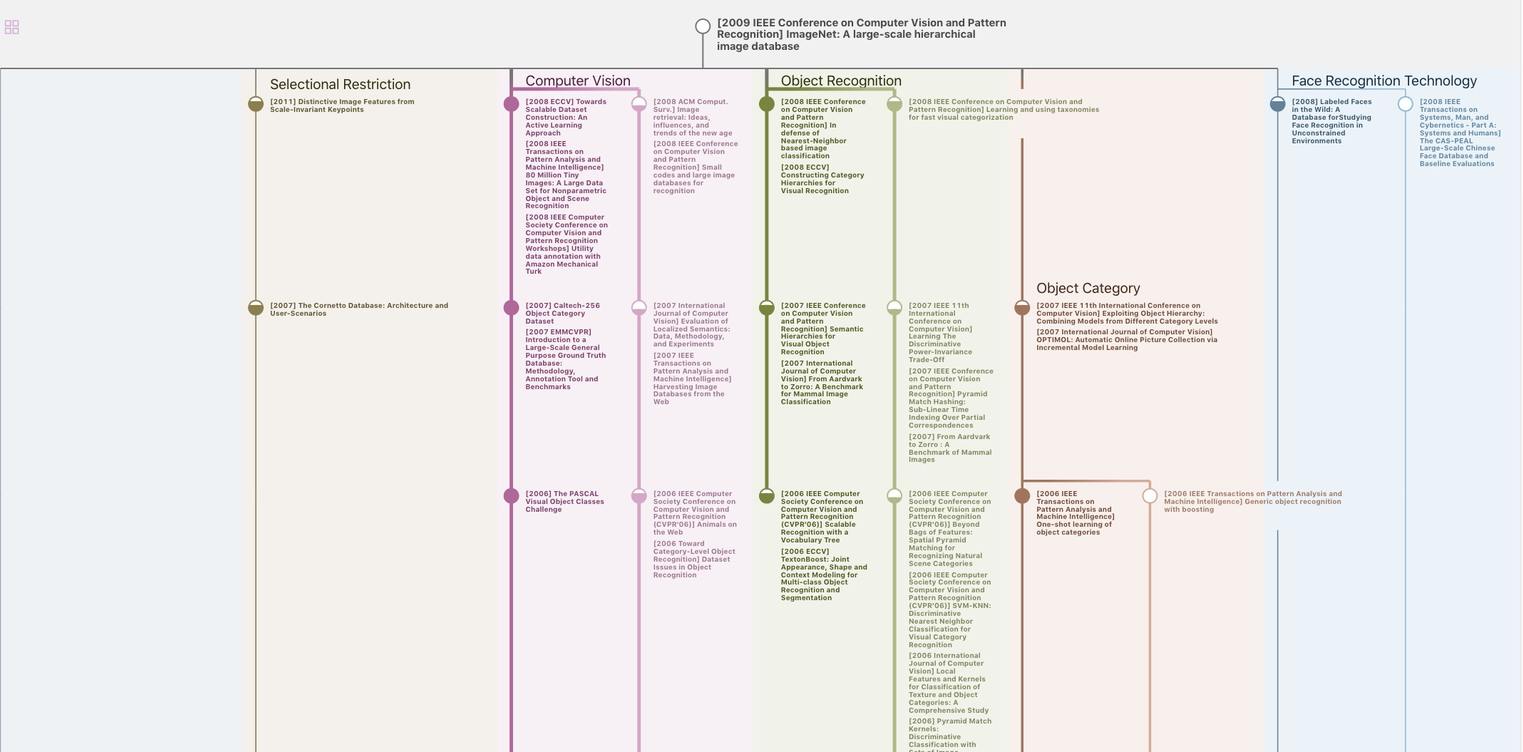
生成溯源树,研究论文发展脉络
Chat Paper
正在生成论文摘要